the Creative Commons Attribution 4.0 License.
the Creative Commons Attribution 4.0 License.
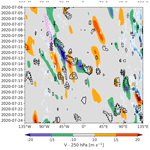
Investigation of links between dynamical scenarios and particularly high impact of Aeolus on numerical weather prediction (NWP) forecasts
Anne Martin
Martin Weissmann
Alexander Cress
Global wind profiles from the Aeolus satellite mission provide an important source of wind information for numerical weather prediction (NWP). Data assimilation experiments show large mean changes in the analysis and a significant reduction in forecast errors. At Deutscher Wetterdienst (DWD), a 3-month observing system experiment (OSE), from July 2020 to October 2020, was performed to evaluate the impact of the Aeolus horizontal line-of-sight (HLOS) wind observations in the operational data assimilation system of the ICOsahedral Nonhydrostatic (ICON) global model. To better understand the underlying dynamics leading to the overall beneficial impact, specific time periods and regions with a particularly high impact of Aeolus are investigated. In this study, we illustrate three examples of atmospheric phenomena that constitute dynamical scenarios for significant forecast error reduction through the assimilation of Aeolus: the phase shift of large-scale tropical circulation systems, namely the Quasi-Biennial Oscillation (QBO) and the El Niño–Southern Oscillation (ENSO), and the interaction of tropical cyclones undergoing extratropical transition (ET) with the midlatitude waveguide.
- Article
(14726 KB) - Full-text XML
- BibTeX
- EndNote
The Aeolus satellite mission from the European Space Agency (ESA) provides a novel dataset of actively sensed wind profiles with quasi-global coverage intended to compensate for deficiencies of the current Global Observing System (GOS). Besides other scientific and technological developments, advances in the operational assimilation of satellite observations have been one of the major contributing factors to the increase in numerical weather prediction (NWP) skill over the last decade (Bauer et al., 2015). However, the information available from satellite instruments is not always directly related to the fundamental variables of the model, and the Global Observing System is heavily biased towards mass observations (Baker et al., 2014). This can be a strong limitation in regions and for spatial scales where the geostrophic mass–wind coupling is weak and the atmospheric dynamics are mainly determined by the wind field. Therefore, direct wind profile information from the Aeolus satellite is expected to be particularly efficient in NWP for the understanding of tropical dynamics on all length scales and the prediction of smaller-scale phenomena at higher latitudes (ESA, 2008). Furthermore, the ESA Mission Advisory Group highlighted potential benefits from the Aeolus wind observations for improvements in the characterization of severe and intense storm developments and scale interaction processes associated with atmospheric wave activity (ESA, 1999, 2008, 2016).
The Aeolus satellite carries a Doppler wind lidar (DWL) instrument that can receive the backscatter signal from molecules (Rayleigh channel) and particles (Mie channel) up to a maximum measurement altitude of about 17–25 km. The wind product suitable for the use in NWP is the horizontal projection of the horizontal line-of-sight (HLOS) wind component. In parallel with the technical development of the Aeolus mission, several scientific and campaign activities were carried out to evaluate the potential of the HLOS observations for NWP. Adding simulated ADM-Aeolus-like (Atmospheric Dynamics Mission) lidar observations to the GOS has been found to reduce forecast errors for poorly predicted severe weather events (Marseille et al., 2008a, b), in the 500 hPa average medium-range wind forecast over the Northern Hemisphere (Stoffelen et al., 2006), for the analysis and forecasts over oceans (Tan et al., 2007) and for tropical wave dynamics (Žagar, 2004; Žagar et al., 2008). Furthermore, DWL instruments were used on research flights during measurement campaigns over the North Atlantic (A-TReC; Atlantic THORPEX (The Observing System Research and Predictability Experiment) Regional Campaign) and the Asian Pacific Ocean (T-PARC; THORPEX Pacific Asian Regional Campaign). Their assimilation into global NWP models led to a significant impact on the analysis and forecasts over Europe and around the development of tropical cyclones, as well as their interaction with the midlatitude waveguide (Pu et al., 2010; Weissmann and Cardinali, 2007; Weissmann et al., 2012). Since the launch of the Aeolus satellite in August 2018, several observing system experiment (OSE) studies have been conducted to investigate the impact of the Rayleigh and Mie HLOS wind observations in various NWP models (Garrett et al., 2022; Laroche and St-James, 2022; Pourret et al., 2022; Rennie et al., 2021). Most of these studies concentrated on global forecast error statistics showing large overall improvements. A particularly high impact of assimilating Aeolus observations was found for the 2–3 d wind and temperature forecasts in the tropical upper troposphere and lower stratosphere and in the Southern Hemisphere. In the Northern Hemisphere, Aeolus HLOS winds were found to have a less pronounced but still a relatively large influence on average compared to other observing systems.
Martin et al. (2022) performed an OSE for 3 months, from July 2020 to October 2020, using the global ICOsahedral Nonhydrostatic (ICON) model from DWD. The study introduced the experimental setup and the data quality and consistency and provided an overview of the systematic changes in the analysis and impact on forecast error. The results overall confirm the promising statistical improvements demonstrated in the global impact studies of Garrett et al. (2022), Laroche and St-James (2022), Pourret et al. (2022), and Rennie et al. (2021).
Using the OSE from Martin et al. (2022), this study aims to further investigate specific dynamical changes and processes related to the positive impact of Aeolus observations and to document dynamical scenarios that lead to particularly high forecast error reduction. In the tropics, 2 weeks of large improvement in the eastern Pacific west of the Peruvian coast and about 5 weeks in the global equatorial band in the stratosphere particularly stand out. These regions and periods are associated with a change in phase of the large-scale tropical circulation systems, the El Niño–Southern Oscillation (ENSO) and the Quasi-Biennial Oscillation (QBO). In addition, we focus on the midlatitudes, where spatiotemporal surveys show large forecast error reduction following the extratropical transition (ET) of tropical cyclones and their interaction with the midlatitude waveguide.
The outline of this paper is as follows: details about the Aeolus observations and the experimental design, including the quality control criteria and verification methods, are described in Sect. 2. Section 3 provides a global overview of the Aeolus impact in the ICON model and provides motivation for the further investigation of selected regions and periods. Section 4 examines the relation of the impact of Aeolus to specific atmospheric features such as the variability in the stratospheric jet (QBO), the El Niño–Southern Oscillation (ENSO) state change in the equatorial eastern Pacific and the extratropical transition (ET) of tropical cyclones in association with synoptic-scale Rossby wave packets (RWPs). Finally, Sect. 5 summarizes the study's main conclusions.
2.1 Aeolus HLOS observations
The Doppler wind lidar (DWL) on board the Aeolus satellite consists of a dual-channel receiver analyzing the Doppler shift from the narrowband Mie and the broadband Rayleigh backscatter separately. The signals are detected and binned into 24 range bins that can be varied along orbit from a minimum vertical resolution of 250 to 2000 m and thus be adjusted to the needs of science applications and NWP. Typically, the uppermost measurement altitude is about 17–25 km. Horizontally, a minimum along-track resolution of 2.9 km (temporal resolution of 0.4 s) is achieved by accumulating 20 laser pulses and referred to as one “measurement”. The operational on-ground processing of the different Aeolus products is performed in near real time (NRT) at the European Centre for Medium-Range Weather Forecasts (ECMWF). The Aeolus Level-2B (L2B) wind product contains the HLOS wind observations used for NWP. To control the horizontal resolution and achieve a sufficient signal-to-noise ratio, the measurements are grouped according to a scene-classification procedure into a “clear” or “cloudy” type (Tan et al., 2008). Therefore, measurement-scale optical properties, e.g., scattering ratio or particle extinction coefficient, are used to determine how much particulate and molecular backscatter contributes to the signal of an accumulated measurement bin. Four wind observation types are available: Rayleigh clear and Rayleigh cloudy with a horizontal average length of about 90 km and Mie clear and Mie cloudy, representing a horizontal mean of 10 km. A key step within the L2B processing chain is the correction for temperature and pressure-dependent Doppler broadening in the molecular backscatter signal (Rayleigh–Brillouin correction). This uses a series of auxiliary files containing information about, e.g., geolocation, calibration and error estimates for several variables, as well as a priori (AUX_MET) data of atmospheric temperature and pressure from a short-range forecast (Šavli et al., 2021). The L2B processor provides several additional output data, such as uncertainty estimates or quality flags for the wind observations, which are useful for data assimilation systems. The Aeolus data processing of the OSE period includes an NRT bias correction method. During the first part of the mission, validation studies showed large systematic differences, which vary seasonally, spatially and with orbital phase and are particularly pronounced for the Rayleigh wind observations (e.g., A. Martin et al., 2021; Rennie et al., 2021). These detected bias dependencies were found to be related to longwave and solar radiation fluctuations and the radiative response to which the spectrometers of the DWL are very sensitive. The operationally implemented bias correction is based on a multiple linear regression method of ECMWF observation minus background (O − B) statistics and the thermistors of the telescope M1 mirror, eliminating most parts of the bias (Weiler et al., 2021).
2.2 Model and experimental design
This section provides a brief description of the model and the experimental setup. For further information please refer to Martin et al. (2022). In this study, the impact of the Aeolus L2B HLOS wind observations is assessed using an experiment with the operational version of the ICON model at DWD that is based on the nonhydrostatic system of equations in the global domain (Zängl et al., 2015). In the current operational version, the atmosphere is resolved by an icosahedral grid with a 13 km horizontal mesh size and 90 layers in the vertical direction. The core module of the global data assimilation system is a hybrid variational ensemble Kalman filter (VarEnKF) combining the flow-dependent background error covariance matrix from a local ensemble transform Kalman filter (LETKF) with the static covariance matrix from the three-dimensional variational (3D-Var) data assimilation system (Reinert et al., 2023). This combination allows for the inclusion of the time-varying background error structures and thus a better weighting of observations and background. The LETKF is based on a 40-member ensemble with a horizontal resolution of 40 km. The assimilation is carried out with 3 h cycling (00:00, 03:00, …, 18:00, 21:00 UTC). A 3 h short-term forecast serves as a background field that is adjusted using all observations within ±1.5 h around the corresponding time step to generate the analysis field from which the next forecast is initialized.
The impact of an existing observation network in an NWP model can be estimated by conducting OSEs. In an OSE, two continuous assimilation cycles are performed: a control run which typically uses the operational model and observation dataset and an experimental run in which the observation type of interest is either added or removed. Comparisons of the resulting analyses and corresponding forecasts then serve as the basis for impact studies. As described in Martin et al. (2022), the control run (CTRL) was performed without Aeolus but with all other operationally used observation types assimilated. The observations assimilated operationally are mainly radiances that account for ∼ 64 % of the total observations. Winds from scatterometers, satellite imagery and global navigation satellite system (GNSS) signals together constitute about 18 %. Conventional observations from aircraft reports, radiosondes, surface stations, buoys, pilots and wind profilers represent ∼ 7 % of the total number of observations. The proportion of assimilated wind profiles from the spaceborne lidar of the Aeolus mission is about 2 % (∼ 20 500 HLOS wind observations per assimilation cycle). In the experimental run (EXP_A), the Rayleigh and Mie HLOS wind observations have been assimilated in addition to all other observation types. Both assimilation experiments were conducted with a corresponding cycled LETKF ensemble run to provide individual background error covariance estimates for the experiments. For EXP_A, the model background winds u and v have been interpolated to the Aeolus observation geolocation point (latitude, longitude and height) and transformed to the Aeolus HLOS wind equivalents using the azimuth angle ϕ, which is defined as the angle of the LOS pointing vector of the laser projected onto the horizontal plane measured clockwise from north:
ϕ is provided as part of the observation geolocation information by the L2B product, typical being ∼ 260∘ for ascending and ∼ 100∘ for descending orbits. Assuming the HLOS winds as point observations with neglected vertical velocity is a reasonable approach since the averaging length scale of the Rayleigh HLOS winds is approximately the same size as the effective model resolution of ICON (between 80 and 100 km in the horizontal direction and between a few hundred meters in the lower troposphere up to 2 km in the stratosphere). The Mie winds' averaging box is about a factor of 10 smaller, but no thinning is applied. The assigned observation error for EXP_A was derived based on the Desroziers method (Desroziers et al., 2005) and provided in a table-driven format (Martin et al., 2022). It is the smallest in the middle troposphere and largest in the lower troposphere and the stratospheric levels.
2.2.1 Quality control settings for the OSE
To optimize the use of the L2B wind observations in the ICON model, the following quality control criteria were applied before the assimilation:
-
Only observations with valid overall confidence flags have been used.
-
Only the observation types Rayleigh clear and Mie cloudy were used, as they are generally of better quality than the other two observation types.
-
Rayleigh winds with range bin thicknesses of 250 m were rejected because of excessive noise.
-
Rayleigh winds with a horizontal accumulation length < 60 km and Mie winds with horizontal accumulation length < 5 km were rejected due to a large number of outliers in observation departure statistics.
-
Rayleigh winds with an L2B processor-estimated observation error > 8 m s−1 were excluded.
-
Mie winds with an L2B processor-estimated observation error > 6 m s−1 were excluded.
These quality control criteria were found to be reasonable in a preceding validation study (A. Martin et al., 2021). The OSE for this study covers the Northern Hemisphere summer of July–October 2020. Although the operationally implemented telescope primary-mirror M1 bias correction is very effective, the DWD system still shows a small residual bias that depends on altitude for the Rayleigh wind observations. Therefore, a model-based bias correction scheme has been applied to the Rayleigh and Mie wind observations. The bias correction is a function of latitude and conducted for specific pressure levels: surface–850, 850–500, 500–200, 200–70 and 70–5 hPa. The bias correction values are derived from the previous 7 d and separately for ascending and descending orbits. More details can be found in Martin et al. (2022).
2.2.2 Verification data and method
In general, forecast errors are defined as the differences between the forecast provided by a NWP model and the true atmospheric state. To assess the systematic impact of the Aeolus HLOS wind observations in the OSE, we compare the forecast errors in the CTRL and EXP_A experiment. Therefore, ERA5 reanalyses are used as the verification dataset. The ERA5 output is produced using the 4D-Var data assimilation of the ECMWF Integrated Forecasting System (IFS) at a horizontal resolution of 31 km and with 137 vertical model levels up to the height of 80 km (from 1000 to 1 hPa, with 40 levels below 5 km) (Hersbach et al., 2020). As ERA5 reanalyses are based on a different model with a different resolution compared to the OSE, they provide a relatively independent data source. The higher vertical resolution of ERA5 allows for finer details of atmospheric phenomena to be resolved, such as a more realistic representation of atmospheric waves and their interaction with the mean flow, which is especially crucial for the study of QBO in Sect. 4.1. Furthermore, ERA5 assimilates a partly different set of observations than the global data assimilation system in the ICON model (e.g., more satellite radiances) and does not use the Aeolus observations. It is well known that NWP models in the stratosphere are typically subject to large uncertainties. ERA5 was found to have a cold bias in the lower stratosphere and a warm bias near the stratopause (Hersbach et al., 2020). However, the increased number of assimilated GPSRO (GPS radio occultation) bending angles in ERA5 since 2006 has significantly reduced this model bias, increasing confidence in using the stratospheric reanalyses for verification (Laloyaux et al., 2020).
The methods applied in this study are described according to Martin et al. (2022). The forecast error in an assimilation experiment X is calculated as
with X being either the CTRL or the EXP_A run and i representing the time step when the forecast and analysis are valid, respectively. The root-mean-squared error (RMSE) of the experiment X determines how strongly the forecast deviates from the verification data:
where the overline is the mean over the requested dimension/dimensions – time, pressure level, latitude or longitude. Improvement or degradation of the forecast quality through the assimilation of Aeolus observations can then be assessed by the differences between RMSE(eEXP_A) and RMSE(eCTRL). Because the RMSE depends on the magnitude of forecasts and observations, the results are additionally verified by calculating the normalized RMSE differences:
The selection of particular regions of pronounced forecast error reduction due to the Aeolus observations, which are examined in the subsequent section (Sect. 4), is motivated by Fig. 1 (according to Martin et al., 2022).
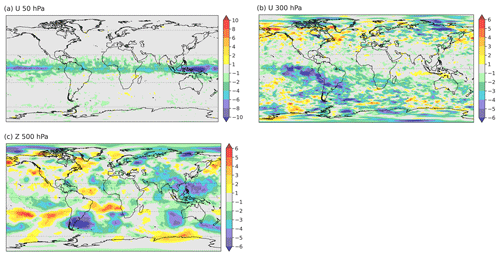
Figure 1The mean relative differences in 24–120 h forecast RMSE between the EXP_A and the CTRL run (%) for 1 July to 30 September 2020: (a) 50 hPa zonal-wind component (U), (b) 300 hPa zonal-wind component (U) and (c) 500 hPa geopotential (Z).
Table 1Mean relative difference in 24–120 h forecast RMSE between the EXP_A and the CTRL run (%) averaged over the polar, midlatitude and tropical region in the Northern Hemisphere and Southern Hemisphere for the 50 hPa zonal-wind component (U), the 300 hPa zonal-wind component (U) and 500 hPa geopotential (Z).

Results of the OSE impact statistics evaluated by Martin et al. (2022) show the largest reduction in the zonal-wind RMSE in the upper troposphere and lower stratosphere. Within the tropical band, the forecast errors in forecast lead times from 24 to 120 h are reduced by 2 % up to almost 5 % on average (Table 1). The spatial distribution of the relative RMSE reduction (Fig. 1a) reveals that above the tropical tropopause, the beneficial impact is primarily located around the equatorial band. On large scales, this area is affected by the Quasi-Biennial Oscillation of the zonal wind (QBO). Typically, the equatorial zonal wind between 70 and 10 hPa alternates between easterlies and westerlies, with the particular phase of the oscillation propagating downward over a period of 22 to 34 months. During the OSE period in summer 2020, the QBO cycling evolved as a westerly jet between 50 and 30 hPa, which was found to be largely strengthened by the Aeolus observation (Martin et al., 2022). In Sect. 4.1, we evaluate the hypothesis that there is a relation between the QBO phase change and the high impact of Aeolus.
Furthermore, large forecast error reduction can be found in the 300 hPa zonal wind in the midlatitudes in the Southern Hemisphere and the tropics with an average improvement of 1.7 %–2.6 %. The midlatitudes in the Southern Hemisphere overall show a fluctuating impact pattern. However, the most pronounced forecast error reduction appears around the storm track region downstream of South America. In the tropics, a striking forecast error reduction is located around the eastern Pacific Ocean and the subtropical jet over South America at 300 hPa. The large-scale dynamics there are mainly dominated by the coupled circulation system ENSO, which is characterized by the interaction between surface temperatures and upper-level winds. Large systematic changes in the analysis over the eastern Pacific due to the assimilation of Aeolus observations in the OSE were found by Martin et al. (2022) in the form of a strengthening of easterly winds. The OSE covers the onset of a shift in ENSO conditions which is generally associated with modifications in the zonal Walker circulation. This change in the wind pattern is expected to be the dynamical source of the large Aeolus impact and is discussed in more detail in the following section (Sect. 4.2).
Further north, at 500 hPa geopotential, large forecast error reduction occurs near the Tropic of Cancer (∼ 23∘ N) in the western Pacific and Atlantic. These are regions of tropical cyclone activity in the experimental period. Furthermore, large improvement can be seen in the Southern Hemisphere storm track areas, such as the Indian Ocean, the eastern side of Australia and the region near 30∘ S over South America. On average, forecast errors are reduced by 1.9 % in the tropics and by 1.7 % (0.7 %) in the Southern Hemisphere (Northern Hemisphere) midlatitudes. Overall, the mean impact pattern in the Northern Hemisphere is characterized by large variability likely related to fluctuations in the polar jet and the associated midlatitude circulation that partially obscures the mean error reduction. Large forecast error reduction over continents occurs over the Himalayan region, India and eastern Asia. Moreover, both the zonal wind at 300 hPa and geopotential at 500 hPa show large improvements of 1.4 %–1.6 % in the polar region in the Northern Hemisphere. Aeolus's impact in these regions may be investigated further in future investigations. In this study, we further examine the spatiotemporal evolution of forecast error reduction in midlatitudes to better understand the impact of Aeolus in relation to the general midlatitude circulation and to identify associated specific extratropical weather systems with a particular focus on the ET of tropical cyclones (Sect. 4.3).
4.1 Impact on tropical stratospheric wind variations (QBO)
Systematic changes in the analysis field (Martin et al., 2022) and improvements in forecast quality of the stratospheric zonal-wind field (Fig. 1) indicate an impact of the Aeolus observations on the QBO phase change from easterly to westerly that took place in summer 2020. In order to better monitor the QBO in 2020, the vertical range bin setting of Aeolus was adjusted to also allow for measurements up to 25 km in the tropics. This advanced setting was active in the tropical belt ±10∘ once a week for 24 h only, from Wednesday at 01:00 UTC until Thursday at 01:15 UTC. After an unusual disruption of QBO cycling in winter 2019/20 (Anstey et al., 2021), the regular oscillation in that stratospheric layer emerged again as an eastward jet around 20 August. Figure 2 focuses on the impact of Aeolus HLOS observation between 30 and 50 hPa around the Equator, showing the time evolution. The Aeolus observations affect the change in zonal-mean wind from easterly to westerly in the way that the evolved westerly winds are strengthened. The forecast errors in the CTRL run are fairly constant in time, only slightly varying between 4 and 6 m s−1. On the other hand, the forecast errors in EXP_A noticeably decrease with time. The relative differences in RMSE between EXP_A and CTRL already show improvements in the quality of the zonal-wind forecast between 30 and 50 hPa by the Aeolus observations at the beginning of the OSE period. The reversal of the zonal-wind direction is then accompanied by a marked reduction in the RMSE for all lead times. Towards the end of September, improvements of 10 % to over 15 % occur.
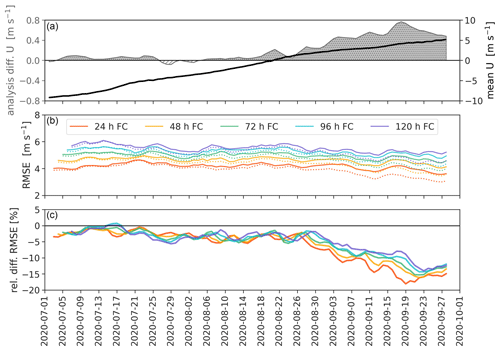
Figure 2Time series for the tropical belt ±10∘ latitude between 30 and 50 hPa. (a) The mean zonal wind (black line) and the mean analysis differences in the zonal wind between EXP_A and CTRL (shaded grey). (b) The RMSE for forecast (FC) lead times from 24 to 120 h for the CTRL (solid line) and the EXP_A (dotted line) run. (c) Relative differences in RMSE between EXP_A and CTRL for forecast lead times from 24 to 120 h.
It should be taken into account that both the ERA5 reanalysis used for verification and the global model ICON exhibit large uncertainties in the tropical stratosphere, probably contributing to the pronounced impact of Aeolus observations. The QBO is mainly driven by a combination of upward-propagating low-frequency equatorial waves and inertia–gravity waves from the troposphere that dissipate and deposit momentum to the upper-level zonal-mean zonal winds (Shepherd et al., 2018). However, a realistic representation of the wave–mean flow interaction behind the QBO is typically limited by insufficient vertical model resolution, uncertainties in parameterized processes such as tropical convection and the sparseness of direct wind measurements in the tropics. Given the lack of direct wind observations in the area, the models there could be prone to biases.
Due to QBO teleconnections with other parts of the atmospheric system such as the polar vortex (Anstey and Shepherd, 2014; Baldwin et al., 2001; Gray et al., 2018) or tropical oscillations such as, e.g., the Madden–Julian Oscillation (MJO) (Z. Martin et al., 2021) and ENSO (Anstey et al., 2021), accurate prediction of the QBO by an additional observing system might also provide a meaningful source of longer-term predictive skill outside the tropics. Furthermore, several statistical studies showed that besides or in interaction with the ENSO, the QBO could also modulate the tropical cyclone activities over various oceans (Baldwin et al., 2001; Gray, 1984; Gray et al., 1992; Jury et al., 1999) and that the QBO western phase is usually associated with enhanced deep convection on both sides of the Equator.
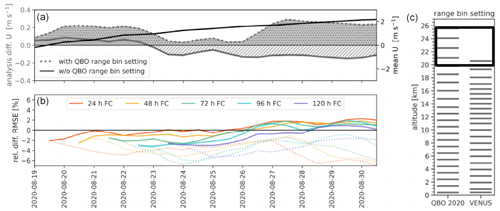
Figure 3Time series for the tropical belt ±10∘ latitude between 30 and 50 hPa. (a) The mean zonal wind (black line) and the mean analysis differences in the zonal wind between EXP_A and CTRL (shaded grey), with (dotted line) and without (solid line) the QBO range bin setting. (b) Relative differences in RMSE between EXP_A and CTRL for forecast lead times from 24 to 120 h, with (dotted line) and without (solid line) the QBO range bin setting. The distribution of the general tropical range bins (VENUS) and the advanced QBO setting is illustrated in panel (c), with the black rectangle highlighting the 30–50 hPa level.
Both accurate Aeolus wind measurements in the stratosphere and a good capture of upward-propagating waves from the lower levels can cause the strong positive impact on the QBO phase change. To exclude the influence of the extended special QBO range bin setting, which amplifies the effect of the stratospheric Aeolus observations, another experimental run, with a shorter period of 14 d, was performed. For this, the Aeolus observations between Wednesday at 01:00 UTC and Thursday at 01:15 UTC were not used in the assimilation. Figure 3 displays the relevant time series from 18 to 31 August 2020 of the analysis differences and the relative differences in RMSE for the tropical belt ±10∘ latitude between 30 and 50 hPa. The experiment with and without the QBO range bin setting are compared. On the right, in Fig. 3 the distribution of the general tropical range bin setting VENUS (VErtical momeNtum flUxeS) and the advanced QBO setting is illustrated. The experiment's start covers the time when the QBO western phase manifested again. Whereas the Aeolus experiment with the QBO range bin setting shows a large influence on the analysis in the form of an intensification of the western winds, the exclusion of the high-resolution stratospheric Aeolus observations leads to a weakening of the western jet after a few days. Striking differences also appear in terms of forecast error for lead times from 24 to 120 h. Initially, both experiments show beneficial effects in the equatorial stratosphere, but with time the forecast error reduction in the experiment without the QBO range bin setting decreases up to degradation of 2 %. As the stratosphere is characterized by large model uncertainties, a longer OSE would be useful to draw robust conclusions. However, it is noteworthy that even within 14 d, the elimination of observations during 2 d of higher-resolution Aeolus observations can have significant effects. This underscores the importance of the range bin settings for the Aeolus mission.
4.2 Impact on change in the ENSO state in the eastern Pacific
In the equatorial region, the ENSO is another important tropical oscillation pattern whose interannual tropospheric variability influences both weather and climate on a global scale. It is characterized by irregular periodic fluctuations through a neutral phase between warm (El Niño) and cold (La Niña) extremes in sea surface temperature (SST) across the equatorial Pacific Ocean. In summer 2020, the state of the ENSO changed from neutral to the La Niña state. The ENSO phases relate to the zonal Walker circulation that is strengthened during La Niña events as the eastern Pacific is colder and the western Pacific is warmer than on average, leading to an enhanced rise in warm moist air over Indonesia and South America and an enhanced downward branch over the mid-Pacific. Figure 4 displays the time evolution of relative differences in the 48 h forecast RMSE of the zonal-wind component averaged over the equatorial eastern Pacific (5∘ S–5∘ N, 90–160∘ W) as a function of altitude. The shift in the ENSO conditions is associated with a major change in the wind patterns of the tropical belt that appear to be strongly influenced by the assimilation of Aeolus wind observations. The 3-month average equatorial Pacific SST anomaly (Oceanic Niño Index, ONI) is a common measure and NOAA's primary indicator for monitoring the state of the ENSO. Around 8 August 2020, the SST anomaly, determined as the difference from the average ERA5 reanalysis of 1985 to 2015, exceeds the −0.5 K threshold, indicating the presence of La Niña conditions. This point in time is the beginning of a large forecast error reduction for upper-level zonal wind. The reduction in forecast error increases over the 14 d after the La Niña onset and extends into the middle troposphere. Enhanced improvements continue to occur afterwards and are also apparent for a shorter period before the onset. The largest error reduction in the 48 h forecast occurs about 48 h after the strongest negative increase in the SST anomaly, which corresponds to the time of initialization.
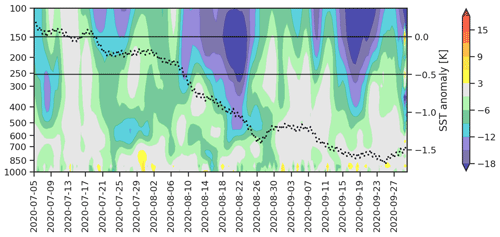
Figure 4Relative differences in 48 h forecast RMSE of the zonal-wind component (%) between EXP_A and CTRL (5∘ S–5∘ N, 90–160∘ W) as function of forecast time and pressure (hPa). The black dotted line is the sea surface temperature (SST) anomaly from the ERA5 reanalysis of 1985 to 2015.
Together with the results of Sect. 4.1, this suggests that Aeolus particularly reduces uncertainty in the model representation in the beginning of variations in the large-scale circulation systems. The better representation of the ENSO pattern as provided by the HLOS winds in the ICON model is expected to have a variety of further beneficial impacts. The fluctuations in the ENSO pattern can, for example, greatly affect the location of tropical rainfall and wind patterns. Moreover, influences on the extratropics are possible via the interaction with Rossby wave trains (Hoskins and Karoly, 1981), the North Atlantic Oscillation (NAO) phase (Rogers, 1984) or the Pacific–North American (PNA) pattern (Horel and Wallace, 1981), as well as through planetary wave activity into the stratosphere (Iza et al., 2016).
4.3 Dynamical impact in the midlatitudes
Hovmöller diagrams are a common way of plotting data in meteorology to display both the change over time and the spatial variability of a variable. In particular, they serve to highlight the behavior of atmospheric waves. To identify dynamical sources of the extratropical influence of Aeolus in the Northern Hemisphere, Fig. 5 represents the latitudinal average between 25 and 60∘ N of the 250 hPa meridional wind field and the convective precipitation as a function of longitude and time from 8 to 19 July 2020. The dashed black contour lines display the largest error reductions in the 48 h forecast of 500 hPa geopotential. At the beginning of the time period shown here, Cyclone Fay, which originally formed from a surface low over the northern Gulf of Mexico, emerged into the western Atlantic Ocean. The storm intensified while moving northward, reaching its peak intensity on 10 July. Later that day, Cyclone Fay made landfall over New Jersey and interacted with the midlatitude upper-level flow. The Hovmöller diagram shows pairs of green–blue and orange–red patches that form a clear banded pattern from 70–80∘ W around 10 July all the way to Europe on 15 July, representing individual troughs and ridges of an RWP. The contour lines related to the reduction in the 48 h forecast error in the 500 hPa geopotential are associated with this wave structure.
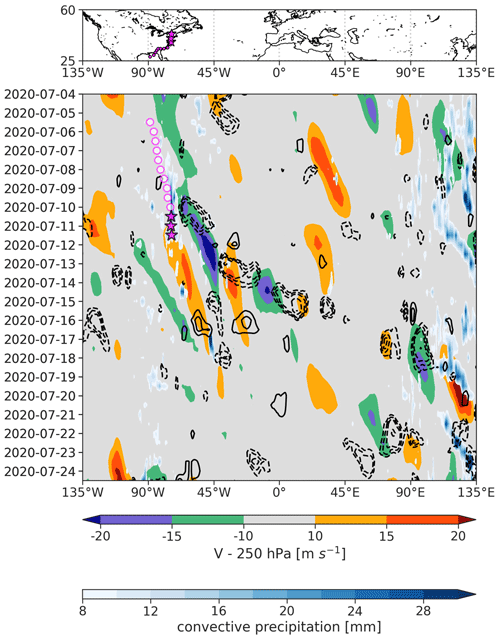
Figure 5Hovmöller diagram of the 250 hPa meridional wind (color shading, in m s−1) for 4 to 24 July 2020 and the convective precipitation averaged between 25 and 60∘ N. The black contours are the largest differences in the 48 h forecast error in 500 hPa geopotential between EXP_A and CTRL (dashed: negative, solid: positive). The magenta circles mark the position of Cyclone Fay; the stars highlight the onset of the ET and interaction with the midlatitude flow.
ET typically involves a complex interaction with the midlatitude baroclinic environment, which causes considerable changes in the characteristics of the cyclone (Grams et al., 2013). Interactions with the midlatitude waveguide can lead to increased forecast uncertainty, mainly associated with upper-level divergence, vertical wind shear and cirrus clouds (Jones et al., 2003). We, therefore, expect potential for a beneficial impact from good Mie wind coverage with a comparably high resolution. In general, there is no commonly accepted definition of ET, but various classification factors have been proposed for a typical ET event. In Jones et al. (2003), a definition of a two-stage classification of ET based on Klein et al. (2000) can be found. Typically, when the cyclone is affected by vertical wind shear associated with the baroclinic zone, the axisymmetric structure of the tropical cyclone around the core is distorted, resulting in an asymmetry in the wind and thermal structures and consequently in the moisture, cloud and precipitation fields. The upper-tropospheric divergent outflow, which appears as a cirrus cloud shield, may directly impact the large-scale midlatitude flow by interacting with the upstream trough of the midlatitude jet. Due to the tropical origin, low potential vorticity (PV) is advected by the divergent flow, which leads to a strengthening of the meridional PV gradient. This results in an amplification of the jet streak and the development of a ridge–trough couplet downstream of the transitioning cyclone, which marks the generation of a new RWP or the modification of an existing one (Wirth et al., 2018). The RWP then disperses further downstream and can contribute to the development of strong cyclogenesis or atmospheric blocking anticyclones (Riboldi et al., 2019). Therefore, ET associated with amplification of RWPs may also lead to high-impact weather in distant downstream regions (Keller et al., 2019). The many different atmospheric processes involved in ET events pose a major challenge for NWP models and can considerably reduce the skill of the medium-range forecasts downstream of the tropical cyclone (Jones et al., 2003).
It is suggested that the evolution of the reduction in the 48 h forecast error displayed in the Hovmöller diagram is related to the interaction of Cyclone Fay undergoing ET with the midlatitude waveguide. The downward propagation of the forecast error reduction seems to be similar to the group velocity of the RWP. This picture fits the theory described by Keller et al. (2019), in which uncertainties regarding the ability to predict ET events typically first manifest as uncertainties in the prediction of the strengthening of the downstream ridge and then propagate with the evolving RWP.
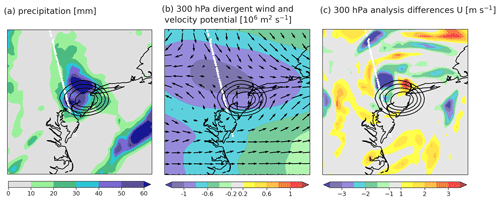
Figure 6(a) Precipitation, (b) 300 hPa divergent wind (vectors) and velocity potential, and (c) the analysis differences in 300 hPa zonal wind between EXP_A and CTRL for 10 July 2020 at 12:00 UTC for the region around Cyclone Fay (35–45∘ N, 80–70∘ W). The black contours represent the minimum surface pressure.
Figure 6 focuses on the time around the onset of the ET of Cyclone Fay. The contour lines are the minimum surface pressure showing Cyclone Fay making landfall. Pronounced values in the divergent wind field of the upper troposphere (Fig. 6b) occur northwest of the cyclone center, representing the poleward-expanding and anticyclonically rotating outflow. Characteristic for ET events are the regions of significant precipitation embedded in the cloud shield apparent in Fig. 6a. The rain field tends to be located to the west of the cyclone center. The Mie wind observations from Aeolus assimilated at this time cover the area of the diverging flow very well, which could be the origin of the forecast improvements in the Hovmöller diagram right at the beginning of the RWP development. The differences in zonal wind at 300 hPa (Fig. 6c) exhibit an increased influence of Aeolus observations in terms of both western and eastern wind acceleration around the region of the interaction of the cyclone with the large-scale flow.
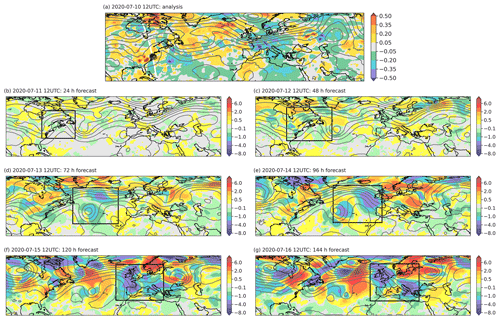
Figure 7Downstream impact of Aeolus at 500 hPa geopotential from forecast start on 10 July 2020 at 12:00 UTC for the region (20–70∘ N, 110∘ W–70∘ E). (a) Differences in analysis between EXP_A and CTRL for 10 July 2020 at 12:00 UTC: the white line is the Aeolus track; the black contours are the minimum surface pressure. (b) Differences in 24 h forecast error (EXP_A − CTRL) for 11 July 2020 at 12:00 UTC. (c) Differences in 48 h forecast error for 12 July 2020 at 12:00 UTC. (d) Differences in 72 h forecast error for 13 July 2020 at 12:00 UTC. (e) Differences in 96 h forecast error for 14 July 2020 at 12:00 UTC. (f) Differences in 120 h forecast error for 15 July 2020 at 12:00 UTC. (g) Differences in 144 h forecast error for 16 July 2020 at 12:00 UTC. The box highlights the area of interest associated with the ET event; the grey contour lines represent the 500 hPa geopotential from the ERA5 reanalysis in 5 gpdm (geopotential decameter) steps.
The forecast impact in the course of the downstream development following the ET event is displayed in Fig. 7 by the differences in forecast errors in the 500 hPa geopotential between EXP_A and CTRL for lead times from 24 to 144 h. All forecasts are initialized on 10 July 2020 at 12:00 UTC. Figure 7a shows the analysis differences at this time, when Cyclone Fay is located ahead of the upstream trough of the jet and the Aeolus track passes directly over the cyclone and trough front. Larger analysis influence is visible in the areas around the cyclone, especially at the trough axis. The evolution of the Aeolus impact described in the following is believed to be related to better coverage of upper-troposphere outflow associated with the latent heat release of Cyclone Fay or/and reduction in uncertainties in the dry baroclinic upper-level flow dynamics. In the 24 h forecast (Fig. 7b), only small effects of the Aeolus observations on the midlatitude flow are visible in the region around the amplifying ridge, presumably related to the outflow. However, due to the assimilation cycling, the effects cannot be clearly assigned, but it is assumed that previous measurements and the cycling lead to differences in the cyclone as well as in the jet environment. The improvements become more distinct in the 48 h forecast (Fig. 7c), where the area of forecast error reduction moved towards the deepening trough downstream; 1 d later (Fig. 7d), a distinct RWP with a ridge–trough couplet and cyclogenesis over the northern Atlantic Ocean developed. This cyclogenesis and the region of the jet streak above are associated with a large reduction in 72 h forecast error. Subsequently, the impact propagated downstream over the eastern Atlantic (Fig. 7e). Figure 7f shows a clear wave structure in the 500 hPa geopotential, from the eastern coast of North America to Europe. The 120 and 144 h forecast error differences (Fig. 7f, g) highlight the area of increased error reduction spreading eastward across the whole of Europe. This spatial perspective of the individual forecast times along the wave packet further supports the assumption that the downstream forecast improvement is related to the Aeolus observations in the area of the ET of Cyclone Fay and preceding nearby observations.
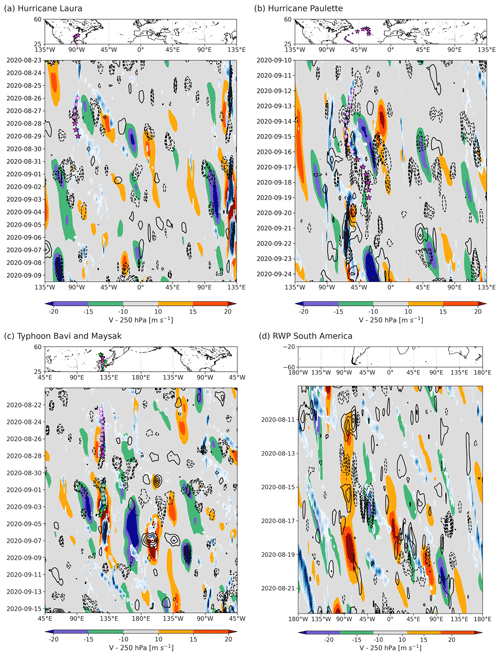
Figure 8Same as Fig. 5 for the ET event of (a) Hurricane Laura, (b) Hurricane Paulette, and (c) Typhoon Bavi and Typhoon Maysak and (d) an RWP development around the cyclogenesis region over South America.
The data denial experiment period includes a large part of the tropical cyclone season, and we detected additional ET cases associated with forecast error reduction by the assimilation of Aeolus observations. Figure 8 shows three further examples of Hovmöller diagrams for ET and the spatiotemporal evolution of RWPs as well as differences in 500 hPa geopotential forecast error covering the time period of Hurricane Laura (Fig. 8a), Hurricane Paulette (Fig. 8b), and Typhoon Bavi and Typhoon Maysak in the western Pacific (Fig. 8c). As in Fig. 5, wave-like structures in the meridional wind component that form or intensify around the beginning of the ET are visible. However, the RWPs are not as pronounced as in Cyclone Fay's ET event. Furthermore, the correspondence between the propagation velocity of the impact structures and the RWP does not seem to be as clear. But it was found that the area around the upper-level divergent outflow of the ET examples in Fig. 8a–c was well captured by the Aeolus Mie observations during the onset of the transition (not shown here). Therefore, some impact on the downstream development of the RWPs can be assumed. We also investigated the impact of Aeolus observations on tropical cyclone tracks. However, no significant improvements were found. This may be related to the lack of observations beneath clouds and consequently within and nearby the cyclone, but it could also be due to the need for a large sample size when evaluating tropical cyclone tracks.
Furthermore, Fig. 8d shows an example of an RWP developing in the region over southern South America, which is expected to be related to the clear structure of the largest forecast error reduction in 500 hPa geopotential. Typically, in the Southern Hemisphere winter season, cyclogenesis is associated with the subtropical jet in the lee of the Andes in South America (Hoskins and Hodges, 2005). Berbery and Barros (2002) discussed the strong moisture transport in winter and spring from the tropics into the La Plata River basin on the eastern side of the Andes and the importance of associated latent heat release for subtropical cyclogenesis process. The increased beneficial impact of the Aeolus observations in this region along the subtropical waveguide on the Southern Hemisphere has already been shown in the mean forecast error reduction in 500 hPa geopotential in Sect. 3 (Fig. 1). This might be related to good Mie wind observations in moist areas associated with cyclogenesis, capturing the onset or modification of orographically excited RWPs. Overall, there is significantly less knowledge available for the storm tracks in the Southern Hemisphere than in the Northern Hemisphere, which has been observed and examined more comprehensively in the past.
The importance of upscale error growth from convective to larger atmospheric scales limiting the predictability in NWP is discussed in several recent studies (e.g., Hohenegger and Schär, 2007; Rodwell et al., 2013; Selz and Craig, 2015; Selz et al., 2022). Selz et al. (2022) found that latent heat release in convective systems and the divergent component of the atmospheric flow dominate the error growth with respect to physical processes. It is assumed that accurate observations of the divergent wind field as expected from the Aeolus winds can reduce the uncertainty in the initial conditions around mesoscale convective ET events, as well as in cyclogenesis regions in other parts of the world. Overall, the results of this section show potential for further investigation of the dynamical effects of the assimilation of the Aeolus wind observations for the higher latitudes in the both Northern Hemisphere and Southern Hemisphere.
The observations of the DWL satellite mission Aeolus provide wind profiles in the troposphere and the lower stratosphere with quasi-global coverage. A 3-month OSE from July to October 2020 was conducted to evaluate the impact of the Aeolus horizontal line-of-sight (HLOS) wind observations in the ICON system's operational global data assimilation system. In a preceding study (Martin et al., 2022), it was shown that the Aeolus observations improve both analyses and forecasts, with the highest impact in the tropics around the tropopause level. However, a relatively large forecast error reduction of up to 2 % was also found from global statistics in the midlatitudes. So far, the dynamical processes responsible for improving the forecast have only been given little consideration. Therefore, the main objective of this study is to highlight indications of atmospheric features and dynamical changes constituting pathways for significant impact of the Aeolus observations for future research studies. Based on the global statistical analysis, regions of enhanced forecast error reduction were chosen to discuss the underlying dynamics.
Two important large-scale tropical circulation systems – the QBO and ENSO – appear to be very well measured by Aeolus during the time that is associated with a shift in the oscillatory phase. The change in the stratospheric jet from easterly to westerly in August 2020 was found to be related to the high positive impact of the Aeolus winds. Further investigation suggests that the impact of 10 %–15 % in the equatorial band around 50 hPa is largely amplified by a special QBO range bin setting that has been adjusted to account for atmospheric winds up to altitudes of 25 km on a single day per week. This would mean that the improvements are mainly due to good measurements of the upper atmosphere rather than improved detection of propagating waves from the troposphere. However, a longer accompanying experiment would be necessary for a precise assessment, especially since the stratosphere generally exhibits large uncertainties in NWP models. Furthermore, in August 2020, the tropical troposphere in the Pacific Ocean was characterized by large-scale dynamic variability. The El Niño–Southern Oscillation state changed from the normal to the La Niña phase. The associated strengthening of upper-tropospheric and midtropospheric wind patterns in the region of the eastern Pacific related to the zonal Walker circulation is advantageously captured by Aeolus. The QBO and ENSO are typically characterized by teleconnections with other atmospheric systems through, e.g., other oscillation phenomena in the extratropics or wave activity. Therefore, the variability in the tropics can lead to large uncertainties in many parts of the world. In addition to short- and medium-range NWP, seasonal and subseasonal forecasts can benefit from the potential of the Aeolus observations to better represent the initial state of fluctuations in such large-scale circulation systems.
In addition to the tropics, the influence of Aeolus observations on midlatitude dynamics was investigated. Although especially continents are already comparably well observed by other observation types, the HLOS winds have, on average, a relatively high beneficial impact. However, the spatial distribution shows that the effects appear to be partially masked by fluctuations in the 3-month mean flow. Systematically positive impact particularly stands out in storm track regions in the Southern Hemisphere, but some average improvements are also seen in the Northern Hemisphere. Furthermore, it was found that the development of an RWP likely associated with cyclogenesis in the lee of the Andes in South America may be related to the reduction in large forecast errors.
For future studies, we recommend long-term experiments in combination with feature-based diagnostics to further investigate the influence of HLOS winds on tropical cyclones and the upscale interaction and representation of error growth. Since tropical cyclones are not common events, a long integration period is necessary to achieve a sufficiently large sample and thus robust results. A longer OSE would also be required to further study the impact on other large-scale convective circulation systems, such as the Madden–Julian Oscillation (MJO), that affect weekly to monthly forecast skills. Besides the Northern Hemisphere and tropical dynamics, a better assessment of the large impacts found in the southern parts of the world would be an interesting research focus, especially since the causes of forecast busts in the Northern Hemisphere are much better understood.
The Aeolus L2B data can be downloaded from https://aeolus-ds.eo.esa.int/oads/access/collection/L2B_Wind_Products/ (last access: 22 March 2023; ESA, 2023). The ERA5 data can be obtained from https://doi.org/10.24381/cds.bd0915c6 (Hersbach et al., 2023). The OSE dataset (created by Alexander Cress) can be made available upon reasonable request.
AM performed the data analysis and prepared the main part of the publication. MW supervised the work, conceived the research concept, and contributed to the development of methods and analysis of the data. AC conducted the experiment at DWD and provided important information on their use and interpretation. All co-authors engaged in discussions and contributed to the interpretation of the results.
The contact author has declared that none of the authors has any competing interests.
Publisher’s note: Copernicus Publications remains neutral with regard to jurisdictional claims in published maps and institutional affiliations.
This article is part of the special issue “Aeolus data and their application (AMT/ACP/WCD inter-journal SI)”. It is not associated with a conference.
We want to thank the European Space Agency (ESA) for providing the datasets and the experts from the Experimental Validation And Assimilation (EVAA) consortium (Ludwig-Maximilians-Universität, LMU; Deutscher Wetterdienst, DWD; Deutsches Zentrum für Luft- und Raumfahrt, DLR), the Data Innovation and Science Cluster (DISC), and the Aeolus Cal/Val community for valuable discussions, particularly Oliver Reitebuch, Michael Rennie and Alexander Geiss. Furthermore, the exchange of tools and discussion with Maurus Borne and Peter Knippertz from W2W is greatly appreciated.
This research has been supported by the German Federal Ministry for Economic Affairs and Climate Action (BMWK) (FKZ/grant no. 50EE1721A) and the Transregional Collaborative Research Center Waves to Weather (W2W) (SFB/TRR165) funded by the German Research Foundation (DFG).
This paper was edited by Ad Stoffelen and reviewed by Erland Kallen and one anonymous referee.
Anstey, J. A. and Shepherd, T. G.: High-latitude influence of the quasi-biennial oscillation, Q. J. Roy. Meteor. Soc., 140, 1–21, https://doi.org/10.1002/qj.2132, 2014. a
Anstey, J. A., Banyard, T. P., Butchart, N., Coy, L., Newman, P. A., Osprey, S., and Wright, C. J.: Prospect of Increased Disruption to the QBO in a Changing Climate, Geophys. Res. Lett., 48, e2021GL093058, https://doi.org/10.1029/2021GL093058, 2021. a, b
Baker, W. E., Atlas, R., Cardinali, C., Clement, A., Emmitt, G. D., Gentry, B. M., Hardesty, R. M., Källén, E., Kavaya, M. J., Langland, R., Ma, Z., Masutani, M., McCarty, W., Pierce, R. B., Pu, Z., Riishojgaard, L. P., Ryan, J., Tucker, S., Weissmann, M., and Yoe, J.: Lidar-measured wind profiles: The missing link in the Global Observing System, B. Am. Meteorol. Soc., 95, 543–564, https://doi.org/10.1175/BAMS-D-12-00164.1, 2014. a
Baldwin, M. P., Gray, L. J., Dunkerton, T. J., Hamilton, K., Haynes, P. H., Randel, W. J., Holton, J. R., Alexander, M. J., Hirota, I., Horinouchi, T., Jones, D. B. A., Kinnersley, J. S., Marquardt, C., Sato, K., and Takahashi, M.: The quasi-biennial oscillation, Rev. Geophys., 39, 179–229, https://doi.org/10.1029/1999RG000073, 2001. a, b
Bauer, P., Thorpe, A., and Brunet, G.: The quiet revolution of numerical weather prediction, Nature, 525, 47–55, https://doi.org/10.1038/nature14956, 2015. a
Berbery, E. H. and Barros, V. R.: The Hydrologic Cycle of the La Plata Basin in South America, J. Hydrometeorol., 3, 630–645, https://doi.org/10.1175/1525-7541(2002)003<0630:THCOTL>2.0.CO;2, 2002. a
Desroziers, G., Berre, L., Chapnik, B., and Poli, P.: Diagnosis of observation, background and analysis-error statistics in observation space, Q. J. Roy. Meteor. Soc., 131, 3385–3396, https://doi.org/10.1256/qj.05.108, 2005. a
ESA: Atmospheric dynamics mission, Mission Selection Rep., ESA SP-1233(4), ISBN 92-9092-528-0, 1999. a
ESA: ADM-Aeolus Science Report, ESA SP-1311, 121 pp., https://earth.esa.int/documents/10174/1590943/AEOL002.pdf (last access: 22 March 2023), 2008. a, b
ESA: ADM-Aeolus Mission Requirements Documents, ESA EOP-SM/2047, 57 pp., http://esamultimedia.esa.int/docs/EarthObservation/ADM-Aeolus_MRD.pdf (last access: 22 March 2023), 2016. a
ESA: Aeolus Online Dissemination System [data set], https://aeolus-ds.eo.esa.int/oads/access/collection/L2B_Wind_Products/, last acess: 22 March 2023. a
Garrett, K., Liu, H., Ide, K., Hoffman, R. N., and Lukens, K. E.: Optimization and impact assessment of Aeolus HLOS wind assimilation in NOAA's global forecast system, Q. J. Roy. Meteor. Soc., 148, 2703–2716, https://doi.org/10.1002/qj.4331, 2022. a, b
Grams, C. M., Jones, S. C., Davis, C. A., Harr, P. A., and Weissmann, M.: The impact of Typhoon Jangmi (2008) on the midlatitude flow. Part I: Upper-level ridgebuilding and modification of the jet, Q. J. Roy. Meteor. Soc., 139, 2148–2164, https://doi.org/10.1002/qj.2091, 2013. a
Gray, L. J., Anstey, J. A., Kawatani, Y., Lu, H., Osprey, S., and Schenzinger, V.: Surface impacts of the Quasi Biennial Oscillation, Atmos. Chem. Phys., 18, 8227–8247, https://doi.org/10.5194/acp-18-8227-2018, 2018. a
Gray, W. M.: Atlantic Seasonal Hurricane Frequency. Part I: El Niño and 30 mb Quasi-Biennial Oscillation Influences, Mon. Weather Rev., 112, 1649–1668, https://doi.org/10.1175/1520-0493(1984)112<1649:ASHFPI>2.0.CO;2, 1984. a
Gray, W. M., Sheaffer, J. D., and Knaff, J. A.: Influence of the Stratospheric QBO on ENSO Variability, J. Meteorol. Soc. Jpn. Ser. II, 70, 975–995, https://doi.org/10.2151/jmsj1965.70.5_975, 1992. a
Hersbach, H., Bell, B., Berrisford, P., Hirahara, S., Horányi, A., Muñoz-Sabater, J., Nicolas, J., Peubey, C., Radu, R., Schepers, D., Simmons, A., Soci, C., Abdalla, S., Abellan, X., Balsamo, G., Bechtold, P., Biavati, G., Bidlot, J., Bonavita, M., De Chiara, G., Dahlgren, P., Dee, D., Diamantakis, M., Dragani, R., Flemming, J., Forbes, R., Fuentes, M., Geer, A., Haimberger, L., Healy, S., Hogan, R. J., Hólm, E., Janisková, M., Keeley, S., Laloyaux, P., Lopez, P., Lupu, C., Radnoti, G., de Rosnay, P., Rozum, I., Vamborg, F., Villaume, S., and Thépaut, J.-N.: The ERA5 global reanalysis, Q. J. Roy. Meteor. Soc., 146, 1999–2049, https://doi.org/10.1002/qj.3803, 2020. a, b
Hersbach, H., Bell, B., Berrisford, P., Biavati, G., Horányi, A., Muñoz Sabater, J., Nicolas, J., Peubey, C., Radu, R., Rozum, I., Schepers, D., Simmons, A., Soci, C., Dee, D., and Thépaut, J.-N.: ERA5 hourly data on pressure levels from 1940 to present, Copernicus Climate Change Service (C3S) Climate Data Store (CDS) [data set], https://doi.org/10.24381/cds.bd0915c6, 2023. a
Hohenegger, C. and Schär, C.: Predictability and Error Growth Dynamics in Cloud-Resolving Models, J. Atmos. Sci., 64, 4467–4478, https://doi.org/10.1175/2007JAS2143.1, 2007. a
Horel, J. D. and Wallace, J. M.: Planetary-Scale Atmospheric Phenomena Associated with the Southern Oscillation, Mon. Weather Rev., 109, 813–829, https://doi.org/10.1175/1520-0493(1981)109<0813:PSAPAW>2.0.CO;2, 1981. a
Hoskins, B. J. and Hodges, K. I.: A New Perspective on Southern Hemisphere Storm Tracks, J. Climate, 18, 4108–4129, https://doi.org/10.1175/JCLI3570.1, 2005. a
Hoskins, B. J. and Karoly, D. J.: The Steady Linear Response of a Spherical Atmosphere to Thermal and Orographic Forcing, J. Atmos. Sci., 38, 1179–1196, https://doi.org/10.1175/1520-0469(1981)038<1179:TSLROA>2.0.CO;2, 1981. a
Iza, M., Calvo, N., and Manzini, E.: The Stratospheric Pathway of La Niña, J. Climate, 29, 8899–8914, https://doi.org/10.1175/JCLI-D-16-0230.1, 2016. a
Jones, S. C., Harr, P. A., Abraham, J., Bosart, L. F., Bowyer, P. J., Evans, J. L., Hanley, D. E., Hanstrum, B. N., Hart, R. E., Lalaurette, F., Sinclair, M. R., Smith, R. K., and Thorncroft, C.: The Extratropical Transition of Tropical Cyclones: Forecast Challenges, Current Understanding, and Future Directions, Weather Forecast., 18, 1052–1092, https://doi.org/10.1175/1520-0434(2003)018<1052:TETOTC>2.0.CO;2, 2003. a, b, c
Jury, M. R., Pathack, B., and Parker, B.: Climatic Determinants and Statistical Prediction of Tropical Cyclone Days in the Southwest Indian Ocean, J. Climate, 12, 1738–1746, http://www.jstor.org/stable/26244387 (last access: 22 March 2023), 1999. a
Keller, J. H., Grams, C. M., Riemer, M., Archambault, H. M., Bosart, L., Doyle, J. D., Evans, J. L., Galarneau, T. J., Griffin, K., Harr, P. A., Kitabatake, N., McTaggart-Cowan, R., Pantillon, F., Quinting, J. F., Reynolds, C. A., Ritchie, E. A., Torn, R. D., and Zhang, F.: The Extratropical Transition of Tropical Cyclones. Part II: Interaction with the Midlatitude Flow, Downstream Impacts, and Implications for Predictability, Mon. Weather Rev., 147, 1077–1106, https://doi.org/10.1175/MWR-D-17-0329.1, 2019. a, b
Klein, P. M., Harr, P. A., and Elsberry, R. L.: Extratropical Transition of Western North Pacific Tropical Cyclones: An Overview and Conceptual Model of the Transformation Stage, Weather Forecast., 15, 373–395, https://doi.org/10.1175/1520-0434(2000)015<0373:ETOWNP>2.0.CO;2, 2000. a
Laloyaux, P., Bonavita, M., Dahoui, M., Farnan, J., Healy, S., Hólm, E., and Lang, S. T. K.: Towards an unbiased stratospheric analysis, Q. J. Roy. Meteorol. Soc., 146, 2392–2409, https://doi.org/10.1002/qj.3798, 2020. a
Laroche, S. and St-James, J.: Impact of the Aeolus Level-2B horizontal line-of-sight winds in the Environment and Climate Change Canada global forecast system, Q. J. Roy. Meteor. Soc., 148, 2047–2062, https://doi.org/10.1002/qj.4300, 2022. a, b
Marseille, G., Stoffelen, A., and Barkmeijer, J.: Impact assessment of prospective spaceborne Doppler wind lidar observation scenarios, Tellus A, 60A, 234–248, https://doi.org/10.1111/j.1600-0870.2007.00289.x, 2008a. a
Marseille, G., Stoffelen, A., and Barkmeijer, J.: A cycled sensitivity observing system experiment on simulated Doppler wind lidar data during the 1999 Christmas storm “Martin”, Tellus A, 60A, 249–260, https://doi.org/10.1111/j.1600-0870.2007.00290.x, 2008b. a
Martin, A., Weissmann, M., Reitebuch, O., Rennie, M., Geiß, A., and Cress, A.: Validation of Aeolus winds using radiosonde observations and numerical weather prediction model equivalents, Atmos. Meas. Tech., 14, 2167–2183, https://doi.org/10.5194/amt-14-2167-2021, 2021. a, b
Martin, A., Weissmann, M., and Cress, A.: Impact of assimilating Aeolus observations in the global model ICON: A global statistical overview, https://ucloud.univie.ac.at/index.php/s/DIP0XxSgKA6eXek (last access: 22 March 2023), 2022. a, b, c, d, e, f, g, h, i, j, k, l, m
Martin, Z., Son, S. W., Butler, A., Hendon, H., Kim, H., Sobel, A., Yoden, S., and Zhang, C.: The influence of the quasi-biennial oscillation on the Madden–Julian oscillation, Nature Reviews Earth & Environment, 2, 477–489, https://doi.org/10.1038/s43017-021-00173-9, 2021. a
Pourret, V., Šavli, M., Mahfouf, J.-F., Raspaud, D., Doerenbecher, A., Bénichou, H., and Payan, C.: Operational assimilation of Aeolus winds in the Météo-France global NWP model ARPEGE, Q. J. Roy. Meteor. Soc., 148, 2652–2671, https://doi.org/10.1002/qj.4329, 2022. a, b
Pu, Z., Zhang, L., and Emmitt, G. D.: Impact of airborne Doppler wind lidar profiles on numerical simulations of a tropical cyclone, Geophys. Res. Lett., 37, L05801, https://doi.org/10.1029/2009GL041765, 2010. a
Reinert, D., Prill, F., Frank, H., Denhard, M., Baldauf, M., Schraff, C., Gebhardt, C., Marsigli, C., and Zängl, G.: DWD Database Reference for the Global and Regional ICON and ICON-EPS Forecasting System, Deutscher Wetterdienst, Business Area “Research and Development”, https://www.dwd.de/DWD/forschung/nwv/fepub/icon_database_main.pdf, last access: 22 March 2023. a
Rennie, M., Isaksen, L., Weiler, F., de Kloe, J., Kanitz, T., and Reitebuch, R.: The impact of Aeolus wind retrievals on ECMWF global weather forecast, Q. J. Roy. Meteor. Soc., 147, 1–32, https://doi.org/10.1002/qj.4142, 2021. a, b, c
Riboldi, J., Grams, C. M., Riemer, M., and Archambault, H. M.: A Phase Locking Perspective on Rossby Wave Amplification and Atmospheric Blocking Downstream of Recurving Western North Pacific Tropical Cyclones, Mon. Weather Rev., 147, 567–589, https://doi.org/10.1175/MWR-D-18-0271.1, 2019. a
Rodwell, M. J., Magnusson, L., Bauer, P., Bechtold, P., Bonavita, M., Cardinali, C., Diamantakis, M., Earnshaw, P., Garcia-Mendez, A., Isaksen, L., Källén, E., Klocke, D., Lopez, P., McNally, T., Persson, A., Prates, F., and Wedi, N.: Characteristics of Occasional Poor Medium-Range Weather Forecasts for Europe, B. Am. Meteorol. Soc., 94, 1393–1405, https://doi.org/10.1175/BAMS-D-12-00099.1, 2013. a
Rogers, J. C.: The Association between the North Atlantic Oscillation and the Southern Oscillation in the Northern Hemisphere, Mon. Weather Rev., 112, 1999–2015, https://doi.org/10.1175/1520-0493(1984)112<1999:TABTNA>2.0.CO;2, 1984. a
Šavli, M., Pourret, V., Payan, C., and Mahfouf, J.-F.: Sensitivity of Aeolus HLOS winds to temperature and pressure specification in the L2B processor, Atmos. Meas. Tech., 14, 4721–4736, https://doi.org/10.5194/amt-14-4721-2021, 2021. a
Selz, T. and Craig, G. C.: Upscale Error Growth in a High-Resolution Simulation of a Summertime Weather Event over Europe, Mon. Weather Rev., 143, 813–827, https://doi.org/10.1175/MWR-D-14-00140.1, 2015. a
Selz, T., Riemer, M., and Craig, G.: The transition from practical to intrinsic predictability of midlatitude weather, J. Atmos. Sci., 79, 2013–2030, https://doi.org/10.1175/JAS-D-21-0271.1, 2022. a, b
Shepherd, T., Polichtchouk, I., Hogan, R., and Simmons, A.: Report on Stratosphere Task Force, ECMWF Technical Memoranda, https://doi.org/10.21957/0vkp0t1xx, 2018. a
Stoffelen, A., Marseille, G.-J., Bouttier, F., Vasiljevic, D., de Haan, S., and Cardinali, C.: ADM-Aeolus Doppler wind lidar observing system simulation experiment, Q. J. Roy. Meteor. Soc., 132, 1927–1947, https://doi.org/10.1256/qj.05.83, 2006. a
Tan, D. G. H., Andersson, E., Fisher, M., and Isaksen, L.: Observing-system impact assessment using a data assimilationensemble technique: application to the ADM Aeolus wind profiling mission, Q. J. Roy. Meteor. Soc., 133, 381–390, https://doi.org/10.1002/qj.43, 2007. a
Tan, D. G. H., Andersson, E., Kloe, J. D., Marseille, G.-J., Stoffelen, A., Poli, P., Denneulin, M.-L., Dabas, A., Huber, D., Reitebuch, O., Flamant, P., Rille, O. L., and Nett, H.: The ADM-Aeolus wind retrieval algorithms, Tellus A, 60, 191–205, https://doi.org/10.1111/j.1600-0870.2007.00285.x, 2008. a
Weiler, F., Rennie, M., Kanitz, T., Isaksen, L., Checa, E., de Kloe, J., Okunde, N., and Reitebuch, O.: Correction of wind bias for the lidar on board Aeolus using telescope temperatures, Atmos. Meas. Tech., 14, 7167–7185, https://doi.org/10.5194/amt-14-7167-2021, 2021. a
Weissmann, M. and Cardinali, C.: Impact of airborne Doppler lidar observations on ECMWF forecasts, Q. J. Roy. Meteor. Soc., 133, 107–116, https://doi.org/10.1002/qj.16, 2007. a
Weissmann, M., Langland, R. H., Cardinali, C., Pauley, P. M., and Rahm, S.: Influence of airborne Doppler wind lidar profiles near Typhoon Sinlaku on ECMWF and NOGAPS forecasts, Q. J. Roy. Meteor. Soc., 138, 118–130, https://doi.org/10.1002/qj.896, 2012. a
Wirth, V., Riemer, M., Chang, E. K. M., and Martius, O.: Rossby Wave Packets on the Midlatitude Waveguide – A Review, Mon. Weather Rev., 146, 1965–2001, https://doi.org/10.1175/MWR-D-16-0483.1, 2018. a
Žagar, N.: Assimilation of equatorial waves by line of sight wind observations, J. Atmos. Sci., 61, 1877–1893, https://doi.org/10.1175/1520-0469(2004)061<1877:AOEWBL>2.0.CO;2, 2004. a
Žagar, N., Stoffelen, A., Marseille, G., Accadia, C., and Schlüssel, P.: Impact Assessment of Simulated Doppler Wind Lidars with a Multivariate Variational Assimilation in the Tropics, Mon. Weather Rev., 136, 2443–2460, https://doi.org/10.1175/2007MWR2335.1, 2008. a
Zängl, G., Reinert, D., Rípodas, P., and Baldauf, M.: The ICON (ICOsahedral Non-hydrostatic) modelling framework of DWD and MPI-M: Description of the non-hydrostatic dynamical core, Q. J. Roy. Meteor. Soc., 141, 563–579, https://doi.org/10.1002/qj.2378, 2015. a
- Abstract
- Introduction
- Data and methods
- Global forecast error reduction
- Dynamical scenarios leading to pronounced forecast improvements
- Conclusions
- Data availability
- Author contributions
- Competing interests
- Disclaimer
- Special issue statement
- Acknowledgements
- Financial support
- Review statement
- References
- Abstract
- Introduction
- Data and methods
- Global forecast error reduction
- Dynamical scenarios leading to pronounced forecast improvements
- Conclusions
- Data availability
- Author contributions
- Competing interests
- Disclaimer
- Special issue statement
- Acknowledgements
- Financial support
- Review statement
- References