the Creative Commons Attribution 4.0 License.
the Creative Commons Attribution 4.0 License.
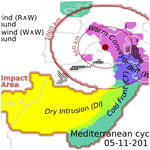
Linking compound weather extremes to Mediterranean cyclones, fronts, and airstreams
Shira Raveh-Rubin
Jennifer L. Catto
Yonatan Givon
Olivia Martius
Mediterranean cyclones are the primary driver of many types of surface weather extremes in the Mediterranean region, the association with extreme rainfall being the most established. The large-scale characteristics of a Mediterranean cyclone, the properties of the associated airflows and temperature fronts, the interaction with the Mediterranean Sea and with the topography around the basin, and the season of occurrence all contribute to determining its surface impacts. Here, we take these factors into account to interpret the statistical links between Mediterranean cyclones and compound extremes of two types, namely co-occurring rain–wind and wave–wind extremes. Compound extremes are attributed to a cyclone if they fall within a specially defined Mediterranean cyclone impact area. Our results show that the majority of Mediterranean rain–wind and wave–wind extremes occur in the neighbourhood of a Mediterranean cyclone, with local peaks exceeding 80 %. The fraction of compounds happening within a cyclone's impact area is highest when considering transition seasons and for rain–wind events compared with wave–wind events. Winter cyclones, matching with the peak occurrence of large and distinctively baroclinic cyclones, are associated with the highest compound frequency. A novel deconstruction of cyclones' impact areas based on the presence of objectively identified airstreams and fronts reveals a high incidence of both types of compound extremes below warm conveyor belt ascent regions and of wave–wind extremes below regions of dry intrusion outflow.
- Article
(11061 KB) - Full-text XML
-
Supplement
(8969 KB) - BibTeX
- EndNote
In November 2011 a Mediterranean cyclone named Rolf struck the western Mediterranean Basin, causing fatalities and damage in the Balearic Islands, southern France, Corsica, and northern Italy. The cyclone formed on 5 November through the interaction of a strong eastward-propagating front with the Pyrenees; it then remained stationary south of the French coast for approximately 4 d, where it developed tropical-like characteristics (see details in Dafis et al., 2018; Gutiérrez-Fernández et al., 2024) before making landfall in southeastern France and dissipating on 10 November. The stationarity and intensity of Rolf resulted in persistent torrential rainfall, strong winds, and high waves, leading to floods, landslides, and falling trees (The Washington Post, 2011; DWD, 2011). Major impacts, ranging from house evacuations, traffic disruptions, and blackouts to costly infrastructural damage in the public and private sectors, affected “Var département” in France and “regione Liguria” in Italy, extending to other adjacent areas (Impact Forecasting LLC, 2011). This is only one example of a high-impact compound extreme event brought by the passage of a Mediterranean cyclone.
The compounding of extreme events (referred to in the following as “compound extremes” or simply “compounds”) constitutes a higher societal and economic risk compared with single extreme events (Zscheischler et al., 2018; Seneviratne et al., 2012; Ridder et al., 2020; Zscheischler et al., 2020). In this work we focus on the co-occurrence of meteorological hazards, specifically of extreme rain1 and wind and of extreme wave height and wind, because of the potential impacts of these weather conditions in the European region (Ridder et al., 2020; De Luca et al., 2020).
Severe weather in the midlatitudes is often associated with the presence of extratropical cyclones (e.g. Jansa et al., 2001; Nissen et al., 2010). Coherent airstreams and surface impacts are arranged around the storm's low-pressure centre by the anticyclonic circulation, according to a conceptual extratropical-cyclone model (e.g. Carlson, 1980; Schultz et al., 2019, and references therein). In the eastern warm sector, warm moist air, namely the warm conveyor belt, ascends northward and around the cyclone centre, producing intense precipitation (Madonna et al., 2014). The cold front, separating the warm and cold sector of the cyclone, trails to the south of the storm and produces pre-frontal convective activity (Catto and Pfahl, 2013). In satellite imagery the combination of warm conveyor belt and cold front forms a synoptic-scale comma-shaped cloud structure wrapping around the cyclone from the southeast. In the western cold sector, anomalously dry and cold airflows descending from the north generate a predominantly cloud-free region, characterised by isolated convection forming cumulus-type clouds. Specifically, dry intrusions transport cold and dry air from the upper troposphere and, when interacting with the warmer boundary layer, may generate strong surface wind gusts and low-level instability to the south of the cyclone (Raveh-Rubin, 2017).
Mediterranean cyclones, generally shorter-lived and smaller than their Atlantic equivalents (e.g. Campins et al., 2011; Lionello et al., 2016), share the aforementioned dynamical features (Flaounas et al., 2022, 2015), although interactions with regional topography may modify the distribution of airstreams and surface impacts. A recent classification of Mediterranean cyclones based on their large-scale dynamics unveils differences in terms of lifecycle characteristics, spatio-temporal occurrence, and associated meteorological hazards (Givon et al., 2024a) and proves to be useful for understanding which types of cyclones are most prone to inducing compound extremes.
The passage of cyclones around the Mediterranean Basin is statistically well linked to regional (extreme) weather conditions in terms of precipitation (Pfahl and Wernli, 2012; Jansa et al., 2001; Pfahl, 2014) and wind (Pfahl, 2014; Nissen et al., 2010; Dowdy and Catto, 2017). The connection with rain–wind extremes has received less attention at this regional scale (for works on larger domains see Owen et al., 2021; Ridder et al., 2020; Catto and Dowdy, 2021). Recent studies on Mediterranean rain–wind extremes have considered a small sample of large-scale events (Raveh-Rubin and Wernli, 2015) or have adopted a Lagrangian cyclone-centred perspective (Rousseau-Rizzi et al., 2023). The concomitant occurrence of high waves and strong winds within the Mediterranean Sea has hardly been analysed, in spite of its considerable impacts, e.g. infrastructural damage along the coast, interruption of vessel traffic, or ship accidents (Cavaleri et al., 2012; Bertotti and Cavaleri, 2008; Zhang and Li, 2017), and its societal relevance when considering the number of people crossing the Mediterranean on poor-quality boats.
In this work, we perform a benchmark analysis of the distributions of compound extremes (rain–wind and wave–wind, Sect. 2.1) and their association with Mediterranean cyclone tracks (Sect. 2.2) against existing literature. Our work extends previous studies by using the latest datasets to examine a range of previously unexplored settings and questions. In particular, we analyse mutual dependencies between compound extremes and Mediterranean cyclones to tackle the following research questions:
-
Does the presence of a cyclone impact the frequency of compound extremes? What proportion of compounds is associated with a nearby cyclone? (Sect. 4, 4.1, 4.2)
-
Which amongst cyclone cold fronts, dry intrusions, or warm conveyor belts (collectively referred to as dynamical features) is the most important for compound extremes? (Sect. 4.1, 4.2)
-
Extending the work by Givon et al. (2024a), what types of cyclones are locally relevant for the occurrence of compound extremes? (Sect. 4.3)
While exploring the statistics of compounds and cyclones from a (geographical) Eulerian perspective, we put equivalent emphasis on winter and transition seasons (autumn, spring) because of severe cyclone-related weather conditions occurring at such times.
An additional yet fundamental methodological question concerns the attribution of weather events to Mediterranean cyclones. This has been done in Flaounas et al. (2018) for the case of intense regional rainfall, but their method is too restrictive for identifying extreme surface winds and waves, often happening at some distance from the storm centre (Nissen et al., 2010; Pfahl, 2014; Raveh-Rubin and Wernli, 2016). Alternative reference approaches are designed for extratropical cyclones in general, which spend most of their lifecycle over the open ocean (e.g. Catto and Dowdy, 2021). Here we propose an alternative definition for a Mediterranean cyclone impact area that takes into account the type of compound, the peculiarity of the geographic setting, and the synoptic airstreams and fronts developing around the system (Sect. 3).
2.1 Compound extremes
Univariate extremes of 6 h cumulative precipitation (rain), maximum 10 m wind gust (wind), and maximum significant swell and wave height (wave) are identified in the ERA5 reanalysis dataset (Hersbach et al., 2020) from January 1980 to December 2019. The data have a temporal frequency of 6 h, with accumulations or maxima computed for each 6 h time interval centred around 00:00, 06:00, 12:00, and 18:00 UTC. A horizontal resolution of 0.5° suffices for the identification of surface extremes with scales of the order of 100 km, such as those typically induced by synoptic-scale weather systems, but it is unable to capture small-scale (convectively driven) extremes. Grid-point values exceeding the local 98th year-round percentile (other percentiles were tested) and a minimum threshold of 2 mm for rain, 10 m s−1 for wind, and 2 m for waves constitute our sample of univariate extremes (see maps of thresholds in Fig. S1 in the Supplement2); these are classified as rain–wind or wave–wind compound extremes when occurring at the same time step and grid point.
We adopt year-round thresholds for the identification of extremes to obtain consistent and potentially impactful events across seasons, with minimum thresholds intended for neglecting the occurrences which, despite belonging to the upper quantile, are very weak in intensity (e.g. rainfall over the Sahara; see Fig. S1). Weather extremes from the upper two percentiles, although allowing for a limited detection of summer compounds of both types, provide an adequate sampling of compounds during the other seasons. The selection comprises extremes with varied impact potential: while the effects of extreme 6 h rain depend on its accumulation over hourly to seasonal time spans (e.g. Guzzetti et al., 2008; Froidevaux et al., 2015; Kilsdonk et al., 2022), wind events of this type are damage-relevant (Klawa and Ulbrich, 2003). Therefore, it is worth bearing in mind that, even if not all statistically extreme meteorological conditions have serious societal or environmental consequences, extremes that are per se moderate occasionally combine to produce severe impacts (Seneviratne et al., 2012).
To estimate rainfall we use ERA5 total precipitation, corresponding to the sum of the large-scale and convective precipitation generated by the ECMWF Integrated Forecasting System (IFS). Since the IFS grid (∼30 km spacing in the horizontal) does not resolve convective processes, these are parametrised; note that convection can be relevant for the (concomitant) occurrence of small-scale rain, wind, and wave extremes. Lavers et al. (2022) visually compare ERA5 patterns with four observed impactful rainfall extremes and show a satisfactory performance of the reanalysis, notwithstanding a negative bias in representing the highest precipitation totals. The temporal correspondence between observed and ERA5 intense precipitation events is estimated by Rivoire et al. (2021) to be around 40 %–50 %. Owen et al. (2021) analyse the geographical and temporal distribution of compound rain–wind extremes and report a high consistence between ERA5 and observed datasets. The simulation of wind gusts and waves in ERA5 has improved compared with ERA-Interim (Hersbach et al., 2020), although negative wind gust biases are measured inland and over orography because of the incorrect representation of wind channelling (see Minola et al., 2020; Obermann-Hellhund, 2022, and ECMWF user guide, https://confluence.ecmwf.int/display/CKB/Windstorm+footprints%3A+Product+User+Guide, last access: 29 July 2024), and negative wave biases are reported, especially around coastal areas (Fanti et al., 2023).
2.2 Mediterranean cyclones
We consider cyclones over the Mediterranean region in the period of 1980–2019. The cyclone tracks are a result of the efforts of the MedCyclones COST Action3 to produce a reference dataset of cyclone intensity and position (i.e. the value and location of the sea level pressure (SLP) minimum) within the Mediterranean region. The composite tracking approach described in Flaounas et al. (2023) combines 10 different cyclone detection and tracking methods applied to the ERA5 reanalysis (Hersbach et al., 2020) to identify cyclones spending at least 24 h in a broad Mediterranean domain (20–50° N and 20° W–45° E). Although the tracks are computed on the original ERA5 temporal and spatial resolution, in this study, for consistency with the compound data described in Sect. 2.1, we interpolate the position onto a 0.5° horizontal grid and select the time steps coinciding with 00:00, 06:00, 12:00, and 18:00 UTC.
As in recent articles using the Flaounas et al. (2023) cyclone dataset (see Givon et al., 2024a; Rousseau-Rizzi et al., 2023), we select the tracks with a confidence level of 5, i.e. those satisfying an agreement amongst a minimum of five detection methods for at least 12 h in the cyclone's lifetime. The selection yields 3043 cyclones from 1980 to 2019 and excludes many shallow heat lows occurring in summer or in the transition seasons (Givon et al., 2024a).
2.2.1 Cyclone clusters
A clustering of the aforementioned Mediterranean cyclones based on their upper-level potential vorticity (PV) characteristics is performed by Givon et al. (2024a). They obtain nine cyclone classes (identified by numbers), which differ by construction in terms of upper-level dynamics and synoptic-scale drivers but also reveal a dominant seasonality and spatial distribution, together with distinctive tropospheric characteristics and surface impacts (see Table 1). Clusters 1, 2, and 4 are winter baroclinic cyclones (stage A and B lee lows and cyclones developing from Rossby wave breaking); clusters 5 and 8, peaking in the transition seasons, correspond to anticyclonic and cyclonic wave breaking; clusters 3 and 7, showing maximum occurrence in spring, are associated with long-wave cut-off lows and daughter lows, respectively; clusters 6 and 9, often land based and displaying a shallow low-level structure, are summer lows (Sharav heat low and short-wave cut-off low, respectively). For more details refer to Givon et al. (2024a) and Rousseau-Rizzi et al. (2023).
Table 1List of cyclone clusters from Givon et al. (2024a) with synoptic setting, peak season, and peak region of occurrence.
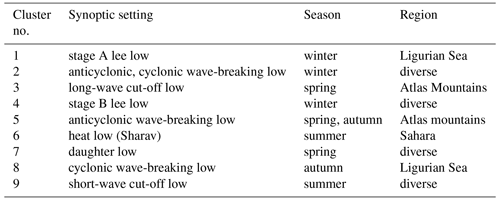
2.3 Dynamical features
Mediterranean cyclones, as extratropical cyclones in general, are circulation anomalies which displace air masses and create strong temperature gradients (Flaounas et al., 2022; Carlson, 1980). In this work we consider three types of airstreams and fronts typical of extratropical cyclones, namely warm conveyor belts (WCBs), dry intrusions (DIs), and cold fronts (CFs), and we analyse their links to compound extremes. A schematic of their organisation around Mediterranean cyclones and the related surface impacts is provided in Fig. 17 of Raveh-Rubin and Wernli (2016).
We use existing datasets of objectively identified dynamical features to label Boolean feature masks, as in the following:
-
WCB masks where the WCB inflow and ascent trajectory density is greater than zero. Lagrangian WCB trajectories are identified in the ERA5 reanalysis based on an ascent of at least 600 hPa within 48 h and on the presence of a nearby extratropical cyclone (Madonna et al., 2014; Heitmann et al., 2023; Wernli and Davies, 1997; Sprenger et al., 2017); the areas of low-level inflow (up to 800 hPa) and mid-level ascent (up to 400 hPa) are taken into account. Because the global cyclone tracks of Heitmann et al. (2023) include a different set of systems and because they use a different WCB–cyclone attribution method, in this work WCB objects are not strictly associated with a Mediterranean cyclone track.
-
DI masks where the trajectory density of DI outflow4 is greater than zero. DI outflow trajectories, identified in ERA5 based on a descent of at least 400 hPa within 48 h (Raveh-Rubin, 2017), are selected in the lower troposphere (up to 700 hPa) as in Catto and Raveh-Rubin (2019).
-
CF masks around CF lines. CF lines are detected in ERA5 reanalysis following Hewson (1998) and Sansom and Catto (2022) and are extended by a 2.5° distance to obtain a 2D object around each front as in Catto and Pfahl (2013).
Feature masks of WCB inflow and ascent, DI outflow, and CF area correspond to connected objects5 satisfying the conditions above; examples are shown in Fig. 1b. Furthermore, based on the “impact area criteria” described in Sect. 3, each feature mask is attributed – or not – to any of the co-occurring Mediterranean cyclones; cycloneless features are labelled “isolated”.
2.4 Notation
We express the percentage frequency of an event e as %, where n(e) is the number of events, and N is the total number of time steps. The percentage frequency of e conditional on event f is represented by %, where ∧ indicates the co-occurrence of two events (∨ indicates the occurrence of at least one of the two events).
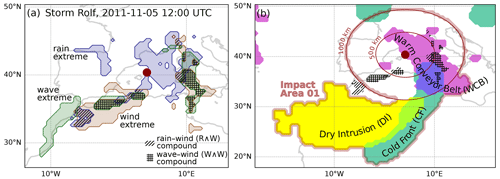
Figure 1The dark red dot indicates Cyclone Rolf's centre at 12:00 UTC on 5 November 2011 (a) with extreme and compound events and (b) with cyclone features and compound events. Rain, wind, and wave extremes are denoted with blue, brown, and green contours and shading; rain–wind compounds are denoted with slantwise hatching; wave–wind compounds are denoted with cross hatching. Dry intrusions, cold fronts, and warm conveyor belts are shown as yellow, aquamarine, and magenta objects, while thin red contours indicate the 500 and 1000 km radii around the centre and the thick red contour encircles the cyclone's impact area IA01 (definition in Sect. 3).
The impact area of a cyclone is the region within which we suppose an event (e.g. a compound extreme) to be related to the presence of that cyclone. In the literature on extratropical cyclones many possibilities are explored:
-
geometric definitions using a specific radius around the cyclone centre (e.g. a 1000 km radius in Owen et al., 2021; a 1100 km radius in Utsumi et al., 2016; variable radii in Hepworth et al., 2022);
-
dynamical definitions based on closed SLP contours, as in Pfahl and Wernli (2012), Papritz et al. (2014), and Dowdy and Catto (2017);
-
in some cases, the contribution of dynamical features related to the presence of the cyclones is also taken into account (e.g. cold fronts in Papritz et al., 2014) or advocated for (Pfahl and Wernli, 2012).
Mediterranean cyclones are on average weaker, smaller, and shorter-lived than their North Atlantic equivalents (Trigo et al., 1999; Trigo, 2006; Čampa and Wernli, 2012; Campins et al., 2011). However, a Mediterranean cyclone's impact area must account for the interactions of the induced atmospheric flow with coastal boundaries and orographic features, since these modify the spatial distribution of the surface impacts (Pfahl, 2014; Houze, 2012; Obermann-Hellhund, 2022; Owen et al., 2021; Flaounas et al., 2019) compared with a conceptual airflow model of an extratropical cyclone over the ocean (Carlson, 1980; Wernli and Davies, 1997; Schultz, 2001). The position of a cyclone centre relative to the coast and to the Mediterranean Sea, the main source of moisture, also determines the character of the impacts (Jansa et al., 2001; Pfahl, 2014).
Reconciling the above aspects, and based on the observation of the synoptic maps and the extreme impacts of the 86 Mediterranean cyclone tracks occurring in the year 19806, we define an impact area for Mediterranean cyclones (labelled IA01) as a fixed central area, a circle of 1000 km radius from the cyclone centre7, eventually extended by the feature masks satisfying the conditions below:
-
cold front masks intercepting a circle of 500 km radius from the cyclone centre
-
warm conveyor belt masks intercepting a circle of 500 km radius from the cyclone centre
-
dry intrusion masks intercepting a circle of 1000 km radius from the cyclone centre.
The interception criteria are satisfied when the concerned feature masks overlap in at least one grid point (see an example of IA01 for cyclone Rolf in Fig. 1).
In this work we prefer a broad impact area definition (IA01) to a narrower definition based on a central area of 500 km radius (henceforth IA02) for three reasons: (i) IA01 allows for the attribution of extreme winds and waves occurring at a considerable distance from the cyclone centre (Nissen et al., 2010; Pfahl, 2014; Field and Wood, 2007; Cornér et al., 2024); (ii) in spite of being broad for the identification of cyclone-related precipitation extremes, IA01 captures distant rainfall events resulting from the interaction of the cyclonic flow with orography (Pfahl, 2014; Owen et al., 2021); (iii) IA01 allows for comparability between rain–wind and wave–wind cyclone statistics. The differences between IA01 and IA02 are discussed in Appendix A. We also mention that the results using IA01 largely correspond to those using a fixed 1000 km radius impact area (not shown).
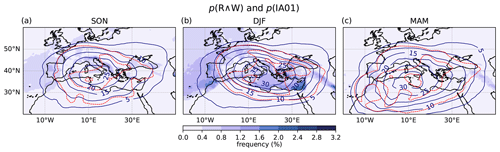
Figure 2Frequency of the R∧W compound (p(R∧W), shading) and of the cyclone impact area (p(IA01), blue contours) in (a) autumn – SON, (b) winter – DJF, and (c) spring – MAM. The frequency is expressed in percentage occurrence per time step for each season. The negative difference between p(IA02) and p(IA01) is represented by the dashed red contours at 5 % intervals.
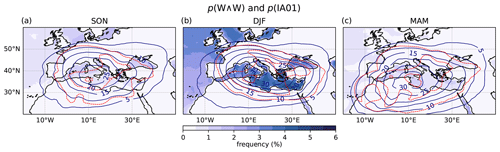
Figure 3As in Fig. 2 but for the W∧W compound (note difference in colour scale).
The frequency of compound extremes is unevenly distributed across regions and seasons. Concomitant rain–wind extremes are most frequent in winter (Fig. 2) as a result of a winter peak in the univariate extremes (Fig. S2) and in the compounding ratio (; see Fig. S5). The winter R∧W events occur most often in the eastern Mediterranean, specifically along the coastlines of Israel and North Africa and of the eastern Adriatic Sea. In agreement with Raveh-Rubin and Wernli (2015) (their Figs. 3 and 4), the autumn spatial maximum in the R∧W frequency is shifted to the central and western Mediterranean, where differences from the winter season are small. The spring frequency, limited by the number of extreme rainfall events (Fig. S2c), is substantially lower everywhere (on average8 about half of the autumn and a quarter of the winter frequencies). The R∧W compounding ratio is generally higher over coastal regions facing northwest (Fig. S5) and over orography (consistent with Fig. 2b in Owen et al., 2021, and Fig. 4a in Martius et al., 2016).
Concomitant wave and wind extremes also occur most frequently in winter but, differently from R∧W compounds, show a greater spatial homogeneity within the basin (Fig. 3). A shift in the position of the spatial maximum in compound frequency, from the western Mediterranean in autumn to the eastern Mediterranean and the Black Sea in winter, is detected. Spring W∧W extremes display relatively small spatial variability, with an average incidence similar to autumn values. The high compounding ratio across seasons (Fig. S6) is a consequence of the strong physical dependence of wave height on the surface wind field (e.g. Komen et al., 1996). Nevertheless, both the frequency and the compounding ratio of W∧W events are generally higher in the Mediterranean than in the western Atlantic (compare to Fig. 1 in Catto and Dowdy, 2021).
The winter peak in compound extremes coincides with the highest frequency of cyclones (p(IA01), blue contours in Fig. 2). The Mediterranean cyclone density maximum shows a peak over the Italian peninsula in the autumn season, which expands towards the southeast in winter and shifts towards the southwest in spring (extending to northwestern Africa). The cyclone density distribution is spatially smoother and displays different regional and seasonal patterns compared with the compound frequency; factors such as geographical exposure and seasonal changes in the local climatological weather conditions account for the difference in the distribution of compound extremes.
4.1 Rain–wind compounds (R∧W)
The frequency of R∧W events within cyclones' impact areas (Fig. 4a–c) is highest in winter, with a 1 % to 10 % frequency over most of the basin, and lowest in spring – below 1 % because of cyclones producing less rainfall (Flaounas et al., 2019).
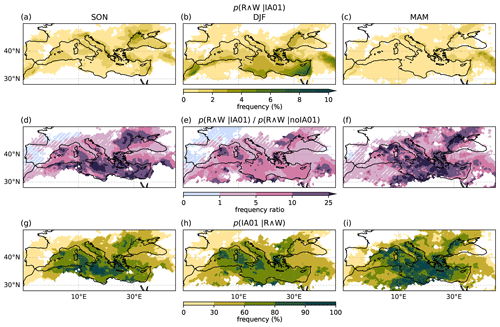
Figure 4(a–c) The frequency of the R∧W compound conditional on the presence of a cyclone, (d–f) the ratio of the R∧W compound frequency during cyclone occurrence over the R∧W compound frequency during times when cyclones do not occur, (g–i) the cyclone frequency conditional on the presence of R∧W compounds. Results are shown by season (autumn – SON, winter – DJF, spring – MAM). In panels (d–f) the slanted white hatching indicates where the compound frequency during cyclone occurrence is not significantly different from the compound frequency during no cyclone occurrence according to a two-sided z test at a 95 % confidence level (Wilks, 2011). Grid points displaying fewer than four (IA01) events are masked in white.
The presence of a nearby cyclone increases the compound frequency (Fig. 4d–f), particularly in the transition seasons compared with winter and in the eastern and central Mediterranean, where a 10-fold amplification is not uncommon. The maps in Fig. 4g–i, representing the proportion of cyclone-related R∧W compounds, show that autumn and spring events often co-occur with cyclones (on average9 50 % and 58 % of the R∧W events co-occur with cyclones in autumn and spring, compared with 48 % in winter; differences are locally larger). Moreover, the association with cyclones is much stronger for R∧W compounds than for univariate extremes (compare to Fig. S4). These results are not sensitive to the specific percentile threshold (see Fig. S3, using the 95th percentile).
The relation between the quantities p(IA01) and IA01) in Fig. 4 follows Bayes' theorem (i.e. p(IA01IA01) ⋅ p(IA01)). The relation states that the fraction of cyclone-related compounds decreases with the ratio of cyclones over compounds, implying a smaller proportion of cyclone-related events in winter because of the distinct decline in p(IA01). The occurrence of winter R∧W events outside cyclones' impact areas is particularly high in the eastern and southern sectors of the Mediterranean (Fig. 4h) and coincides with where larger fractions of R∧W compounds co-occur with isolated10 dynamical features. This is shown in Fig. 5b and e, where unhatched areas indicate where less than 90 % of the features occurring with compounds are related to a cyclone.
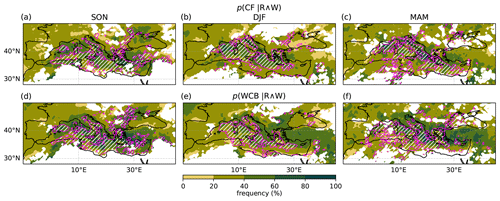
Figure 5Frequency of (a–c) CFs and (d–f) WCBs conditional on R∧W compound occurrence in (a, d) autumn – SON, (b, e) winter – DJF, and (c, f) spring – MAM. Note that in this plot we consider all CFs and WCBs, independently of whether they are part of a cyclone's impact area; magenta contours and slanted white hatching identify regions where more than 90 % of the features are related to a cyclone (definition in Sect. 3). Note that since compounds occurring within superposing CFs and WCBs contribute to the statistics of both, the sum of their conditional frequencies can exceed 100 %.
We next separate the influence of the Mediterranean cyclones' dynamical features, as in cold fronts (CF-IA), regions of warm conveyor belt inflow (WCBin-IA) and ascent (WCBas-IA), and dry intrusions (DI-IA), from the rest of the impact area (IA-noDF). Figure 6a shows the feature co-occurring most frequently with R∧W events, and Fig. 6b represents the feature maximising the R∧W event incidence; both are computed year-round because seasonal differences are small. Over the whole Mediterranean region, the occurrence of R∧W events is maximised below the ascending branch of WCBs (Fig. 6b), while the WCB inflow is more important over land and mountain reliefs during the winter season (not shown). On the other hand, when selecting the most frequent feature during R∧W compounds (Fig. 6a), WCB ascent is still predominant in the northern Mediterranean close to mountain reliefs or on sea-based grid points facing west- or south-oriented coasts (see also Fig. 7c for precipitation extremes in Pfahl et al., 2014). CFs show the highest frequency on the rest of the basin (see also Fig. 4b for precipitation extremes in Catto and Pfahl, 2013), except around the North African coasts, where the impact area without dynamical features is relevant. The differences between Fig. 6a and b derive from the fact that in Fig. 6b the results are normalised by the features' individual frequencies (see Fig. S7).
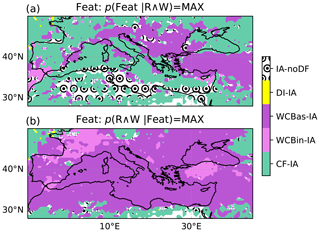
Figure 6(a) The most frequent cyclone feature during R∧W compounds; (b) the cyclone feature associated with the highest occurrence of R∧W compounds. We compute the conditional probability maxima amongst five cyclone features: the cold front (CF-IA), the warm conveyor belt inflow (WCBin-IA) and ascent (WCBas-IA) regions, the dry intrusion (DI-IA), and the 1000 km radius around the cyclone centre after removal of the aforementioned dynamical features' masks (IA-noDF). Compounds occurring within superposing CFs, WCBs, and DIs contribute to the statistics of all the objects concerned. Because of a weak variability across seasons, the results are provided using year-round data.
4.2 Wave–wind compounds (W∧W)
In the cyclone neighbourhood, wave–wind extremes occur much more frequently than rain–wind extremes (see Figs. 7a–c and 4a–c). The main similarity between the two types of compounds is the higher compound frequency for winter cyclones (in Fig. 7b W∧W reaches and locally surpasses a 10 % frequency) and the tendency for the spatial peak in the cyclone compound density to shift from the western Mediterranean in autumn to the southeastern basin in winter. This second aspect is in agreement with the geographical shift in W∧W and impact area frequency (Fig. 3).
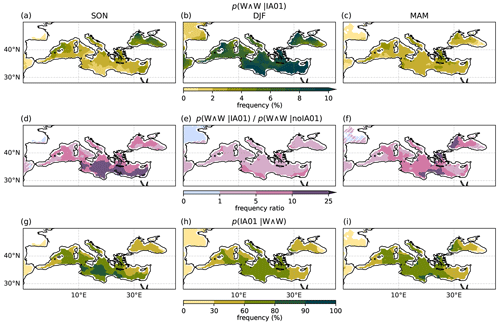
Figure 7As in Fig. 4 but for the W∧W compound.
The proportion of W∧W events associated with cyclones peaks in the central part of the basin and is quite constant across seasons (on average11 ∼50 %, Fig. 7g–i). Moreover, the percentage of W∧W co-occurrence with cyclones, rarely exceeding an 80 % level, is smaller compared with that of R∧W events (compare Figs. 7g–i and 4g–i) and comparable to the rates computed for the univariate extremes (see Fig. S4c–i). This suggests cyclones are less relevant for W∧W than for R∧W compounding. The W∧W compound frequency increases by approximately a factor of 5 in the cyclone neighbourhood (Fig. 7d–f) but exhibits a strong inter-seasonal variability, which is likely related to a larger fraction of winter events occurring with isolated dynamical features (Fig. S9).
Dry intrusions, which drive intense surface wind gusts (Raveh-Rubin, 2017) and induce impacts on surface and atmospheric conditions (e.g. dust uplift; see Fluck and Raveh-Rubin, 2023), are the dynamical feature showing the highest frequency of wave–wind compounding over the western and eastern parts of the basin (Fig. 8b). WCB ascent is important for W∧W in the central Mediterranean (comprising the Adriatic Sea and most of the Tyrrhenian and Ligurian seas), southeast of Spain, and in the easternmost part of the basin. In contrast, CFs are the most frequent feature detected during W∧W events in the northern Mediterranean, replaced by the feature-free 1000 km radius in the southern Mediterranean (Fig. 8a).
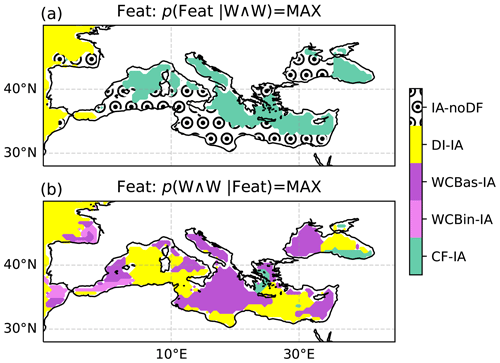
Figure 8As in Fig. 6 but for the W∧W compound.
4.3 Cyclone clusters relevant for compounding
In this section we refer to the Mediterranean cyclone clusters described in Givon et al. (2024a) and listed in Table 1. While the association of each cluster with surface compounds from a cyclone-centric view is discussed in Rousseau-Rizzi et al. (2023), here we show the cyclone clusters with the highest frequency of R∧W and W∧W from a (geographical) Eulerian perspective.
Baroclinic clusters 4 and 1 are, in order of importance, the most relevant for both types of compounds in autumn and winter (Figs. 9 and 10). In winter we also find a strong regional association of cluster 8 with R∧W events over the western Mediterranean and of cluster 5, typical of transition seasons, with both compound extremes in the central and southeastern Mediterranean (the R∧W region in Fig. 9e is to the east of the W∧W region in Fig. 10e, as expected in the schematic of Mediterranean cyclone impacts by Raveh-Rubin and Wernli, 2016). In the autumn season cluster 7, corresponding to daughter lows often originating over North Africa in a relatively dry environment, is important in the southwestern Mediterranean and in the easternmost part of the basin but only relative to W∧W occurrence (Fig. 10a and d).
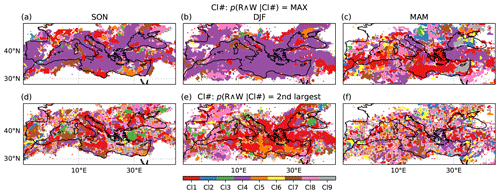
Figure 9The first and second cyclone cluster number (Cl#) maximising the R∧W compound frequency in (a, d) autumn – SON, (b, e) winter – DJF, and (c, f) spring – MAM. Note that compounds occurring within multiple cyclones' IA01 contribute to the frequencies of all the relevant clusters. Note that grid points displaying fewer than four (IA01) events are masked in white and that statistical noise results in irregular and intermittent colour patches.
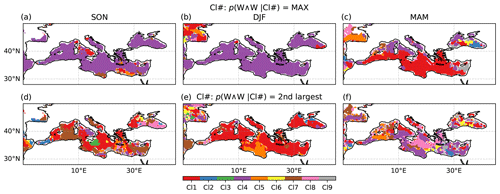
Figure 10As in Fig. 9 but for the W∧W compound.
In spring the dominant-cluster distribution is more diverse, with cluster 1 maximising the compound occurrence over most of the basin. Cluster 4, because of its frequency peaking earlier in winter, becomes the second most important cluster (first in the central-northern Mediterranean). Cluster 9, corresponding to cut-off lows, acquires relevance for both types of compounds in the eastern Mediterranean and in the Black Sea, while in the western Mediterranean W∧W events are often linked to clusters 5 and 7 and R∧W events to the moister cluster 8. The sparse distribution of cluster 8 extends to a wide central Mediterranean region, where it is also associated with frequent W∧W events (Figs. 9c, f and 10c, f). Cluster 2, typically comprising large, baroclinic winter cyclones, although identified by Rousseau-Rizzi et al. (2023) as the cluster with the highest simultaneity and overlap of rain and wind extremes, shows the highest R∧W compound frequency only in spring over the Balkan peninsula. This is likely a result of its relatively low occurrence frequency compared with clusters 1 and 4 (Fig. S8).
We note that clusters 1 and 4, dominant across regions and seasons, show an average R∧W and W∧W frequency that is only slightly higher than the aggregated statistics in Figs. 4a–c and 7a–c, whereas summer clusters 6 and 9 and cluster 2, maximising compound frequency over limited domains, show an amplification of the overall cyclone compound frequency by a factor generally larger than 5. The other clusters, typical of the transition seasons, show an intermediate increase in R∧W and W∧W frequencies (factors close to 3) compared with the aggregated statistics. Note that values are computed seasonally over the regions where each cluster maximises the compound probability, i.e. colour-filled areas in Figs. 9a–c and 10a–c.
Frequency maps of rain–wind and wave–wind clustering show a pronounced winter peak and an eastward shift during the transition from autumn to spring (Fig. 2), following the density of the Mediterranean cyclones' impact area but with a more distinct seasonal cycle. Indeed, seasonal differences in compound frequencies are induced by climatological differences and by higher compounding around winter cyclones (Figs. 4a–c and 7a–c) and only at a second order by the cyclone frequency.
During the transition seasons, compounds – R∧W in particular – happen mostly within the impact area of a cyclone (Figs. 4g–i and 7g–i), while in winter they show a weaker relation to cyclones and happen more frequently with isolated dynamical features (see Figs. 4, 5, 7, and S9). Moreover, the association with cyclones is stronger for R∧W extremes than for separate univariate extremes (compare to Fig. S4). These results are consistent with Owen et al. (2021), who, with regard to winter R∧W compounds, highlight the importance of cold frontal regions far from storm centres and cite atmospheric rivers (ARs) as a potential driver (see also Hénin et al., 2021, for regional patterns). The high winter AR frequency in the region and the associated potential increase in the likelihood of R∧W events support such a hypothesis (Guan and Waliser, 2015; Waliser and Guan, 2017). Nevertheless, the association between ARs and rain–wind compounds around the Mediterranean Basin and the superposition of AR and WCB objects (see discussion in Ralph et al., 2017) have yet to be systematically explored.
Geographical peculiarities, listed in the following, are more pronounced for R∧W compared with W∧W compounds because of varying seasonal and regional moisture availability and the strong effects of orography and coastlines on precipitation (e.g. Flaounas et al., 2019; Jansa et al., 2001; Pfahl, 2014). We also acknowledge that the statistical links between W∧W events and cyclones in the northwestern Mediterranean Basin (shown in Fig. 7) are sensitive to the inclusion of Atlantic cyclones, inducing strong wind impacts in the region (Pfahl, 2014; Nissen et al., 2010).
-
The Gulf of Lion shows a year-round minimum in the R∧W compound to extreme ratio (Fig. S5) and a maximum in the W∧W ratio (Fig. S6). The small rain–wind compounding ratio is related to dry northerly mistral-wind events that bear no rainfall, yet are known to induce lee cyclogenesis in the form of Genoa lows (Givon et al., 2024b). The systems formed in this way carry intense precipitation elsewhere, mainly over the Italian and Balkan peninsulas (Givon et al., 2021). The maximum in the W∧W compounding ratio is related to the high wind intensity in the region (Givon et al., 2024b, and the peak in the 98th percentile wind gust in Fig. S1e) and to the physical dependence between the wave height and the wind field (e.g. Komen et al., 1996; Gentile et al., 2021).
-
In eastern Spain, southwestern France, and the eastern alpine region a low proportion of R∧W events is associated (year-round) with Mediterranean cyclones (Fig. 4g–i). Despite the exclusion of Atlantic tracks, our results correspond qualitatively to those using global storm tracks by Owen et al. (2021), who relate the low co-occurrence of cyclones and R∧W compounds north of the Mediterranean (their Figs. 10–12) to the fact that regional precipitation extremes are usually brought by Mediterranean cyclones, while wind extremes are often attributed to Atlantic weather systems. Similar findings are discussed in Pfahl (2014) and Nissen et al. (2010).
-
The relation between cyclones and compounds is strong in the northwest of the Black Sea (Fig. 4) because of a secondary peak in Mediterranean cyclone track density over the basin (see Fig. 13b in Flaounas et al., 2023).
Regional differences in compound–cyclone statistics are further influenced by the varying size and type of Mediterranean cyclones. For example, the high compound frequency around winter cyclones in the southeastern Mediterranean (Figs. 4 and 7) can be attributed to geographical exposure – the coast faces northwest, the direction from which most weather systems propagate (see also Fig. 4a in Martius et al., 2016) – and to the small size of cyclones, which favours the superposition of wind and rain impacts close to the core of the system (see Fig 10 in Raveh-Rubin and Wernli, 2015). Our analyses confirm the results of Raveh-Rubin and Wernli (2015) by indicating a high ratio of compounds around eastern Mediterranean cyclones (Fig. 4b); this extends to the case when the impact area is restricted to a fixed 500 km radius (not shown).
Concerning the size of Mediterranean cyclones, we note that reducing the impact area extension (IA02 definition; see Sect. 3) does not determine qualitative changes in the results (compare Figs. 4, 7, A1, and A2). However, further analyses in Appendix A demonstrate how the wider impact area IA01 is well suited for detecting cyclone-related wave–wind compounds (see Raveh-Rubin and Wernli, 2015, 2016; Cornér et al., 2024), while the more stringent IA02 performs well in identifying cyclone-related rain–wind compounds. The suitability of IA02 for rain impacts partly verifies the attribution approach by Flaounas et al. (2018).
The Mediterranean cyclone clustering introduced in Givon et al. (2024a) allows us to study the spatial distribution of the cyclone types associated with the highest compound frequency:
-
Clusters 1 and 4, typically strong baroclinic cyclones, show the strongest connection with regional compounds across seasons because of the high rainfall rates and the wide wind footprints (Givon et al., 2024a; Rousseau-Rizzi et al., 2023). Their peak winter frequency helps to explain the maximum compound occurrence around winter cyclones (Fig. 4).
-
Cluster 8 cyclones, which are relatively stationary and characterised by strong convective activity, show a high likelihood of R∧W compounds across multiple seasons and regions, despite being associated with surface hazards of limited spatial extension (see Figs. 5 and 11 in Givon et al., 2024a).
-
Cluster 5 and 7 cyclones are generated by the interaction of PV streamers with topography. Cluster 7 is relevant for W∧W extremes and cluster 5 for both compounds in winter and W∧W in spring.
-
Cluster 9, i.e. cut-off lows typical of the extended summer season, emerges in spring over the eastern Mediterranean in connection with both types of compounds.
The results of the cluster analysis are independent of the specific definition of impact area (Sect. 3 and Appendix A).
Additionally, our study highlights the importance of cyclone-related dynamical features for the two types of compound extremes. The presence of WCB ascent maximises the likelihood of R∧W events across the Mediterranean region, although a frequency-weighed analysis suggests the importance of diverse airstreams in the central and northern Mediterranean (CFs over the sea, WCBs over land and close to west- and south-facing coastal boundaries) and of the dynamical-feature-free impact area along the southern Mediterranean coast (Fig. 6). The results for the southern coast are probably linked to a smaller size of the cyclones (Raveh-Rubin and Wernli, 2015) and to the presence of dry, weakly precipitating WCBs from North Africa (Ziv et al., 2010). W∧W events are most likely below DIs and WCBs, although they co-occur frequently with CFs in the northern Mediterranean and with the dynamical-feature-free impact area in the southern basin (Fig. 8). Results remain qualitatively unchanged when considering all dynamical features independently of their attribution to cyclone centres (not shown).
Although in the literature the WCB is mainly connected to precipitation (Pfahl et al., 2014), in the Mediterranean region we find a high superposition of WCBs with rain–wind and wave–wind compounds (20 %–80 % and 0 %–40 % of the R∧W and W∧W events occur below a WCB, Fig. 5d–f). Regarding the plausibility of wind extremes below WCBs, a WCB jet in the form of strong geostrophic winds is not uncommon during the cyclone intensification stage in the Atlantic (Hewson and Neu, 2015; Figs. 7 and 9 in Eisenstein et al., 2023; Fig. 5 in Wernli, 1997). Such a “warm jet” usually starts in the lower troposphere ahead of the cold front and ascends in the mid-troposphere to the east of the cyclone centre. Moreover, convective activity embedded within the WCB (Flaounas et al., 2018), i.e. in pre-frontal environments (Catto et al., 2015; Pacey et al., 2023) or in regions of strong ascent near the cyclone centre (Flaounas et al., 2015), is known to induce downward momentum transport from the mid-troposphere through convective downdraughts (see discussion in Hewson and Neu, 2015) and hence may favour the presence of strong surface wind gusts within the WCB. Wind channelling and forced ascent induced by coastal and orographic features (Carrera et al., 2009) combined with higher precipitation efficiency over land and orography (Flaounas et al., 2019; Pfahl, 2014; Houze, 2012) may further contribute to the overlap of R∧W compounding and WCBs (see compound pattern and brown isohypse in Fig. 9 of Rousseau-Rizzi et al., 2023).
We quantify the relation between compound weather extremes (rain–wind and wave–wind) and the presence of nearby cyclones in the extended Mediterranean region. Based on a new definition of cyclone impact area, i.e. a fixed radius around the cyclone centre and overlapping fronts and cyclone airstreams (Sect. 3), we answer the research questions listed in Sect. 1:
-
We verify that the presence of a cyclone increases the odds of compound incidence, particularly in the transition seasons – by at least a factor of 5 – and for R∧W events. Winter cyclones, even if they are less determinant for the occurrence of compound extremes, display the strongest compound frequency (1 %–10 % for R∧W, more than 5 % for W∧W). Differences between autumn and spring R∧W statistics are related to a lower moisture availability and to a southward extension of the storm track in the spring season. Additionally, R∧W extremes show a stronger link to cyclones than the two separate univariate extremes (Sect. 4).
-
R∧W compounding and W∧W compounding tend to overlap with different dynamical features around a cyclone, showing a distinct regional dependence. In general, the probability of rain–wind extremes around cyclones is maximised in areas of warm conveyor belt ascent (∼7 % of the cases) and that of wave–wind extremes is maximised below dry intrusion outflow and warm conveyor belts (more than 10 % of the cases). Furthermore, in the northern Mediterranean region, cold fronts are frequently detected during compound events, whereas in the south, this is the case for the feature-free cyclone impact area (Sect. 4.1, 4.2).
-
Baroclinic cyclones (clusters 1 and 4 from Givon et al., 2024a) maximise the occurrence of compounds across all seasons, and their peak occurrence in winter explains the highest cyclone compound density for the winter season (see point 1). Other cyclone categories are relevant at a local and seasonal scale. For example, cluster 8 is associated with frequent R∧W extremes over different seasons and parts of the basin (Sect. 4.3).
Crucial questions remain regarding the role of orography and coastal boundaries, associated with wind channelling and forced ascent, in the generation of compound R∧W and W∧W events in the Mediterranean region. In particular, our results point to the importance of warm conveyor belts (WCBs) for rain–wind and wave–wind compounding in the northern Mediterranean, even though WCBs are principally known for rainfall impacts. Interactions of the WCBs with orography and coastal boundaries, together with the WCB jet and embedded convection, may be important for the generation of surface rain–wind extremes. Additionally, the role of atmospheric rivers, potentially overlapping with WCBs, in driving Mediterranean rain and rain–wind extremes deserves targeted analyses.
Future research could separate compounds in events induced by the synoptic circulation or by deep convection embedded around the cyclone. Based on previous literature (e.g. Flaounas et al., 2018; Givon et al., 2024a; Berthou et al., 2022), we expect a different intensity, spatial extent, and distribution (geographical and relative to the cyclone centre) of the two types of events. Additional insight into convective environments and hazards around Mediterranean cyclones will be addressed in a separate paper.
Finally, we expect Mediterranean compounds in a future warmer climate to change in distribution and intensity, following changes in the cyclones' frequency and physical properties (e.g. Pfahl and Wernli, 2012; Manning et al., 2024). Recent trends show a decrease in the number of large baroclinic systems and an increase in the number of small cyclones typical of the extended summer season (Givon et al., 2024a). This agrees with end-of-21st-century climate projections indicating a general reduction in the frequency and overall intensity of Mediterranean cyclones (Reale et al., 2022). The connection between projected Mediterranean cyclone changes and the future regional distribution of compound extremes has yet to be examined.
Various possibilities of impact areas were tested before establishing the definition of IA01 described in Sect. 3. Particularly interesting is the comparison between the results using IA01 and IA02, where the latter corresponds to a smaller central area (radius of 500 km instead of 1000 km) extended by the same dynamical features as IA01 (see Sect. 3). The frequency difference, p(IA02)−p(IA01), is shown in Fig. 2. Limit cases of IA01 and IA02 compound density correspond to the following:
- i.
All cyclone compounds (C) captured by IA01 fall within IA02,
- ii.
The density of cyclone compounds is uniformly distributed within IA01,
In (i) the best choice is IA02, since IA01 overestimates the extension of the expected impacts and underestimates p(C|IA01), the compound occurrence around the cyclone; in (ii) IA01 is adequate, since IA02 underestimates the area of influence and p(IA02|C), the ratio of cyclone-related compounds.
In Fig. A1 we show the conditional probabilities between cyclones and R∧W compounds obtained using IA02, while hatching indicates the percentage levels of the difference from the same quantities computed using IA01 (full IA01 statistics in Fig. 4). Differences between IA02) and IA01) are positive and often exceed the IA01 values by more than 25 % (slanted hatching, Fig. A1a–c), indicating a higher frequency of compounds within the smaller impact area. The largest differences in R∧W incidence are detected in the transition seasons, when cyclones are smaller and severe hazards are distributed closer to the cyclone centre (within IA02) (Givon et al., 2024a), and in the region separating the Mediterranean and the Black Sea. Hatching along the North African coast in Fig. A1d–f also highlights a substantial regional decrease in p(IA02) relative to p(IA01). This probably relates to IA02 missing distant compound events, which result from the interaction between the large-scale cyclonic flow and the African topography.
The comparison of IA01 and IA02 for W∧W events diverges substantially from that of R∧W compounds (compare Figs. A2 and A1). The probability of W∧W within IA02 (Fig. A1a–c) does not increase nearly as much as in the case of R∧W, while the choice of IA02 strongly reduces the proportion of cyclone-related compounds throughout the southern Mediterranean and over the Black Sea (Fig. A1d–f).
In summary, rain–wind compounds, showing a large increase in compound frequency close to cyclones when using IA02, are reminiscent of limit-case (i) with a preference for IA02 (except over the southern Mediterranean coast). Differently, wave–wind compounds, because of small differences in the compound frequency within IA02 and IA01 and because of an important fraction of compounds occurring outside IA02 but within IA01, are relatable to limit-case (ii) with a preference for IA01. This is consistent with the expected geographical distribution of cyclone impacts, with intense rainfall and convective activity happening close to the centre and strong wind and waves occurring further away. The result validates previous hazard-dependent choices of Mediterranean cyclone impact area (e.g. Flaounas et al., 2018; Cornér et al., 2024); in this work, for the sake of methodological consistency across results, we use the IA01 definition.
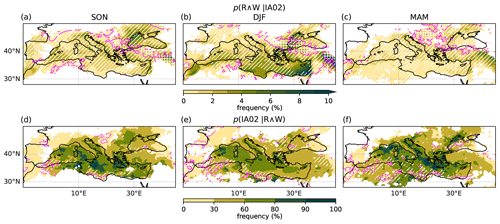
Figure A1(a–c) The frequency of R∧W compounds conditional on the presence of a cyclone; (d–f) the cyclone frequency conditional on the presence of R∧W compounds, using an impact area with a reference radius of 500 km (IA02). A difference between IA02 and IA01 frequencies by more than 25 % of the IA01 value is denoted by the white slanted hatching; a 50 % difference is highlighted by the white cross hatching within the magenta contours (dashed for negative differences). Seasonal results for autumn (SON), winter (DJF), and spring (MAM) are displayed in the left, centre, and right panels, respectively. Grid points displaying fewer than four (IA02) events are masked out.
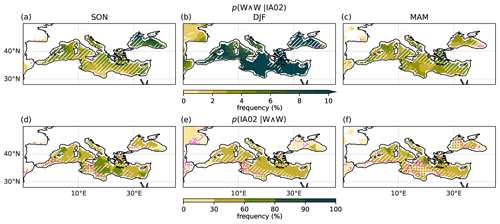
Figure A2As in Fig. A1 but for the W∧W compound.
The code is not publicly accessible. The computations used for this work reduce to the simple frequency statistics described in Sect. 2.4.
ERA5 reanalysis is publicly available at https://doi.org/10.24381/cds.adbb2d47 (Hersbach et al., 2023). Mediterranean cyclone tracks are provided as a supplement of Flaounas et al. (2023).
The supplement related to this article is available online at: https://doi.org/10.5194/wcd-5-1043-2024-supplement.
AP, OM, SRR, and JLC conceived the study. YG provided data and gave advice on the cyclone cluster analysis. AP performed the analyses and prepared the paper with contributions from all co-authors.
At least one of the (co-)authors is a member of the editorial board of Weather and Climate Dynamics. The peer-review process was guided by an independent editor, and the authors also have no other competing interests to declare.
Publisher's note: Copernicus Publications remains neutral with regard to jurisdictional claims made in the text, published maps, institutional affiliations, or any other geographical representation in this paper. While Copernicus Publications makes every effort to include appropriate place names, the final responsibility lies with the authors.
Alice Portal is grateful for insightful discussions with David Stephenson, Matthew Priestley, and Emmanouil Flaounas and for the constructive contributions of the two reviewers. The authors are thankful to all the collaborators who produced and provided the CF and DI datasets, as well as to Heini Wernli and Michael Sprenger for kindly providing the WCB dataset. The research contributes to the efforts of COST Action CA19109 under MedCyclones – European network for Mediterranean cyclones in weather and climate.
This research has been supported by the Schweizerischer Nationalfonds zur Förderung der Wissenschaftlichen Forschung (grant no. IZCOZ0_205461). Shira Raveh-Rubin received funding from the Israel Science Foundation (grant no. 1242/23) and the De Botton Center for Marine Science at the Weizmann Institute of Science.
This paper was edited by Irina Rudeva and reviewed by Paolo De Luca and one anonymous referee.
Berthou, S., Roberts, M.J., Vannière, B., Ban, N., Belušić, D., Caillaud, C., Crocker, T., de Vries, H., Dobler, A., Harris, D., and Kendon, E. J.: Convection in future winter storms over Northern Europe, Environ. Res. Lett., 17, 114055, https://doi.org/10.1088/1748-9326/aca03a, 2022. a
Bertotti, L. and Cavaleri, L.: The predictability of the “Voyager” accident, Nat. Hazards Earth Syst. Sci., 8, 533–537, https://doi.org/10.5194/nhess-8-533-2008, 2008. a
Čampa, J. and Wernli, H.: A PV perspective on the vertical structure of mature midlatitude cyclones in the Northern Hemisphere, J. Atmos. Sci., 69, 725–740, 2012. a
Campins, J., Genovés, A., Picornell, M., and Jansà, A.: Climatology of Mediterranean cyclones using the ERA-40 dataset, Int. J. Climatol., 31, 1596–1614, 2011. a, b
Carlson, T. N.: Airflow through midlatitude cyclones and the comma cloud pattern, Mon. Weather Rev., 108, 1498–1509, 1980. a, b, c
Carrera, M. L., Gyakum, J. R., and Lin, C. A.: Observational study of wind channeling within the St. Lawrence River Valley, J. Appl. Meteorol. Clim., 48, 2341–2361, 2009. a
Catto, J. L. and Dowdy, A.: Understanding compound hazards from a weather system perspective, Weather Clim. Extrem., 32, 100313, https://doi.org/10.1016/j.wace.2021.100313, 2021. a, b, c
Catto, J. L. and Pfahl, S.: The importance of fronts for extreme precipitation, J. Geophys. Res.-Atmos., 118, 10–791, 2013. a, b, c
Catto, J. L. and Raveh-Rubin, S.: Climatology and dynamics of the link between dry intrusions and cold fronts during winter. Part I: global climatology, Clim. Dynam., 53, 1873–1892, 2019. a
Catto, J. L., Madonna, E., Joos, H., Rudeva, I., and Simmonds, I.: Global relationship between fronts and warm conveyor belts and the impact on extreme precipitation, J. Climate, 28, 8411–8429, 2015. a
Cavaleri, L., Bertotti, L., Torrisi, L., Bitner-Gregersen, E., Serio, M., and Onorato, M.: Rogue waves in crossing seas: The Louis Majesty accident, J. Geophys. Res.-Oceans, 117, C00J10, https://doi.org/10.1029/2012JC007923, 2012. a
Cornér, J. S., Bouvier, C. G. F., Doiteau, B., Pantillon, F., and Sinclair, V. A.: Classification of North Atlantic and European extratropical cyclones using multiple measures of intensity, EGUsphere [preprint], https://doi.org/10.5194/egusphere-2024-1749, 2024. a, b, c
Dafis, S., Rysman, J.-F., Claud, C., and Flaounas, E.: Remote sensing of deep convection within a tropical-like cyclone over the Mediterranean Sea, Atmos. Sci. Lett., 19, e823, https://doi.org/10.1002/asl.823, 2018. a
De Luca, P., Messori, G., Pons, F. M., and Faranda, D.: Dynamical systems theory sheds new light on compound climate extremes in Europe and Eastern North America, Q. J. Roy. Meteorol. Soc., 146, 1636–1650, 2020. a
Dowdy, A. J. and Catto, J. L.: Extreme weather caused by concurrent cyclone, front and thunderstorm occurrences, Sci. Rep., 7, 40359, https://doi.org/10.1038/srep40359, 2017. a, b
DWD: Selected Significant Events, https://www.dwd.de/EN/ourservices/rcccm/int/rcccm_int_sse.html?nn=495490&cl2Categories_Jahr=2011&cl2Categories_Monat=rcccm_int_sse_11&cl2Categories_LeistungsId=rcccm_int_sse&lsId=446082 (last access: 9 April 2024), 2011. a
Eisenstein, L., Schulz, B., Pinto, J. G., and Knippertz, P.: Identification of high-wind features within extratropical cyclones using a probabilistic random forest – Part 2: Climatology over Europe, Weather Clim. Dynam., 4, 981–999, https://doi.org/10.5194/wcd-4-981-2023, 2023. a
Fanti, V., Ferreira, Ó., Kümmerer, V., and Loureiro, C.: Improved estimates of extreme wave conditions in coastal areas from calibrated global reanalyses, Commun. Earth Environ., 4, 151, https://doi.org/10.1038/s43247-023-00819-0, 2023. a
Field, P. R. and Wood, R.: Precipitation and cloud structure in midlatitude cyclones, J. Climate, 20, 233–254, 2007. a
Flaounas, E., Raveh-Rubin, S., Wernli, H., Drobinski, P., and Bastin, S.: The dynamical structure of intense Mediterranean cyclones, Clim. Dynam., 44, 2411–2427, 2015. a, b, c
Flaounas, E., Kotroni, V., Lagouvardos, K., Gray, S. L., Rysman, J.-F., and Claud, C.: Heavy rainfall in Mediterranean cyclones. Part I: contribution of deep convection and warm conveyor belt, Clim. Dynam., 50, 2935–2949, 2018. a, b, c, d, e
Flaounas, E., Fita, L., Lagouvardos, K., and Kotroni, V.: Heavy rainfall in Mediterranean cyclones, Part II: Water budget, precipitation efficiency and remote water sources, Clim. Dynam., 53, 2539–2555, 2019. a, b, c, d
Flaounas, E., Davolio, S., Raveh-Rubin, S., Pantillon, F., Miglietta, M. M., Gaertner, M. A., Hatzaki, M., Homar, V., Khodayar, S., Korres, G., Kotroni, V., Kushta, J., Reale, M., and Ricard, D.: Mediterranean cyclones: current knowledge and open questions on dynamics, prediction, climatology and impacts, Weather Clim. Dynam., 3, 173–208, https://doi.org/10.5194/wcd-3-173-2022, 2022. a, b
Flaounas, E., Aragão, L., Bernini, L., Dafis, S., Doiteau, B., Flocas, H., Gray, S. L., Karwat, A., Kouroutzoglou, J., Lionello, P., Miglietta, M. M., Pantillon, F., Pasquero, C., Patlakas, P., Picornell, M. Á., Porcù, F., Priestley, M. D. K., Reale, M., Roberts, M. J., Saaroni, H., Sandler, D., Scoccimarro, E., Sprenger, M., and Ziv, B.: A composite approach to produce reference datasets for extratropical cyclone tracks: application to Mediterranean cyclones, Weather Clim. Dynam., 4, 639–661, https://doi.org/10.5194/wcd-4-639-2023, 2023. a, b, c, d
Fluck, E. and Raveh-Rubin, S.: Dry air intrusions link Rossby wave breaking to large-scale dust storms in Northwest Africa: Four extreme cases, Atmos. Res., 286, 106663, https://doi.org/10.1016/j.atmosres.2023.106663, 2023. a
Froidevaux, P., Schwanbeck, J., Weingartner, R., Chevalier, C., and Martius, O.: Flood triggering in Switzerland: the role of daily to monthly preceding precipitation, Hydrol. Earth Syst. Sci., 19, 3903–3924, https://doi.org/10.5194/hess-19-3903-2015, 2015. a
Gentile, E. S., Gray, S. L., Barlow, J. F., Lewis, H. W., and Edwards, J. M.: The impact of atmosphere–ocean–wave coupling on the near-surface wind speed in forecasts of extratropical cyclones, Bound.-Lay. Meteorol., 180, 105–129, 2021. a
Givon, Y., Keller Jr., D., Silverman, V., Pennel, R., Drobinski, P., and Raveh-Rubin, S.: Large-scale drivers of the mistral wind: link to Rossby wave life cycles and seasonal variability, Weather Clim. Dynam., 2, 609–630, https://doi.org/10.5194/wcd-2-609-2021, 2021. a
Givon, Y., Hess, O., Flaounas, E., Catto, J. L., Sprenger, M., and Raveh-Rubin, S.: Process-based classification of Mediterranean cyclones using potential vorticity, Weather Clim. Dynam., 5, 133–162, https://doi.org/10.5194/wcd-5-133-2024, 2024a. a, b, c, d, e, f, g, h, i, j, k, l, m, n, o
Givon, Y., Keller, D., Pennel, R., Drobinski, P., and Raveh-Rubin, S.: Decomposing the role of dry intrusions for ocean evaporation during mistral, Q. J. Roy. Meteorol. Soc., 150, 1791–1808, https://doi.org/10.1002/qj.4670, 2024b. a, b
Guan, B. and Waliser, D. E.: Detection of atmospheric rivers: Evaluation and application of an algorithm for global studies, J. Geophys. Res.-Atmos., 120, 12514–12535, 2015. a
Gutiérrez-Fernández, J., Miglietta, M. M., González-Alemán, J. J., and Gaertner, M. A.: A new refinement of Mediterranean tropical-like cyclones characteristics, Geophys. Res. Lett., 51, e2023GL106429, https://doi.org/10.1029/2023GL106429, 2024. a
Guzzetti, F., Peruccacci, S., Rossi, M., and Stark, C. P.: The rainfall intensity–duration control of shallow landslides and debris flows: an update, Landslides, 5, 3–17, 2008. a
Heitmann, K., Sprenger, M., Binder, H., Wernli, H., and Joos, H.: Warm conveyor belt characteristics and impacts along the life cycle of extratropical cyclones: case studies and climatological analysis based on ERA5, Weather Clim. Dynam., 5, 537–557, https://doi.org/10.5194/wcd-5-537-2024, 2024. a, b
Hénin, R., Ramos, A. M., Pinto, J. G., and Liberato, M. L.: A ranking of concurrent precipitation and wind events for the Iberian Peninsula, Int. J. Climatol., 41, 1421–1437, 2021. a
Hepworth, E., Messori, G., and Vichi, M.: Association between extreme atmospheric anomalies over Antarctic sea ice, Southern Ocean polar cyclones and atmospheric rivers, J. Geophys. Res.-Atmos., 127, e2021JD036121, https://doi.org/10.1029/2021JD036121, 2022. a
Hersbach, H., Bell, B., Berrisford, P., Hirahara, S., Horányi, A., Muñoz-Sabater, J., Nicolas, J., Peubey, C., Radu, R., Schepers, D., Simmons, A., Soci, C., Abdalla, S., Abellan, X., Balsamo, G., Bechtold, P., Biavati, G., Bidlot, J., Bonavita, M., De Chiara, G., Dahlgren, P., Dee, D., Diamantakis, M., Dragani, R., Flemming, J., Forbes, R., Fuentes, M., Geer, A., Haimberger, L., Healy, S., Hogan, R. J., Hólm, E., Janisková, M., Keeley, S., Laloyaux, P., Lopez, P., Lupu, C., Radnoti, G., de Rosnay, P., Rozum, I., Vamborg, F., Villaume, S., and Thépaut, J.-N.: The ERA5 global reanalysis, Q. J. Roy. Meteorol. Soc., 146, 1999–2049, 2020. a, b, c
Hersbach, H., Bell, B., Berrisford, P., Biavati, G., Horányi, A., Muñoz Sabater, J., Nicolas, J., Peubey, C., Radu, R., Rozum, I., Schepers, D., Simmons, A., Soci, C., Dee, D., Thépaut, J.-N.: ERA5 hourly data on single levels from 1940 to present, Copernicus Climate Change Service (C3S) Climate Data Store (CDS) [data set], https://doi.org/10.24381/cds.adbb2d47, 2023. a
Hewson, T. D.: Objective fronts, Meteorol. Appl., 5, 37–65, 1998. a
Hewson, T. D. and Neu, U.: Cyclones, windstorms and the IMILAST project, Tellus A, 67, 27128, https://doi.org/10.3402/tellusa.v67.2712810.3402/tellusa.v67.27128, 2015. a, b
Houze Jr., R. A.: Orographic effects on precipitating clouds, Rev. Geophys., 50, RG1001, https://doi.org/10.1029/2011RG000365, 2012. a, b
Impact Forecasting LLC: November 2011 Monthly Cat Recap, https://web.archive.org/web/20181202193349/http://thoughtleadership.aonbenfield.com/documents/201112_if_monthly_cat_recap_november.pdf (last access: 12 January 2024), 2011. a
Jansa, A., Genoves, A., Picornell, M. A., Campins, J., Riosalido, R., and Carretero, O.: Western Mediterranean cyclones and heavy rain. Part 2: Statistical approach, Meteorol. Appl., 8, 43–56, 2001. a, b, c, d
Kilsdonk, R. A., Bomers, A., and Wijnberg, K. M.: Predicting Urban Flooding Due to Extreme Precipitation Using a Long Short-Term Memory Neural Network, Hydrology, 9, 105, https://doi.org/10.3390/hydrology9060105, 2022. a
Klawa, M. and Ulbrich, U.: A model for the estimation of storm losses and the identification of severe winter storms in Germany, Nat. Hazards Earth Syst. Sci., 3, 725–732, https://doi.org/10.5194/nhess-3-725-2003, 2003. a
Komen, G. J., Cavaleri, L., Donelan, M., Hasselmann, K., Hasselmann, S., and Janssen, P.: Dynamics and Modelling of Ocean Waves, Cambridge University Press, https://doi.org/10.1017/CBO9780511628955, 1996. a, b
Lavers, D. A., Simmons, A., Vamborg, F., and Rodwell, M. J.: An evaluation of ERA5 precipitation for climate monitoring, Q. J. Roy. Meteorol. Soc., 148, 3152–3165, 2022. a
Lionello, P., Trigo, I. F., Gil, V., Liberato, M. L., Nissen, K. M., Pinto, J. G., Raible, C. C., Reale, M., Tanzarella, A., Trigo, R. M., Ulbrich, S., and Ulbrich, U.: Objective climatology of cyclones in the Mediterranean region: a consensus view among methods with different system identification and tracking criteria, Tellus A, 68, 29391, https://doi.org/10.3402/tellusa.v68.29391, 2016. a
Madonna, E., Wernli, H., Joos, H., and Martius, O.: Warm conveyor belts in the ERA-Interim dataset (1979–2010). Part I: Climatology and potential vorticity evolution, J. Climate, 27, 3–26, 2014. a, b
Manning, C., Kendon, E. J., Fowler, H. J., Catto, J. L., Chan, S. C., and Sansom, P. G.: Compound wind and rainfall extremes: Drivers and future changes over the UK and Ireland, Weather Clim. Extrem., 44, 100673, https://doi.org/10.1016/j.wace.2024.100673, 2024. a
Martius, O., Pfahl, S., and Chevalier, C.: A global quantification of compound precipitation and wind extremes, Geophys. Res. Lett., 43, 7709–7717, 2016. a, b
Minola, L., Zhang, F., Azorin-Molina, C., Pirooz, A. S., Flay, R., Hersbach, H., and Chen, D.: Near-surface mean and gust wind speeds in ERA5 across Sweden: towards an improved gust parametrization, Clim. Dynam., 55, 887–907, 2020. a
Nissen, K. M., Leckebusch, G. C., Pinto, J. G., Renggli, D., Ulbrich, S., and Ulbrich, U.: Cyclones causing wind storms in the Mediterranean: characteristics, trends and links to large-scale patterns, Nat. Hazards Earth Syst. Sci., 10, 1379–1391, https://doi.org/10.5194/nhess-10-1379-2010, 2010. a, b, c, d, e, f
Obermann-Hellhund, A.: State of the Simulation of Mesoscale Winds in the Mediterranean and Opportunities for Improvements, Atmosphere, 13, 1007, https://doi.org/10.3390/atmos13071007, 2022. a, b
Owen, L. E., Catto, J. L., Stephenson, D. B., and Dunstone, N. J.: Compound precipitation and wind extremes over Europe and their relationship to extratropical cyclones, Weather Clim. Extrem., 33, 100342, https://doi.org/10.1016/j.wace.2021.100342, 2021. a, b, c, d, e, f, g, h
Pacey, G., Pfahl, S., Schielicke, L., and Wapler, K.: The climatology and nature of warm-season convective cells in cold-frontal environments over Germany, Nat. Hazards Earth Syst. Sci., 23, 3703–3721, https://doi.org/10.5194/nhess-23-3703-2023, 2023. a
Papritz, L., Pfahl, S., Rudeva, I., Simmonds, I., Sodemann, H., and Wernli, H.: The role of extratropical cyclones and fronts for Southern Ocean freshwater fluxes, J. Climate, 27, 6205–6224, 2014. a, b
Pfahl, S.: Characterising the relationship between weather extremes in Europe and synoptic circulation features, Nat. Hazards Earth Syst. Sci., 14, 1461–1475, https://doi.org/10.5194/nhess-14-1461-2014, 2014. a, b, c, d, e, f, g, h, i, j, k
Pfahl, S. and Wernli, H.: Quantifying the relevance of cyclones for precipitation extremes, J. Climate, 25, 6770–6780, 2012. a, b, c, d
Pfahl, S., Madonna, E., Boettcher, M., Joos, H., and Wernli, H.: Warm conveyor belts in the ERA-Interim dataset (1979–2010). Part II: Moisture origin and relevance for precipitation, J. Climate, 27, 27–40, 2014. a, b
Ralph, F. M., Dettinger, M., Lavers, D., Gorodetskaya, I. V., Martin, A., Viale, M., White, A. B., Oakley, N., Rutz, J., Spackman, J. R., Wernli, H., and Cordeira, J.: Atmospheric rivers emerge as a global science and applications focus, B. Am. Meteorol. Soc., 98, 1969–1973, 2017. a
Raveh-Rubin, S.: Dry intrusions: Lagrangian climatology and dynamical impact on the planetary boundary layer, J. Climate, 30, 6661–6682, 2017. a, b, c
Raveh-Rubin, S. and Wernli, H.: Large-scale wind and precipitation extremes in the Mediterranean: a climatological analysis for 1979–2012, Q. J. Roy. Meteorol. Soc., 141, 2404–2417, 2015. a, b, c, d, e, f
Raveh-Rubin, S. and Wernli, H.: Large-scale wind and precipitation extremes in the Mediterranean: dynamical aspects of five selected cyclone events, Q. J. Roy. Meteorol. Soc., 142, 3097–3114, 2016. a, b, c, d
Reale, M., Cabos Narvaez, W. D., Cavicchia, L., Conte, D., Coppola, E., Flaounas, E., Giorgi, F., Gualdi, S., Hochman, A., Li, L., Lionello, P., Podrascanin, Z., Salon, S., Sanchez-Gomez, E., Scoccimarro, E., Sein, D. V., and Somot, S.: Future projections of Mediterranean cyclone characteristics using the Med-CORDEX ensemble of coupled regional climate system models, Clim. Dynam., 1–24, 2022. a
Ridder, N. N., Pitman, A. J., Westra, S., Ukkola, A., Do, H. X., Bador, M., Hirsch, A. L., Evans, J. P., Di Luca, A., and Zscheischler, J.: Global hotspots for the occurrence of compound events, Nat. Commun., 11, 5956, https://doi.org/10.1038/s41467-020-19639-3, 2020. a, b, c
Rivoire, P., Martius, O., and Naveau, P.: A comparison of moderate and extreme ERA-5 daily precipitation with two observational data sets, Earth Space Sci., 8, e2020EA001633, https://doi.org/10.1029/2020EA001633, 2021. a
Rousseau-Rizzi, R., Raveh-Rubin, S., Catto, J., Portal, A., Givon, Y., and Martius, O.: A storm-relative climatology of compound hazards in Mediterranean cyclones, EGUsphere [preprint], https://doi.org/10.5194/egusphere-2023-2322, 2023. a, b, c, d, e, f, g
Sansom, P. G. and Catto, J. L.: Improved objective identification of meteorological fronts: a case study with ERA-Interim, Geosci. Model Dev. Discuss. [preprint], https://doi.org/10.5194/gmd-2022-255, in review, 2022. a
Schultz, D. M.: Reexamining the cold conveyor belt, Mon. Weather Rev., 129, 2205–2225, 2001. a
Schultz, D. M., Bosart, L. F., Colle, B. A., Davies, H. C., Dearden, C., Keyser, D., Martius, O., Roebber, P. J., Steenburgh, W. J., Volkert, H., and Winters, A. C.: Extratropical cyclones: A century of research on meteorology's centerpiece, Meteorol. Monogr., 59, 16.1–16.56, 2019. a
Seneviratne, S. I., Nicholls, N., Easterling, D., Goodess, C. M., Kanae, S., Kossin, J., Luo, Y., Marengo, J., McInnes, K., Rahimi, M., Reichstein, M., Sorteberg, A., Vera, C., and Zhang, X.: Changes in Climate Extremes and their Impacts on the Natural Physical Environment, Cambridge University Press, 109–230, ISBN 9781139177245, 2012. a, b
Sprenger, M., Fragkoulidis, G., Binder, H., Croci-Maspoli, M., Graf, P., Grams, C. M., Knippertz, P., Madonna, E., Schemm, S., Škerlak, B., and Wernli, H.: Global climatologies of Eulerian and Lagrangian flow features based on ERA-Interim, B. Am. Meteorol. Soc., 98, 1739–1748, 2017. a
The Washington Post: Mediterranean storm brings heavy rain and flooding to Italy and France, https://www.washingtonpost.com/blogs/capital-weather-gang/post/mediterranean-storm-brings-heavy-rain-and-flooding-to-italy (last access: 9 April 2024), 2011. a
Trigo, I. F.: Climatology and interannual variability of storm-tracks in the Euro-Atlantic sector: a comparison between ERA-40 and NCEP/NCAR reanalyses, Clim. Dynam., 26, 127–143, 2006. a
Trigo, I. F., Davies, T. D., and Bigg, G. R.: Objective climatology of cyclones in the Mediterranean region, J. Climate, 12, 1685–1696, 1999. a
Utsumi, N., Kim, H., Kanae, S., and Oki, T.: Which weather systems are projected to cause future changes in mean and extreme precipitation in CMIP5 simulations?, J. Geophys. Res.-Atmos., 121, 10–522, 2016. a
Waliser, D. and Guan, B.: Extreme winds and precipitation during landfall of atmospheric rivers, Nat. Geosci., 10, 179–183, 2017. a
Wernli, H.: A Lagrangian-based analysis of extratropical cyclones. II: A detailed case-study, Q. J. Roy. Meteorol. Soc., 123, 1677–1706, 1997. a
Wernli, H. and Davies, H. C.: A Lagrangian-based analysis of extratropical cyclones. I: The method and some applications, Q. J. Roy. Meteorol. Soc., 123, 467–489, 1997. a, b
Wilks, D. S.: Statistical methods in the atmospheric sciences, in: vol. 100, Academic Press, https://doi.org/10.1016/C2017-0-03921-6, 2011. a
Zhang, Z. and Li, X.-M.: Global ship accidents and ocean swell-related sea states, Nat. Hazards Earth Syst. Sci., 17, 2041–2051, https://doi.org/10.5194/nhess-17-2041-2017, 2017. a
Ziv, B., Saaroni, H., Romem, M., Heifetz, E., Harnik, N., and Baharad, A.: Analysis of conveyor belts in winter Mediterranean cyclones, Theor. Appl. Climatol., 99, 441–455, 2010. a
Zscheischler, J., Westra, S., Van Den Hurk, B. J., Seneviratne, S. I., Ward, P. J., Pitman, A., AghaKouchak, A., Bresch, D. N., Leonard, M., Wahl, T., and Zhang, X.: Future climate risk from compound events, Nat. Clim. Change, 8, 469–477, 2018. a
Zscheischler, J., Martius, O., Westra, S., Bevacqua, E., Raymond, C., Horton, R. M., van den Hurk, B., AghaKouchak, A., Jézéquel, A., Mahecha, M. D., and Maraun, D.: A typology of compound weather and climate events, Nat. Rev. Eearth Environ., 1, 333–347, 2020. a
The term rain is here generalised to indicate precipitation.
The figure label S refers to the Supplement document.
See website at https://medcyclones.eu/ (last access: 29 July 2024).
The original DI dataset is smoothed using 2D convolution on a four-by-four symmetric kernel in order to connect fragmented masks into single DI objects.
Regions of unit-valued grid points connected via a structuring element ([111][111][111]), which allows for diagonal connections.
Maps available at https://boris.unibe.ch/id/eprint/192315 (last access: 29 July 2024).
A fixed-radius area is here preferred to an SLP-based dynamical area because of Mediterranean orography inducing uncertainties in the SLP field (Flaounas et al., 2015).
Average over the Mediterranean region within 28–50° N, 10° W–45° E, excluding white masked areas.
See footnote 8.
The word “isolated” is used to indicate features that do not fulfil the criteria for joining a Mediterranean cyclone's impact area, as described in Sect. 3.
See footnote 8.
- Abstract
- Introduction
- Data and methods
- An impact area for Mediterranean cyclones
- Frequencies of compound extremes and Mediterranean cyclones
- Discussion
- Conclusions
- Appendix A: Discussion on the choice of impact area
- Code availability
- Data availability
- Author contributions
- Competing interests
- Disclaimer
- Acknowledgements
- Financial support
- Review statement
- References
- Supplement
- Abstract
- Introduction
- Data and methods
- An impact area for Mediterranean cyclones
- Frequencies of compound extremes and Mediterranean cyclones
- Discussion
- Conclusions
- Appendix A: Discussion on the choice of impact area
- Code availability
- Data availability
- Author contributions
- Competing interests
- Disclaimer
- Acknowledgements
- Financial support
- Review statement
- References
- Supplement