the Creative Commons Attribution 4.0 License.
the Creative Commons Attribution 4.0 License.
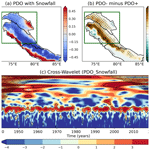
Pacific Decadal Oscillation-driven interdecadal variability of snowfall over the Karakoram and the Western Himalayas
Priya Bharati
Kieran Mark Rainwater Hunt
Our study reveals that the negative phase of the Pacific Decadal Oscillation (PDO) leads to increased winter (DJF) snowfall in the Karakoram and Western Himalayas (KH) from 1940 to 2022. Interdecadal variations in DJF snowfall during the negative phase of PDO are attributed to deep convection and adiabatic cooling near the tropopause in both the northwestern Pacific and the KH region. Additionally, a wave-like pattern characterized by a trough (anomalous cyclone) north of the KH and a ridge (anomalous Tibetan Plateau anticyclone) east of the KH in the upper atmosphere, along the northward shift of the DJF subtropical westerly jet (STJ), was observed. A strong positive correlation between the DJF STJ strength and DJF snowfall in the KH, as well as a significant negative correlation between the DJF STJ strength and the DJF PDO, suggests a wave response over the KH to the direct forcing over the northwestern Pacific Ocean. The intensified STJ across the KH results in higher frequency of western disturbances, leading to anomalous moisture convergence and increased DJF precipitation in the region during the PDO−. These findings hold significant implications for the decadal predictability of winter snowfall in the KH by the various phases of the PDO.
- Article
(9957 KB) - Full-text XML
- BibTeX
- EndNote
Glaciers in the Karakoram and the Western Himalayas (KH) exhibit unique stability compared to other alpine glaciers (known as the “Karakoram anomaly”; Hewitt, 2005; Kääb et al., 2012; Gardelle et al., 2012; Kapnick et al., 2014; Roy et al., 2019). Winter snowfall plays a significant role in preserving the local snowpack and sustaining the glacial mass balance at higher elevations (Tahir et al., 2011; Bolch et al., 2012; Ridley et al., 2013; Cannon et al., 2015; Dimri et al., 2015) and controls almost 60 % of the variability in glacier mass balance in the KH region (Kumar et al., 2019). The decline in average and minimum summer temperatures and the significant increases in winter, summer, and annual precipitation have been proposed as crucial factors influencing the stable glacier budget of the KH in recent decades (Archer and Fowler, 2004; Forsythe et al., 2017).
The KH receives around 50 % of its annual precipitation as snowfall from western disturbances (WDs) (Lang and Barros, 2004; Barros et al., 2006; Bookhagen and Burbank, 2010; Hunt et al., 2024). Furthermore, WDs account for more than 65 % of all winter snowfall and nearly 53 % of total winter precipitation in the KH (Javed et al., 2022). However, using a less conservative method, Midhuna et al. (2020) found that WDs account for about 80 % of winter precipitation in the KH. WDs are upper-level troughs in the subtropical westerly jet (STJ), which grow via baroclinic instability (Norris et al., 2015; Cannon et al., 2017; Hunt et al., 2018). Strong WDs are associated with deep uplift to the east of their centre and drive moist lower-tropospheric southwesterlies from the Arabian Sea (Dimri and Dash, 2012; Hunt et al., 2017), resulting in heavy precipitation along the foothills and mountains of the KH region (Baudouin et al., 2020). The snowfall from WDs in the KH is heavily influenced by the complex topography of the region, as well as by synoptic and mesoscale factors (Cannon et al., 2015; Norris et al., 2015, 2017, 2019). Subsequent snowmelt in the following spring and summer seasons and the associated runoff serve as major sources of downstream river flow and provide relief from drought to populations that are vulnerable to water stress (Bolch et al., 2012; Hewitt, 2014; Rana et al., 2019; Pritchard, 2019).
However, the main climatic drivers affecting seasonal precipitation, and hence glacial mass balance in the region, are only partially understood (Cannon et al., 2015). WD activity during winter season over the KH has been reported to be influenced by several global climate forcings such as the North Atlantic Oscillation–Arctic Oscillation (Yadav et al., 2009; Syed et al., 2010; Filippi et al., 2014; Basu et al., 2017; Midhuna and Dimri, 2019; Hunt and Zaz, 2023), the El Niño–Southern Oscillation (ENSO) (Yadav et al., 2010; Dimri, 2013; Kar and Rana, 2014; Cannon et al., 2017; Kamil et al., 2019; Rana et al., 2019; Bharati et al., 2025), the polar–Eurasian pattern and Siberian High (Wu and Wang, 2002; Cannon et al., 2015), the Madden–Julian Oscillation (Barlow et al., 2005; Cannon et al., 2017), and the Indian Ocean Dipole (IOD) (Yadav et al., 2007; Hoell et al., 2013) on intraseasonal and interannual timescales. In particular, the ENSO exerts the strongest influence on the interannual variability of winter precipitation in the KH (Rana et al., 2019). One of the key aspects of ENSO teleconnection to the Indian Himalayas is the southward shift in the latitude of the winter STJ over the KH during the positive phase of the ENSO (Cannon et al., 2015, 2017), which leads to heavier WD precipitation as their tracks move closer to their primary moisture source, the Arabian Sea (Bharati et al., 2025).
Precipitation gauges in the Himalayas are sparse and recognized as inadequate for accurately measuring snowfall (Anders et al., 2006; Rana et al., 2015). While satellite records of precipitation are available, they cover only a limited time frame, whereas our study requires long-term data to analyse the interdecadal variability of precipitation over the KH region. We currently have an 85-year-long reanalysis from ERA5, which has demonstrated a high degree of similarity in both the quantity and variability of winter precipitation across all timescales when compared to observations and satellite data in the KH region (e.g., Baudouin et al., 2020), though significant improvements in such reanalysis are possible (Beck et al., 2019). The long dataset from ERA5 is sufficient to examine the interdecadal variability of DJF snowfall over the KH. The low-frequency modes of atmospheric variability such as the Pacific Decadal Oscillation (PDO), the Interdecadal Pacific Oscillation (IPO) (Mantua et al., 1998; Zhang et al., 1997; Power et al., 1999; Deser et al., 2004; Dai, 2013), and the Atlantic Multidecadal Oscillation (AMO) (Enfield et al., 2001) are known to modulate the regional climate of the Northern Hemisphere over interdecadal to multidecadal timescales. Among these, the PDO is the dominant mode of sea surface temperature (SST) oscillation in the North Pacific, influencing long-term precipitation patterns globally (Dettinger et al., 1998; Krishnamurthy, 2014a, b; Wang et al., 2014; Dong and Dai, 2015; Yang et al., 2017; Wu and Mao, 2016; Qin et al., 2018; Aggarwal et al., 2024). For example, Indian monsoon rainfall and autumn precipitation in North-Central China were found to have an inverse relationship to the PDO (Krishnan and Sugi, 2003; Krishnamurthy, 2014a; Qin et al., 2018). According to Aggarwal et al. (2024), the PDO has a stronger positive correlation with pre-monsoon precipitation in the northwestern Himalayas compared to the ENSO and the IOD, leading to a significant decrease in precipitation in recent decades. However, there remains a significant gap in our understanding of the PDO's impact on precipitation over the Himalayas during both monsoon and non-monsoon seasons.
The current study aims to address this knowledge gap by examining the modulation of the interdecadal variability of winter snowfall over the KH by the PDO. Our study aims to understand the potential influence of the PDO on the Karakoram anomaly, which deviates from the general climate change patterns observed in the KH region and other mountainous areas. The main objectives of this study are to examine the following: (1) the spatial distribution of decadal snowfall in the KH in different phases of the PDO; (2) how the PDO adjusts global circulation patterns, leading to changes in the STJ; and (3) how these changes cause impacts on a local scale over the KH through WDs and moisture transport.
2.1 Data
2.1.1 Meteorological data
The study uses meteorological datasets including geopotential height, zonal (u) and meridional wind (v) at 200 hPa level, vertically averaged temperature from 500 to 300 hPa level, vertically integrated moisture flux (VIMF), vertically integrated moisture flux convergence (VIMFC), and global SST obtained from the European Centre for Medium-Range Weather Forecasts (ECMWF) ERA5 reanalysis from 1940 to 2022. The jet latitude and strength are computed by 200 hPa zonal winds over the region (50–80° E, 10–60° N). The jet latitude is the mean of the latitudes with the largest value of u for each longitude, and jet strength is the mean value of u along these latitudes. ERA5 data have global coverage at hourly frequency and a horizontal resolution of 0.25°.
2.1.2 Precipitation data
Precipitation in the KH is mainly observed through satellite-derived and reanalysis products (Bosilovich et al., 2008; Joshi et al., 2013; Ménégoz et al., 2013; Palazzi et al., 2013; Rana et al., 2015; Kishore et al., 2016; Baudouin et al., 2020) due to limited and unreliable observations from ground stations in this complex topographical region (Anders et al., 2006; Bookhagen and Burbank 2006; Strangeways, 2010; Rana et al., 2015; Dahri et al., 2018). The ERA5 reanalysis has frequently been used for precipitation and snow in recent studies over the KH (Dahri et al., 2018; Baudouin et al., 2020; Singh et al., 2021) and neighbouring mountainous areas (Hu and Yuan, 2021; Li et al. 2021; Dollan et al., 2024). ERA5 closely matches the most reliable gridded measurements over the KH in terms of number, seasonality, and variability across all timescales during winter (Baudouin et al., 2020). However, the accuracy of precipitation datasets varies depending on the season in the region. We choose ERA5 due to its long period, allowing decadal-scale analysis where other datasets do not.
To evaluate the performance of ERA5 precipitation, we compared it to various gridded precipitation datasets over the KH, such as reanalysis datasets from ECMWF ERA5-Land, Modern-Era Retrospective analysis for Research and Applications Version 2 (MERRA-2), and High Asia Refined analysis version 2 (HAR v2). We also compared it to rain-gauge-based precipitation from Climate Research Unit version 4.07 (CRU_TS v4.07), Global Precipitation Climatology Centre version 2022 (GPCC), Global Precipitation Climatology Project version 3.2 (GPCP v3.2), Asian Precipitation – Highly-Resolved Observational Data Integration Towards Evaluation of Water Resources (APHRODITE MA_v1101), and CPC Merged Analysis of Precipitation (CMAP), as well as satellite data from Tropical Rainfall Measuring Mission (TRMM) Multi-satellite Precipitation Analysis (TMPA) 3B43 and Integrated MultisatellitE Retrievals for GPM version 7 (GPM_IMERG v7).
We computed the linear correlation coefficient between area-averaged precipitation over the KH region (cropped by the shapefile of the traditional boundaries of the Karakoram and Western Himalayas; highlighted by a green box in Fig. 2a and b) in ERA5 and numerous other precipitation datasets. A strong correlation was seen between DJF ERA5 precipitation and rain-gauge-based precipitation products, including GPCC, GPCP, and CRU, with the exception of CMAP, which exhibited a correlation coefficient of 0.51 (Table 1). All reanalysis products, including ERA5, exhibit similar DJF precipitation variability as seen in observational and satellite datasets over the KH region. The variability of ERA5 precipitation in the KH region aligns closely with all available gridded datasets, despite the presence of biases in ERA5 precipitation across this region. Since most of DJF precipitation in the KH occurs as snowfall (Fig. 1b), we utilize ERA5 snowfall data to examine the decadal variability of snowfall in the KH (73–78° E, 33–38° N).
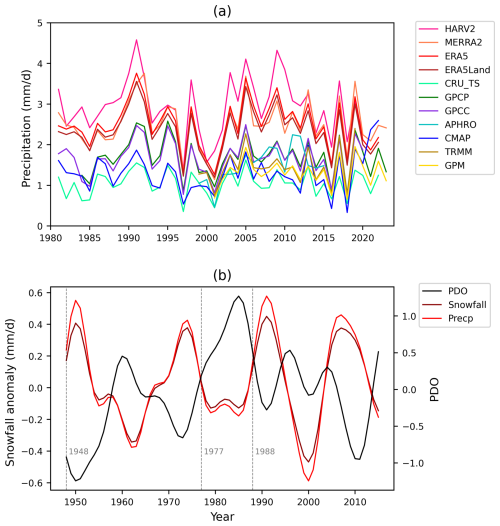
Figure 1(a) Seasonal variability of DJF precipitation in the KH region (highlighted by a green box in Fig. 2a and b; 73–78° E, 33–38° N) from ERA5, ERA5-Land, MERRA-2, HARv2, CRU_TS, GPCP, GPCC, and CMAP during the period from 1980 to 2020; APHRODITE from 1998 to 2015; TRMM from 1998 to 2019; and GPM from 2000 to 2023. (b) Time series of the 9-year filtered DJF PDO index and area-averaged DJF ERA5 snowfall (and precipitation) anomalies over the KH region from 1940 to 2022. The vertical grey lines represent phase transitions of the PDO.
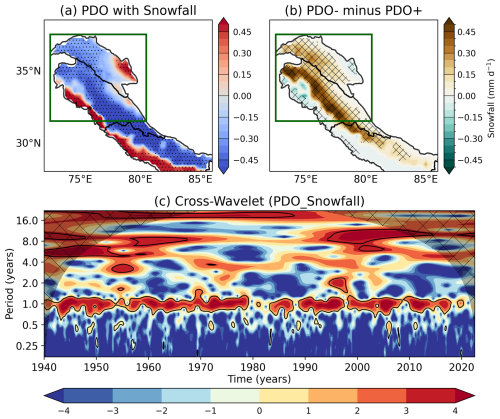
Figure 2(a) Spatial map of correlation between the 9-year filtered PDO index and the snowfall (mm) over the KH region (cropped by the shapefile of the traditional boundaries of the Karakoram–Western and Central Himalayas; highlighted by a green box; 73–78° E, 33–38° N) during DJF. (b) Composite difference of DJF snowfall (mm) between the negative and positive epoch of the PDO. (c) Cross-wavelet of DJF snowfall (mm) over the KH and the DJF PDO index from 1940 to 2022. Traditional boundaries of Karakoram–Western and Central Himalayan regions are marked by thick black lines in (a) and (b). Stippling in (a) and (b) denotes regions where the correlation and composite differences are significant at a 95 % confidence level, as determined by the two-tailed Student's t test and Welch's t test, respectively. Black line contours on the power spectra in (c) indicate where the spectral power of the cross-wavelet is significantly greater than zero at a 95 % confidence level.
2.1.3 PDO index
The PDO index from the National Oceanic and Atmospheric Administration National Climatic Data Center (NOAA-NDC) (https://www.ncei.noaa.gov/access/monitoring/pdo/, last access: 10 February 2024) is employed to describe the interdecadal variability of the Pacific Ocean over the period 1940 to 2022.
2.1.4 Western disturbance data
WD statistics are computed from the WD track catalogue described in Hunt et al. (2018) and Nischal et al. (2022), which is based on ERA5 reanalysis data that are spectrally truncated to T42 to remove noise and small-scale structures. The tracking algorithm detects WDs by identifying upper-tropospheric regions of positive relative vorticity averaged between 450 and 300 hPa, with the locations of candidate WDs identified as centroids of these regions. The candidate WDs are then further refined by only accepting the following: (1) those whose locations are linked through time to form tracks that generally follow the westerly steering winds associated with the STJ, (2) those that persist for at least 48 h, and (3) those that pass through northern India (50–77° E, 22–42.5° N). The northern limit of this box, 42.5° N, is more poleward than has been used in previous studies (36.5° N). This allows us to better capture WD impacts over the Karakoram.
2.2 Methods
2.2.1 Lanczos filter
To isolate the decadal signals, we linearly detrended all meteorological variables and the PDO index for DJF. These datasets were then filtered using a 9-year running mean Lanczos filter, which is a low-pass filter based on the sinc convolution (Duchon, 1979). The positive (negative) phase of the PDO is defined as years when the filtered DJF PDO index is greater than (less than) zero. We define the negative epoch (PDO−) as two negative phases of the PDO that occurred from 1948 to 1977 and 1989 to 2014, and the positive epoch (PDO+) is defined as a positive phase of the PDO that occurred from 1978 to 1988 (Fig. 1b). Also, the detrended variables are used to conduct correlation and composite analyses. Student's and Welch's t tests are used in the study to determine the statistical significance of correlation and composite analyses, respectively.
2.2.2 Wavelet analysis
The PyCWT library (https://pycwt.readthedocs.io/en/latest/tutorial/cwt/, last access: 10 February 2025) is used to calculate the cross-wavelet power spectrum. This library is based on the implementation by Torrence and Compo (1998). We employed the cross-wavelet transform to calculate the wavelet spectrum between monthly time series of the PDO index and the area-averaged monthly ERA5 snowfall over the KH region. The cross-wavelet transform finds regions in time–frequency space where the time series show high common power.
3.1 PDO and KH winter snowfall
This study aims to examine the long-term variability in DJF snowfall in the KH region in relation to the PDO from 1940 to 2022. There is a significant negative correlation between the low-pass-filtered and detrended time series of the DJF PDO and DJF snowfall in the KH (Fig. 1b), with a coefficient of −0.51. However, the PDO is not a single phenomenon but rather a set of processes that occur in both the tropics and the extratropics and reflects the influence of various processes occurring at distinct timescales (Newman et al., 2016). More precisely, elevated sea surface temperature (SST) in the eastern tropical Pacific is linked to lower SST in the central and western North Pacific, while higher SST is observed in the eastern North Pacific (Deser et al., 2004; Newman et al., 2016). Thus, decadal variability in the North Pacific SSTs is linked to tropical Pacific decadal variability, specifically in terms of the long-lasting seasonal ENSO patterns (Newman et al., 2011; Wittenberg et al., 2014) as well as the ENSO-like multidecadal oscillation (i.e. IPO; Zhang et al., 1997). Occasionally, the AMO may also influence multidecadal variability of the PDO (Zhang and Delworth, 2007). After excluding of the influences of the ENSO and the IPO, the correlation slightly increases to −0.53 and −0.54 and rises to −0.67 upon the elimination of the AMO's impact.
The spatial distribution of the correlation between the PDO and KH snowfall in winter (Fig. 2a) is significantly negative along the Western and Central Himalayas and much of the southern Karakoram but positive over the Tibetan Plateau and northern India (not shown). The snowfall in the KH region during the boreal autumn (SON) and spring (MAM) has a strong positive correlation with the PDO (not shown), whereas the summer monsoon season (JJA) displays a weak but positive correlation with the PDO. The different signs of the correlation suggest that the dynamic processes driving KH snowfall either vary by season or the seasonal influence of the PDO on KH snowfall changes.
Figure 2b displays the regional distribution of the difference in detrended DJF snowfall between the negative and positive phases of the PDO, hereafter referred to as the PDO− and the PDO+, respectively. The difference is significantly positive in the KH area, particularly over the southern part of the Karakoram region. DJF snowfall in the KH accounts for around 80 %–90 % of total annual snowfall during the time period (not shown). During the PDO+, DJF snowfall over the KH is nearly 7 % lower than the average seasonal snowfall, while during the PDO− it is about 6 % higher. This indicates that the difference in DJF snowfall in the KH varies significantly depending on the phase of the PDO across several decades.
This strong relationship between the PDO and snowfall in the KH is also demonstrated through a cross-wavelet frequency spectrum analysis between the unfiltered monthly time series of the PDO index and snowfall over the KH from 1940 to 2022 (Fig. 2c). The band of strong and significant power in the period of ∼1 year in the cross-wavelet indicates that the PDO and KH snowfall both have strong interannual variability. The well-known influence of the ENSO on snowfall in the region (operating on interannual timescales) during DJF is also slightly modulated by the low-frequency oscillation of the PDO. Another band of significant power exists between 6 and 15 years, demonstrating a strong decadal-scale correlation between these two time series. The significant power in the 6–15-year range occurred between 1940 and 1970 and again from 1998 to 2015, coinciding with the negative phases of the PDO. An insignificant weak power appeared within the same range from 1971 to 1988, coinciding with the positive phase of the PDO. A long band of strong power exists throughout the 16–20-year range, observed from 1950 to 1990, while a weaker power is shown from 2000 to 2022. This indicates that the low-frequency variability of KH snowfall is influenced by decadal oscillations over various timescales, while the interdecadal variability of KH snowfall is found to influenced by the phase of the PDO.
3.2 Sea surface temperature (SST) variability during DJF
Figure 3a illustrates the well-known positive (or warm) phase of the PDO over the North Pacific, shown as a correlation between low-pass-filtered and detrended sea surface temperature (SST) and the PDO index during DJF. The correlation pattern also reveals a strong El Niño-like pattern in the eastern equatorial and tropical Pacific Ocean. For comparison, the correlation pattern between the DJF SST anomalies and the DJF snowfall anomalies in the KH region is shown in Fig. 3b. This correlation strongly resembles the negative (or cool) phase of the PDO over the North Pacific Ocean. It is characterized by positive SST anomalies in the northwestern Pacific and negative SST anomalies in the northeastern Pacific. Additionally, there are negative SST correlations in the eastern tropical Pacific region and eastern Indian Ocean adjacent to Western Australia, while positive correlations are observed in the southwestern Indian Ocean and across the northwestern Atlantic Ocean. The correlation pattern in the southern Indian Ocean reveals the subtropical Indian Ocean Dipole signature (positive phase) (Behera and Yamagata, 2001; Yamagami and Tozuka, 2015).
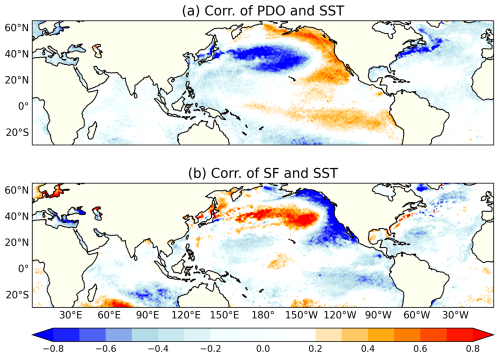
Figure 3Spatial map of correlation of the 9-year filtered (a) DJF PDO index and (b) area-averaged DJF snowfall over the KH region (as defined in Fig. 2) with 9-year filtered DJF sea surface temperature from 1940 to 2022. The correlation patterns are statistically significant at the 95 % confidence level, as determined by the two-tailed Student's t test.
3.3 Upper-atmosphere circulation response with the PDO and snowfall
To understand the anomalous atmospheric circulations that connect the PDO with anomalous DJF snowfall in the KH region, we computed the correlation of 200 hPa geopotential height with both the DJF PDO index (Fig. 4a) and DJF snowfall (Fig. 4b). The correlation pattern between the PDO and upper-level geopotential height shows a prominent upper-level trough over eastern China, Japan, and the northwestern Pacific, which is known as the East Asian trough (EAT; Qin et al., 2018; Yin and Zhang, 2021). In contrast, the correlation pattern over the Caspian Sea, the KH, and the Lake Baikal region is associated with positive geopotential height anomalies. The EAT is a well-known upper-atmospheric response of the positive phase of the PDO to the East Asia–North Pacific region during the Northern Hemisphere winter (Newman et al, 2016; Qin et al., 2018; Yin and Zhang, 2021). The intensity of the EAT is strongly linked to the strength of the winter monsoon in East Asia, and the tilt in the EAT axis is connected to midlatitude baroclinic processes, such as the eddy-driven jet or WD tracks over the East Asia–North Pacific region (Wang et al., 2009). Therefore, changes in location and intensity of the EAT can lead to or otherwise indicate regional climate anomalies, such as temperature in the upper troposphere, which subsequently influence DJF precipitation in East Asia as well as the KH during the positive phase of the PDO.
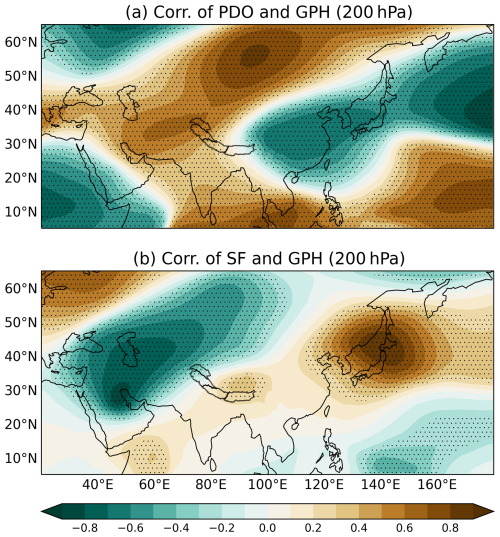
Figure 4Spatial map of correlation of the 9-year filtered (a) DJF PDO index and (b) area-averaged DJF snowfall (mm) over the KH region (as defined in Fig. 2) with 9-year filtered DJF geopotential height at 200 hPa (m) from 1940 to 2022. Stippling in (a) and (b) indicate where the correlations are significant at a 95 % confidence level, as determined by the two-tailed Student's t test.
These patterns change sign during negative phases of the PDO, when KH snowfall is enhanced, implying an anomalous upper-level trough to the west of the Karakoram, consistent with increased WD frequency or intensity. The correlation between upper-level geopotential height and snowfall has a similar pattern to the correlation between PDO and geopotential but, as expected, with reversed sign. The correlation pattern exhibits a strong ridge (or a weakened EAT) over the northwestern Pacific and Japan characterized by the significant positive geopotential height anomalies. The negative correlation to the west of the KH area shows a trough, which is stronger than the positive correlation between PDO and geopotential height in Fig. 4a, indicating the linkage of seasonal snowfall to the passage of WDs over the KH. The appearance of the anomalous trough in both pairs of correlations implies that the PDO may affect KH snowfall by somehow modulating WD activity. Therefore, it is essential to understand how decadal fluctuations in DJF snowfall in the KH are driven by WDs and how the PDO influences WD behaviour. This can be accomplished by investigating the DJF STJ, followed by a detailed investigation of the WDs.
3.4 Modulation of WDs and the subtropical westerly jet by the PDO
To further illustrate the above relationship between the PDO and DJF snowfall in the KH, we examine the composite differences in 200 hPa wind, geopotential height, and temperature (Fig. 5) between the PDO− and the PDO+. Figure 5a displays the difference in 200 hPa circulation over East Asia, the Arabian Peninsula, and the northwestern Pacific region. During the PDO−, there is a large negative geopotential height anomaly to the north of the KH region, which extends from the Caspian Sea–Arabian Peninsula to the KH. Strong westerlies are observed to the south of this trough with a stronger STJ prevailing across the KH during the PDO−. An anomalous trough in the upper atmosphere is indicative of increased WD frequency (or intensity), and the frequency of WDs is strongly affected by variations in both the latitude and intensity of the STJ (Dimri et al., 2015; Hunt et al., 2017, 2018) over South Asia. Therefore, we now focus on understanding the relationship between the PDO and the STJ.
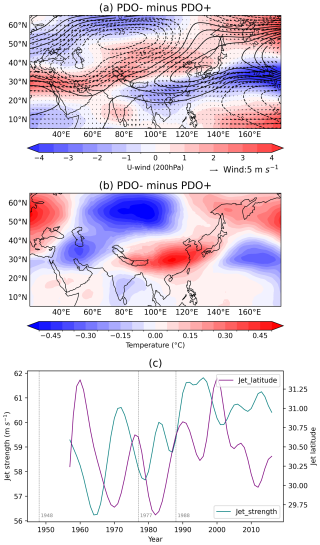
Figure 5Composite difference of (a) U wind (colours; m s−1), wind (vectors; m s−1), and geopotential height (contours; m). (b) Vertically averaged temperature (°C) from 300 to 500 hPa level during DJF between negative and positive epoch of the PDO. (c) Time series of 9-year filtered strength (magenta; m s−1) and latitude (blue) of the DJF subtropical westerly jet over the KH region from 1940 to 2022.
Upper-level jets are thermal wind responses to upper-level meridional temperature gradients. In Fig. 5b, we show the difference in mid- to upper-tropospheric (from 500 to 300 hPa) temperature between the PDO− and the PDO+. A quadrupole in the upper-air temperature gradient is present across the KH, the Tibetan Plateau (TP), and the northwestern Pacific region during the PDO−. Over the Pacific, this is effectively a direct response to the anomalous surface heating provided by the PDO. Anomalously warm SSTs over the northwestern Pacific lead to adiabatic cooling near the tropopause, which results in deep convection over the Maritime Continent during the PDO− (e.g. Wang et al., 2016). Upstream, over continental Asia, the relationship is more complicated and is probably a wave response to the direct forcing over the ocean. Therefore, a strongly enhanced meridional temperature gradient over the KH and the TP leads to a stronger and more meridionally locked STJ.
Figure 5c displays the low-pass-filtered time series of latitude and strength of the DJF STJ. During the PDO−, the STJ tends to sit slightly further north but is also substantially stronger. The correlation of the time series of the strength of the DJF STJ with the DJF PDO is significantly negative (−0.22), and the correlation between the DJF STJ strength and DJF snowfall in the KH is strongly positive (0.51). The positive (negative) phase of the PDO enhances the movement of the STJ towards the south (north) through a response to the decreased (increased) SST over the northwestern Pacific and modulates the cyclonic (anticyclonic) circulation over the northwestern Pacific and the adjacent Maritime Continent (Matsumura et al., 2016). During the PDO−, we observed a quadrupole in the anomalous upper-level temperature gradient (Fig. 5b), resulting in a negative anomaly in the temperature gradient and an anticyclonic circulation (Fig. 5a) over the TP. Thus, by modulating the STJ, the negative phase of the PDO leads to more frequent (more intense) WDs at slightly higher latitudes than usual (e.g. into the Karakoram, where the signal is the strongest).
The presence of a stronger STJ along with a wave-like pattern of trough (anomalous cyclone) over the northern region of the KH, as well as a ridge (anomalous TP anticyclone) in the upper atmosphere, increases the occurrence of WDs over the KH during the PDO−. After examining the impact of the PDO on the STJ, we now quantify its influence on WDs directly. Maps of the difference in the frequency of DJF WDs between the PDO− and the PDO+ (Fig. 6a) indicate that WDs are more frequent (with a 9 % higher frequency) over the KH region during the PDO− compared to the PDO+. Also, the frequency of WDs is found to be reduced by around 3 % in both the northern and southern regions of the KH during the PDO− compared to the PDO+. These WDs are observed to be more intense in the vicinity of the Caspian Sea and north of the KH during the PDO− rather than the PDO+ (not shown).
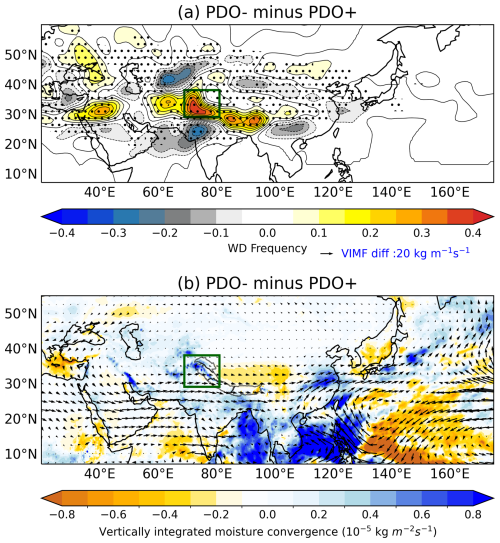
Figure 6Composite difference of (a) WD frequency and (b) vertically integrated moisture flux (vectors; kg m−1 s−1) and vertically integrated moisture convergence (colours; kg m−2 s−1) during DJF between the negative and positive epochs of the PDO from 1940 to 2022. Stippling in (a) indicates where the differences are significant at a 95 % confidence level, as determined by the two-tailed Welch's t test. Green box in (a) and (b) highlights the KH region (as defined in Fig. 2).
3.5 Atmospheric–ocean response of the PDO to moisture transport in the KH
Increased frequency and intensity of WDs have a significant impact on precipitation in the KH and the surrounding region because they govern southwesterly moisture transport from the Arabian Sea (Baudouin et al., 2021; Hunt and Dimri 2021). The composite difference of DJF VIMF and VIMFC between the PDO− and the PDO+ is now examined to determine the response of moisture transport to the PDO and its subsequent effect on the KH (Fig. 6b). The average difference in VIMFC between the PDO− and the PDO+ is about kg m−2 s−1 within the KH region. An advection of moisture from the Black Sea, the Red Sea, and the eastern Mediterranean Sea through the Arabian Peninsula/Arabian Sea towards the KH in a westerly fashion is observed. The precipitation associated with WDs is mostly determined by their intensity and proximity to the Arabian Sea (Baudouin et al., 2021; Bharati et al., 2025). The variations in the moisture transport across the Arabian Peninsula/Arabian Sea are not directly linked to changes in VIMF over the northwestern Pacific, but the presence of more WDs south of the strong DJF STJ over the KH clearly results in greater moisture transport towards the KH during the PDO−. Hence, the anomalous moisture transport nearly perpendicular to the KH results in an increased moisture flux convergence about 16 % greater during the PDO− compared to the PDO+ and leads to greater precipitation in the region during the PDO−.
The recent impacts of climate change over the KH, particularly in mean and extreme winter precipitation, have been largely attributed to anthropogenic forcing, such as greenhouse gases, aerosols, and changes in land use. However, these changes cannot be solely explained by natural forcing (Krishnan et al., 2019). Oceanic conditions, especially changes in SSTs over the equatorial–tropical Pacific and North Pacific, play an important role in driving interdecadal variability in atmospheric circulation and hence winter precipitation over the KH.
DJF snowfall in the KH accounts for around 80 %–90 % of total annual snowfall during the time period. Hence, a 15 % difference in DJF snowfall can have a significant influence on agriculture in this region, especially since most of the rivers in this region, such as tributaries of the Indus, the Tarim, and the Ganges, are partially fed by snowmelt in the spring and later seasons (Armstrong et al., 2019). Understanding the interdecadal variability and its relationship to the PDO is important for understanding the long-term climate of the KH. We have analysed the long-term variability in winter snowfall over the KH due to the PDO by using ERA5 reanalysis data from 1940 to 2022. We found that a strong negative correlation of −0.51 between the PDO and DJF snowfall in the KH. Mean KH snowfall during DJF is approximately 6 % greater than the DJF seasonal average during the PDO− and 7 % lower during the PDO+.
The PDO-associated anomalous warming of SST in the northwestern Pacific modulates the snowfall in the KH via changes in upper-level temperatures over the Pacific and Asia. The warm SSTs lead to increased deep convection and subsequent upper-tropospheric adiabatic cooling over the Pacific. During the PDO−, the anomalous heating of the tropospheric column over North Pacific leads to a wave-like pattern with an upper-level trough over the north of the KH and an upper-level ridge over the Tibetan Plateau. This results in a stronger STJ to the west of and over the KH, before it is deflected northwards over the Tibetan Plateau. There is a strong positive correlation between the strength of the DJF STJ and DJF snowfall in the KH, with a correlation coefficient of 0.51, and a significant negative correlation between the strength of the STJ and the PDO, with a correlation coefficient of −0.22 during DJF at decadal scale. These results indicate a wave response over the KH to the direct forcing of the North Pacific Ocean.
These anomalous jet conditions over the KH are linked to a higher occurrence of WDs across the region. Using a track catalogue, we found that WDs are 9 % more frequent across the KH and drop by approximately 3 % in both the northern and southern regions of the KH during the PDO− compared to the PDO+. However, the WDs are found to be more intense in the vicinity of the Caspian Sea and north of the KH during the PDO− rather than the PDO+, which is not shown in this study. This increase in WD frequency results in anomalous moisture transport from the Arabian Sea, the Black Sea, the Red Sea, and the eastern Mediterranean Sea towards the KH. The moisture transport is almost perpendicular to the orography of the KH, leading to a strong moisture convergence about 16 % greater during the PDO− compared to the PDO+ and thus increased DJF precipitation in the region during the negative phases of the PDO.
Our findings highlight the importance of considering interdecadal variability when trying to quantify the effects of anthropogenic climate change in the KH. The negative phase of PDO has led to increased WD activity and hence increased winter snowfall over this region, and it may be masking the effects of climate change. More research is needed to disentangle climate change from the effects of interdecadal variability over this vulnerable region so that policymakers can be better informed. There are still some uncertainties in the amount of snowfall and precipitation in the KH region, and the short time series available from reanalysis that can be used for studying decadal oscillations is not enough to support such studies. Future long-term climate simulations could be used for subsequent work if the models accurately represent the interaction between the PDO and snowfall (or precipitation) in this region.
The analysis and visualizations presented in this study were performed using Python libraries. The custom scripts and algorithms developed for this research can be accessed by contacting the corresponding author via email.
The precipitation and other meteorological datasets used in this study are publicly available and can be accessed through the URLs provided in the Data (Sect. 2.1) and References sections (Hunt et al., 2018; Nischal et al., 2022).
PB: conceptualization, formal analysis, methodology, investigation, software, visualization, writing (original draft). KMRH: conceptualization, methodology, software, writing (review and editing). PD: supervision, conceptualization, writing (review and editing).
The contact author has declared that none of the authors has any competing interests.
Publisher's note: Copernicus Publications remains neutral with regard to jurisdictional claims made in the text, published maps, institutional affiliations, or any other geographical representation in this paper. While Copernicus Publications makes every effort to include appropriate place names, the final responsibility lies with the authors.
The work was carried out at CORAL, Indian Institute of Technology Kharagpur, under the supervision of Pranab Deb. Priya Bharati is funded by the Ministry of Science and Technology, Government of India, Council of Scientific and Industrial Research (CSIR; 09/081(1371)/2019-EMR-I). Kieran Mark Rainwater Hunt is supported by a NERC independent research fellowship (MITRE; NE/W007924/1).
This research has been supported by the Ministry of Science and Technology, Government of India, Council of Scientific and Industrial Research (CSIR; 09/081(1371)/2019-EMR-I) at IIT Kharagpur.
This paper was edited by Juliane Schwendike and reviewed by two anonymous referees.
Adler, R., Sapiano, M., Huffman, G., Bolvin, D., Gu, G., Wang, J., and Becker, A.: The new version 2.3 of the Global Precipitation Climatology Project (GPCP) monthly analysis product, University of Maryland, 1072–1084, https://psl.noaa.gov/thredds/catalog/Datasets/gpcp/catalog.html?dataset=Datasets/gpcp/precip.mon.mean.nc (last access: 10 February 2024), 2016.
Aggarwal, D., Chakraborty R., and Attada, R.: Investigating bi-decadal precipitation changes over the Northwest Himalayas during the pre-monsoon: role of Pacific decadal oscillations, Clim. Dynam., 62, 1203–1218, https://doi.org/10.1007/s00382-023-06969-3, 2024.
Anders, A. M., Roe, G. H., Hallet, B., Montgomery, D. R., Finnegan, N. J., and Putkonen, J.: Spatial patterns of precipitation and topography in the Himalaya, Geological Society of America, https://doi.org/10.1130/2006.2398(03), 2006.
Archer, D. R. and Fowler, H. J.: Spatial and temporal variations in precipitation in the Upper Indus Basin, global teleconnections and hydrological implications, Hydrol. Earth Syst. Sci., 8, 47–61, https://doi.org/10.5194/hess-8-47-2004, 2004.
Armstrong, R. L., Rittger, K., Brodzik, M. J., Racoviteanu, A., Barrett, A. P., Khalsa, S. J. S., and Armstrong, B.: Runoff from glacier ice and seasonal snow in High Asia: separating melt water sources in river flow, Reg. Environ. Change, 19, 1249–1261, https://doi.org/10.1007/s10113-018-1429-0, 2019.
Barlow, M., Wheeler, M., Lyon, B., and Cullen, H.: Modulation of daily precipitation over southwest Asia by the Madden–Julian oscillation, Mon. Weather Rev., 133, 3579–3594, https://doi.org/10.1175/MWR3026.1, 2005.
Barros, A. P., Chiao, S., Lang, T. J., Burbank, D., and Putkonen, J.: From weather to climate Seasonal and interannual variability of storms and implications for erosion processes in the Himalaya, Geological Society of America, https://doi.org/10.1130/S2006.2398(02), 2006.
Basu, S., Bieniek, P. A., and Deoras, A.: An investigation of reduced western disturbance activity over Northwest India in November–December 2015 compared to 2014 – A case study, Asia-Pacif. J. Atmos. Sci., 53, 75–83, https://doi.org/10.1007/s13143-017-0006-7, 2017.
Baudouin, J. P., Herzog, M., and Petrie, C. A.: Cross-validating precipitation datasets in the Indus River basin, Hydrol. Earth Syst. Sci., 24, 427–450, https://doi.org/10.5194/hess-24-427-2020, 2020.
Baudouin, J. P., Herzog, M., and Petrie, C. A.: Synoptic processes of winter precipitation in the Upper Indus Basin, Weather Clim. Dynam., 2, 1187–1207, https://doi.org/10.5194/wcd-2-1187-2021, 2021.
Beck, H. E., Pan, M., Roy, T., Weedon, G. P., Pappenberger, F., van Dijk, A. I. J. M., Huffman, G. J., Adler, R. F., and Wood, E. F.: Daily evaluation of 26 precipitation datasets using Stage-IV gauge-radar data for the CONUS, Hydrol. Earth Syst. Sci., 23, 207–224, https://doi.org/10.5194/hess-23-207-2019, 2019.
Behera, S. K. and Yamagata, T.: Subtropical SST dipole events in the southern Indian Ocean, Geophys. Res. Lett., 28, 327–330, https://doi.org/10.1029/2000GL011451, 2001.
Bharati, P., Deb, P., Hunt, K. M., Orr, A., and Dash, M. K.: ENSO-induced latitudinal variation of the subtropical jet modulates extreme winter precipitation over the Western Himalaya, Adv. Atmos. Sci., https://doi.org/10.1007/s00376-024-4057-2, in press, 2025.
Bolch, T., Kulkarni, A., Kääb, A., Huggel, C., Paul, F., Cogley, J. G., and Stoffel, M.: The state and fate of Himalayan glaciers, Science, 336, 310–314, https://doi.org/10.1126/science.1215828, 2012.
Bookhagen, B. and Burbank, D. W.: Toward a complete Himalayan hydrological budget: Spatiotemporal distribution of snowmelt and rainfall and their impact on river discharge, J. Geophys. Res.-Earth, 115, F03019, https://doi.org/10.1029/2009JF001426, 2010.
Bosilovich, M. G., Chen, J., Robertson, F. R., and Adler, R. F.: Evaluation of global precipitation in reanalyses, J. Appl. Meteorol. Clim., 47, 2279–2299, https://doi.org/10.1175/2008JAMC1921.1, 2008.
Cannon, F., Carvalho, L. M., Jones, C., and Bookhagen, B.: Multi-annual variations in winter westerly disturbance activity affecting the Himalaya, Clim. Dynam., 44, 441–455, https://doi.org/10.1007/s00382-014-2248-8, 2015.
Cannon, F., Carvalho, L. M., Jones, C., Hoell, A., Norris, J., Kiladis, G. N., and Tahir, A. A.: The influence of tropical forcing on extreme winter precipitation in the western Himalaya, Clim. Dynam., 48, 1213–1232, https://doi.org/10.1007/s00382-016-3137-0, 2017.
Dahri, Z. H., Moors, E., Ludwig, F., Ahmad, S., Khan, A., Ali, I., and Kabat, P.: Adjustment of measurement errors to reconcile precipitation distribution in the high-altitude Indus basin, Int. J. Climatol., 38, 3842–3860, https://doi.org/10.1002/joc.5539, 2018.
Dai, A.: The influence of the inter-decadal Pacific oscillation on US precipitation during 1923–2010, Clim. Dynam., 41, 633–646, https://doi.org/10.1007/s00382-012-1446-5, 2013.
de Kok, R. J., Tuinenburg, O. A., Bonekamp, P. N., and Immerzeel, W. W.: Irrigation as a potential driver for anomalous glacier behavior in High Mountain Asia, Geophys. Res. Lett., 45, 2047–2054, https://doi.org/10.1002/2017GL076158, 2018.
Deser, C., Phillips, A. S., and Hurrell, J. W.: Pacific interdecadal climate variability: Linkages between the tropics and the North Pacific during boreal winter since 1900, J. Climate, 17, 3109–3124, https://doi.org/10.1175/1520-0442(2004)017<3109:PICVLB>2.0.CO;2, 2004.
Dettinger, M. D., Cayan, D. R., Diaz, H. F., and Meko, D. M.: North–south precipitation patterns in western North America on interannual-to-decadal timescales, J. Climate, 11, 3095–3111, https://doi.org/10.1175/1520-0442(1998)011<3095:NSPPIW>2.0.CO;2, 1998.
Dimri, A. P.: Relationship between ENSO phases with Northwest India winter precipitation, Int. J. Climatol., 33, 1917–1923, https://doi.org/10.1002/joc.3559, 2013.
Dimri, A. P. and Dash, S. K.: Wintertime climatic trends in the western Himalayas, Climatic Change, 111, 775–800, https://doi.org/10.1007/s10584-011-0201-y, 2012.
Dimri, A. P., Niyogi, D., Barros, A. P., Ridley, J., Mohanty, U. C., Yasunari, T., and Sikka, D. R.: Western disturbances: a review, Rev. Geophys., 53, 225–246, https://doi.org/10.1002/2014RG000460, 2015.
Dollan, I. J., Maina, F. Z., Kumar, S. V., Nikolopoulos, E. I., and Maggioni, V.: An assessment of gridded precipitation products over High Mountain Asia, J. Hydrol.: Reg. Stud., 52, 101675, https://doi.org/10.1016/j.ejrh.2024.101675, 2024.
Dong, B. and Dai, A.: The influence of the interdecadal Pacific oscillation on temperature and precipitation over the globe, Clim. Dynam., 45, 2667–2681, https://doi.org/10.1007/s00382-015-2500-x, 2015.
Duchon, C. E.: Lanczos filtering in one and two dimensions, J. Appl. Meteorol. Clim., 18, 1016-1022, https://doi.org/10.1175/1520-0450(1979)018<1016:LFIOAT>2.0.CO;2, 1979.
Enfield, D. B., Mestas-Nuñez, A. M., and Trimble, P. J.: The Atlantic multidecadal oscillation and its relation to rainfall and river flows in the continental US, Geophys. Res. Lett., 28, 2077–2080, https://doi.org/10.1029/2000GL012745, 2001.
Farinotti, D., Immerzeel, W. W., de Kok, R. J., Quincey, D. J., and Dehecq, A.: Manifestations and mechanisms of the Karakoram glacier Anomaly, Nat. Geosci., 13, 8–16, https://doi.org/10.1038/s41561-019-0513-5, 2020.
Filippi, L., Palazzi, E., von Hardenberg, J., and Provenzale, A.: Multidecadal variations in the relationship between the NAO and winter precipitation in the Hindu Kush–Karakoram, J. Climate, 27, 7890–7902, https://doi.org/10.1175/JCLI-D-14-00286.1, 2014.
Forsythe, N., Fowler, H. J., Li, X. F., Blenkinsop, S., and Pritchard, D.: Karakoram temperature and glacial melt driven by regional atmospheric circulation variability, Nat. Clim. Change, 7, 664–670, https://doi.org/10.1038/NCLIMATE3361, 2017.
Gardelle, J., Berthier, E., and Arnaud, Y.: Slight mass gain of Karakoram glaciers in the early twenty-first century, Nat. Geosci., 5, 322–325, https://doi.org/10.1038/NGEO1450, 2012.
Gelaro, R., McCarty, W., Suárez, M. J., Todling, R., Molod, A., Takacs, L., and Zhao, B.: The modern-era retrospective analysis for research and applications, version 2 (MERRA-2), J. Climate, 30, 5419–5454, https://doi.org/10.1175/JCLI-D-16-0758.1, 2017.
Harris, I. P. D. J., Jones, P., Osborn, T., and Lister, D.: Updated high-resolution grids of monthly climatic observations-the CRU TS3.10 Dataset, Int. J. Climatol., 34, 623–642, 2014.
Hersbach, H., de Rosnay, P., Bell, B., Schepers, D., Simmons, A., Soci, C., and Berrisford, P.: Operational global reanalysis: Progress, future directions and synergies with NWP, ERA report Series No. 27, European Centre for Medium Range Weather Forecasts, Reading, UK, https://cds.climate.copernicus.eu/datasets/reanalysis-era5-land-monthly-means?tab=download (last access: 10 February 2025), 2018.
Hewitt, K.: The Karakoram anomaly? Glacier expansion and the `elevation effect', Karakoram Himalaya, Mt. Res. Dev., 25, 332–340, https://doi.org/10.1659/0276-4741(2005)025[0332:TKAGEA]2.0.CO;2, 2005.
Hewitt, K..: Glaciers of the Karakoram Himalaya, in: Encyclopedia of Snow, Ice and Glaciers, Vol. 25, edited by: Singh, V. P., Singh, P., and Haritashya, U. K., Springer Netherlands, Dordrecht, 429–436, https://doi.org/10.1007/978-94-007-6311-1, 2014.
Hoell, A., Barlow, M., and Saini, R.: Intraseasonal and seasonal-to-interannual Indian Ocean convection and hemispheric teleconnections, J. Climate, 26, 8850–8867, https://doi.org/10.1175/JCLI-D-12-00306.1, 2013.
Hu, X. and Yuan, W.: Evaluation of ERA5 precipitation over the eastern periphery of the Tibetan plateau from the perspective of regional rainfall events, Int. J. Climatol., 41, 2625–2637, https://doi.org/10.1002/joc.6980, 2021.
Huffman, G. J., Bolvin, D. T., Nelkin, E. J., Wolff, D. B., Adler, R. F., Gu, G., and Stocker, E. F.: The TRMM multisatellite precipitation analysis (TMPA): Quasi-global, multiyear, combined-sensor precipitation estimates at fine scales, J. Hydrometeorol., 8, 38–55, https://doi.org/10.1175/JHM560.1, 2007.
Huffman, G. J., Bolvin, D. T., Braithwaite, D., Hsu, K., Joyce, R., Xie, P., and Yoo, S. H.: NASA global precipitation measurement (GPM) integrated multi-satellite retrievals for GPM (IMERG), Algorithm theoretical basis document (ATBD) version 4, 2020-05, https://disc.gsfc.nasa.gov/datasets?keywords=GPM_IMERGV07&page=1 (last access: 10 February 2025), 2015.
Hunt, K. M. and Zaz, S. N.: Linking the North Atlantic Oscillation to winter precipitation over the Western Himalaya through disturbances of the subtropical jet, Clim. Dynam., 60, 2389–2403, https://doi.org/10.1007/s00382-022-06450-7, 2023.
Hunt, K. M., Turner, A. G., and Shaffrey, L. C.: The evolution, seasonality and impacts of western disturbances, Q. J. Roy. Meteorol. Soc., 144, 278–290, https://doi.org/10.1002/qj.3200, 2017.
Hunt, K. M. R., Curio, J., Turner, A. G., and Schiemann, R.: Subtropical westerly jet influence on occurrence of western disturbances and Tibetan Plateau vortices, Geophys. Res. Lett., 45, 8629–8636, https://doi.org/10.1029/2018GL077734, 2018.
Hunt, K. M. R., Baudouin, J.-P., Turner, A. G., Dimri, A. P., Jeelani, G., Pooja, Chattopadhyay, R., Cannon, F., Arulalan, T., Shekhar, M. S., Sabin, T. P., and Palazzi, E.: Western disturbances and climate variability: a review of recent developments, EGUsphere [preprint], https://doi.org/10.5194/egusphere-2024-820, 2024.
Javed, A., Kumar, P., Hodges, K. I., Sein, D. V., Dubey, A. K., and Tiwari, G.: Does the recent revival of western disturbances govern the Karakoram anomaly?, J. Climate, 35, 4383–4402, https://doi.org/10.1175/JCLI-D-21-0129.1, 2022.
Joshi, M. K., Rai, A., and Pandey, A. C.: Validation of TMPA and GPCP 1DD against the ground truth rain-gauge data for Indian region, Int. J. Climatol., 33, 2633–2648, https://doi.org/10.1002/joc.3612, 2013.
Kääb, A., Berthier, E., Nuth, C., Gardelle, J., and Arnaud, Y.: Contrasting patterns of early twenty-first-century glacier mass change in the Himalayas, Nature, 488, 495–498, https://doi.org/10.1038/nature11324, 2012.
Kamil, S., Almazroui, M., Kang, I. S., Hanif, M., Kucharski, F., Abid, M. A., and Saeed, F.: Long-term ENSO relationship to precipitation and storm frequency over western Himalaya–Karakoram–Hindukush region during the winter season, Clim. Dynam., 53, 5265–5278, https://doi.org/10.1007/s00382-019-04859-1, 2019.
Kapnick, S. B., Delworth, T. L., Ashfaq, M., Malyshev, S., and Milly, P. C.: Snowfall less sensitive to warming in Karakoram than in Himalayas due to a unique seasonal cycle, Nat. Geosci., 7, 834–840, https://doi.org/10.1038/NGEO2269, 2014.
Kar, S. C. and Rana, S.: Interannual variability of winter precipitation over northwest India and adjoining region: impact of global forcings, Theor. Appl. Climatol., 116, 609–623, https://doi.org/10.1007/s00704-013-0968-z, 2014.
Kishore, P., Jyothi, S., Basha, G., Rao, S. V. B., Rajeevan, M., Velicogna, I., and Sutterley, T. C.: Precipitation climatology over India: validation with observations and reanalysis datasets and spatial trends, Clim. Dynam., 46, 541–556, https://doi.org/10.1007/s00382-015-2597-y, 2016.
Krishnan, R. and Sugi, M.: Pacific decadal oscillation and variability of the Indian summer monsoon rainfall, Clim. Dynam., 21, 233–242, https://doi.org/10.1007/s00382-003-0330-8, 2003.
Krishnan, R., Sabin, T. P., Madhura, R. K., Vellore, R. K., Mujumdar, M., Sanjay, J., and Rajeevan, M.: Non-monsoonal precipitation response over the Western Himalayas to climate change, Clim. Dynam., 52, 4091–4109, https://doi.org/10.1007/s00382-018-4357-2, 2019.
Krishnamurthy, L. and Krishnamurthy, V.: Decadal scale oscillations and trend in the Indian monsoon rainfall, Clim. Dynam., 43, 319–331, https://doi.org/10.1007/s00382-013-1870-1, 2014a.
Krishnamurthy, L. and Krishnamurthy, V. J. C. D.: Influence of PDO on South Asian summer monsoon and monsoon–ENSO relation, Clim. Dynam., 42, 2397–2410, https://doi.org/10.1007/s00382-013-1856-z, 2014b.
Kumar, P., Saharwardi, M. S., Banerjee, A., Azam, M. F., Dubey, A. K., and Murtugudde, R.: Snowfall variability dictates glacier mass balance variability in Himalaya-Karakoram, Sci. Rep., 9, 18192, https://doi.org/10.1038/s41598-019-54553-9, 2019.
Lang, T. J. and Barros, A. P.: Winter storms in the central Himalayas, J. Meteorol. Soc. Jpn. Ser. II, 82, 829–844, https://doi.org/10.2151/jmsj.2004.829, 2004.
Mantua, N. J., Hare, S. R., and Zhang, Y.: A Pacific interdecadal climate oscillation with impacts on salmon production, Oceanogr. Literat. Rev., 1, 36, 1998.
Matsumura, S., Horinouchi, T., Sugimoto, S., and Sato, T.: Response of the Baiu rainband to northwest Pacific SST anomalies and its impact on atmospheric circulation, J. Climate, 29, 3075–3093, https://doi.org/10.1175/JCLI-D-15-0691.1, 2016.
Ménégoz, M., Gallée, H., and Jacobi, H. W.: Precipitation and snow cover in the Himalaya: from reanalysis to regional climate simulations, Hydrol. Earth Syst. Sci., 17, 3921–3936, https://doi.org/10.5194/hess-17-3921-2013, 2013.
Midhuna, T. M. and Dimri, A. P.: Impact of arctic oscillation on Indian winter monsoon, Meteorol. Atmos. Phys., 131, 1157–1167, https://doi.org/10.1007/s00703-018-0628-z, 2019.
Midhuna, T. M., Kumar, P., and Dimri, A. P.: A new Western Disturbance Index for the Indian winter monsoon, J. Earth Syst. Sci., 129, 1-14, https://doi.org/10.1007/s12040-019-1324-1, 2020.
Newman, M., Shin, S. I., and Alexander, M. A.: Natural variation in ENSO flavors, Geophys. Res. Lett., 38, L14705, https://doi.org/10.1029/2011GL047658, 2011.
Newman, M., Alexander, M. A., Ault, T. R., Cobb, K. M., Deser, C., Di Lorenzo, E., and Smith, C. A.: The Pacific decadal oscillation, revisited, J. Climate, 29, 4399–4427, https://doi.org/10.1175/JCLI-D-15-0508.1, 2016.
Nischal, Attada, R. and Hunt, K. M.: Evaluating winter precipitation over the western Himalayas in a high-resolution Indian regional reanalysis using multisource climate datasets, J. Appl. Meteorol. Clim., 61, 1613–1633, https://doi.org/10.1175/JAMC-D-21-0172.1, 2022.
Norris, J., Carvalho, L. M., Jones, C., and Cannon, F.: WRF simulations of two extreme snowfall events associated with contrasting extratropical cyclones over the western and central Himalaya, J. Geophys. Res.-Atmos., 120, 3114–3138, https://doi.org/10.1002/2014JD022592, 2015.
Norris, J., Carvalho, L. M., Jones, C., Cannon, F., Bookhagen, B., Palazzi, E., and Tahir, A. A.: The spatiotemporal variability of precipitation over the Himalaya: evaluation of one-year WRF model simulation, Clim. Dynam., 49, 2179–2204, https://doi.org/10.1007/s00382-016-3414-y, 2017.
Norris, J., Carvalho, L. M., Jones, C., and Cannon, F.: Deciphering the contrasting climatic trends between the central Himalaya and Karakoram with 36 years of WRF simulations, Clim. Dynam., 52, 159–180, https://doi.org/10.1007/s00382-018-4133-3, 2019.
Palazzi, E., Von Hardenberg, J., and Provenzale, A.: Precipitation in the Hindu-Kush Karakoram Himalaya: observations and future scenarios, J. Geophys. Res.-Atmos., 118, 85–100, https://doi.org/10.1029/2012JD018697, 2013.
Power, S., Casey, T., Folland, C., Colman, A., and Mehta, V.: Inter-decadal modulation of the impact of ENSO on Australia, Clim. Dynam., 15, 319–324, 1999.
Pritchard, H. D.: Asia's shrinking glaciers protect large populations from drought stress, Nature, 569, 649–654, https://doi.org/10.1038/s41586-019-1240-1, 2019.
Qin, M., Li, D., Dai, A., Hua, W., and Ma, H.: The influence of the Pacific Decadal Oscillation on North Central China precipitation during boreal autumn, Int. J. Climatol., 38, e821–e831, https://doi.org/10.1002/joc.5410, 2018.
Rana, S., McGregor, J., and Renwick, J.: Precipitation seasonality over the Indian subcontinent: An evaluation of gauge, reanalyses, and satellite retrievals, J. Hydrometeorol., 16, 631–651, https://doi.org/10.1175/JHM-D-14-0106.1, 2015.
Rana, S., McGregor, J., and Renwick, J.: Dominant modes of winter precipitation variability over Central Southwest Asia and inter-decadal change in the ENSO teleconnection, Clim. Dynam., 53, 5689–5707, https://doi.org/10.1007/s00382-019-04889-9, 2019.
Ridley, J., Wiltshire, A., and Mathison, C.: More frequent occurrence of westerly disturbances in Karakoram up to 2100, Sci. Total Environ., 468, S31–S35, https://doi.org/10.1016/j.scitotenv.2013.03.074, 2013.
Roy, J., Moors, E., Murthy, M. S. R., Prabhakar, S. V. R. K., Khattak, B. N., Shi, P., Huggel, C., and Chitale, V.: Exploring futures of the Hindu Kush Himalaya: scenarios and pathways. The Hindu Kush Himalaya assessment: Mountains, climate change, sustainability and people, Springer, Cham, 99–125, https://doi.org/10.1007/978-3-319-92288-1_4, 2020.
Schneider, U., Becker, A., Finger, P., Meyer-Christoffer, A., and Ziese, M.: GPCC full data monthly product version 2018 at 0.25: monthly land-surface precipitation from rain-gauges built on GTS-based and historical data, Global Precipitation Climatology Centre, https://downloads.psl.noaa.gov/Datasets/gpcc/full_v7/ (last access: 10 February 2025), 2018.
Singh, T., Saha, U., Prasad, V. S., and Gupta, M. D.: Assessment of newly-developed high resolution reanalyses (IMDAA, NGFS and ERA5) against rainfall observations for Indian region, Atmos. Res., 259, 105679, https://doi.org/10.1016/j.atmosres.2021.105679, 2021.
Strangeways, I.: A history of rain gauges, Weather, 65, 133–138, https://doi.org/10.1002/wea.548, 2010.
Syed, F. S., Giorgi, F., Pal, J. S., and Keay, K.: Regional climate model simulation of winter climate over Central-Southwest Asia, with emphasis on NAO and ENSO effects, Int. J. Climatol., 30, 220–235, https://doi.org/10.1002/joc.1887, 2010.
Tahir, A. A., Chevallier, P., Arnaud, Y., and Ahmad, B.: Snow cover dynamics and hydrological regime of the Hunza River basin, Karakoram Range, Northern Pakistan, Hydrol. Earth Syst. Sci., 15, 2275–2290, https://doi.org/10.5194/hess-15-2275-2011, 2011.
Torrence, C. and Compo, G. P.: A practical guide to wavelet analysis, B. Am. Meteorol. Soc., 79, 61–78, https://doi.org/10.1175/1520-0477(1998)079<0061:APGTWA>2.0.CO;2, 1998.
Wang, L., Chen, W., Zhou, W., and Huang, R.: Interannual variations of East Asian trough axis at 500 hPa and its association with the East Asian winter monsoon pathway, J. Climate, 22, 600–614, https://doi.org/10.1175/2008JCLI2295.1, 2009.
Wang, S., Huang, J., He, Y., and Guan, Y.: Combined effects of the Pacific decadal oscillation and El Nino-southern oscillation on global land dry–wet changes, Sci. Rep., 4, 6651, https://doi.org/10.1038/srep06651, 2014.
Wang, W., Matthes, K., Omrani, N. E., and Latif, M.: Decadal variability of tropical tropopause temperature and its relationship to the Pacific Decadal Oscillation, Sci. Rep., 6, 29537, https://doi.org/10.1038/srep29537, 2016.
Wang, X., Tolksdorf, V., Otto, M., and Scherer, D.: High Asia Refined Analysis Version 2 (HAR v2): a New Atmospheric Data Set for the Third Pole Region, EGU General Assembly 2020, Online, 4–8 May 2020, p. 8756, EGU2020-8756, https://doi.org/10.5194/egusphere-egu2020-8756, 2020.
Wittenberg, A. T., Rosati, A., Delworth, T. L., Vecchi, G. A., and Zeng, F.: ENSO modulation: Is it decadally predictable?, J. Climate, 27, 2667–2681, https://doi.org/10.1175/JCLI-D-13-00577.1, 2014.
Wu, B. and Wang, J.: Winter Arctic oscillation, Siberian high and East Asian winter monsoon, Geophys. Res. Lett., 29, 1897, https://doi.org/10.1029/2002GL015373, 2002.
Wu, X. and Mao, J.: Interdecadal modulation of ENSO-related spring rainfall over South China by the Pacific Decadal Oscillation, Clim. Dynam., 47, 3203–3220, https://doi.org/10.1007/s00382-016-3021-y, 2016.
Xie, P. and Arkin, P. A.: Global precipitation: A 17-year monthly analysis based on gauge observations, satellite estimates, and numerical model outputs, B. Am. Meteorol. Soc., 78, 2539–2558, https://doi.org/10.1175/1520-0477(1997)078<2539:GPAYMA>2.0.CO;2, 1997.
Yadav, R. K., Rupa Kumar, K., and Rajeevan, M.: Role of Indian Ocean sea surface temperatures in modulating northwest Indian winter precipitation variability, Theor. Appl. Climatol., 87, 73–83, https://doi.org/10.1007/s00704-005-0221-5, 2007.
Yadav, R. K., Rupa Kumar, K., and Rajeevan, M.: Increasing influence of ENSO and decreasing influence of AO/NAO in the recent decades over northwest India winter precipitation, J. Geophys. Res.-Atmos., 114, D12112, https://doi.org/10.1029/2008JD011318, 2009.
Yadav, R. K., Yoo, J. H., Kucharski, F., and Abid, M. A.: Why is ENSO influencing northwest India winter precipitation in recent decades?, J. Climate, 23, 1979–1993, https://doi.org/10.1175/2009JCLI3202.1, 2010.
Yamagami, Y. and Tozuka, T.: Interdecadal changes of the Indian Ocean subtropical dipole mode, Clim. Dynam., 44, 3057–3066, https://doi.org/10.1007/s00382-014-2202-9, 2015.
Yang, Q., Ma, Z., and Xu, B.: Modulation of monthly precipitation patterns over East China by the Pacific Decadal Oscillation, Climatic Change, 144, 405–417, https://doi.org/10.1007/s10584-016-1662-9, 2017.
Yatagai, A., Kamiguchi, K., Arakawa, O., Hamada, A., Yasutomi, N., and Kitoh, A.: APHRODITE; Constructing a long-term daily gridded precipitation dataset for Asia based on a dense network of rain gauges, B. Am. Metorol. Soc., 93, 1401–1415, https://doi.org/10.1175/BAMS-D-11-00122.1, 2012.
Yin, J. and Zhang, Y.: Decadal changes of East Asian jet streams and their relationship with the mid-high latitude circulations, Clim. Dynam., 56, 2801–2821, https://doi.org/10.1007/s00382-020-05613-8, 2021.
Zhang, R. and Delworth, T. L.: Impact of the Atlantic multidecadal oscillation on North Pacific climate variability, Geophys. Res. Lett., 34, L23708, https://doi.org/10.1029/2007GL031601, 2007.
Zhang, Y., Wallace, J. M., and Battisti, D. S.: ENSO-like interdecadal variability: 1900–93, J. Climate, 10, 1004–1020, https://doi.org/10.1175/1520-0442(1997)010<1004:ELIV>2.0.CO;2, 1997.