the Creative Commons Attribution 4.0 License.
the Creative Commons Attribution 4.0 License.
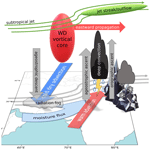
Western disturbances and climate variability: a review of recent developments
Kieran M. R. Hunt
Jean-Philippe Baudouin
Andrew G. Turner
A. P. Dimri
Ghulam Jeelani
Pooja
Rajib Chattopadhyay
Forest Cannon
T. Arulalan
M. S. Shekhar
T. P. Sabin
Eliza Palazzi
Western disturbances (WDs) are synoptic-scale weather systems embedded within the subtropical westerly jet. Manifesting as upper-level troughs often associated with a lower-tropospheric low over western or northern India, they share some dynamical features with extratropical cyclones. WDs are most common during the boreal winter (December to March), during which they bring the majority of precipitation – both rain and snow – to the western Himalaya, as well as to surrounding areas of north India, Pakistan, and the Tibetan Plateau. WDs are also associated with weather hazards such as heavy snowfall, hailstorms, fog, cloudbursts, avalanches, frost, and cold waves.
In this paper, we review recent developments in understanding WDs and their impacts. Over the last decade, recent studies have collectively made use of novel data, novel analysis techniques such as tracking algorithms, and the increasing availability of high-resolution weather and climate models. This review is separated into six main sections – structure and thermodynamics, precipitation and impacts, teleconnections, modelling experiments, forecasting at a range of scales, and paleoclimate and climate change – each motivated with a brief discussion of the accomplishments and limitations of previous research.
A number of step changes in understanding are synthesised. Use of new modelling frameworks and tracking algorithms has significantly improved knowledge of WD structure and variability, and a more frequentist approach can now be taken. Improved observation systems have helped quantification of water security over the western Himalaya. Convection-permitting models have improved our understanding of how WDs interact with the Himalaya to trigger natural hazards. Improvements in paleoclimate and future climate modelling experiments have helped to explain how WDs and their impacts over the Himalaya respond to large-scale natural and anthropogenic forcings. We end by summarising unresolved questions and outlining key future WD research topics.
- Article
(20901 KB) - Full-text XML
- BibTeX
- EndNote
The Indian subcontinent experiences four distinct seasons. The monsoon, lasting from June to September, brings the majority of India's annual precipitation. This is preceded by the pre-monsoon (March to May), a typically dry, warm season associated with the hottest temperatures of the year, and it is followed by the post-monsoon (October to November), associated with cooler and less cloudy conditions – except over southeast India, which gets most of its annual rainfall during this season from the so-called northeast monsoon. The fourth season, winter, lasts from December to February. This is a wet season for much of the northern subcontinent, including the Hindu Kush, Karakoram, and western Himalaya (WH; for locations of these ranges and other important physical features of the region, see Fig. 1), as western disturbances (WDs) – an eastward-moving synoptic-scale trough embedded in the subtropical westerly jet – impact the region, bringing heavy rainfall to the lower foothills and plains, as well as heavy snowfall to the mountains. The Hindu Kush receives up to 80 % of its annual precipitation during the winter months, whereas the WH receives a little less than half – with this fraction decreasing quickly towards the south and east (Fig. 2). WDs differ considerably from monsoon low-pressure systems, the other synoptic-scale vortex that regularly affects the subcontinent, whose development and propagation is driven by moist thermodynamics coupled to the mean monsoon flow.
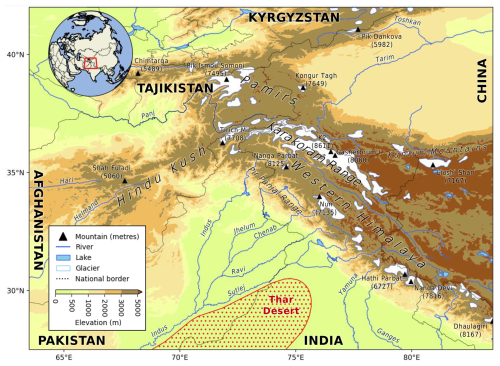
Figure 1Map of the western Himalaya, Karakoram, and Hindu Kush mountain ranges. Glacierised regions, large rivers, and selected mountains are marked. National borders are for illustration only and do not necessarily represent the views of all authors.
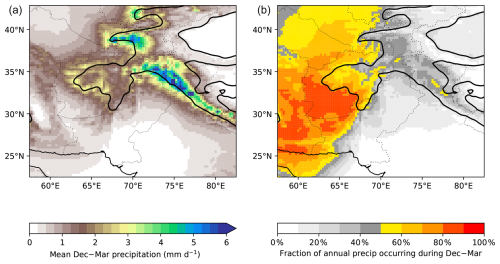
Figure 2Climatological winter (December to March) precipitation over the western Himalaya and Hindu Kush region. (a) Mean winter precipitation. (b) Fraction of annual precipitation that falls during the winter months. Black contours denote smoothed orography at heights of 1, 2.5, and 5 km. Precipitation data are from ERA5, covering 1950–2021. State borders are marked with a thin dotted line.
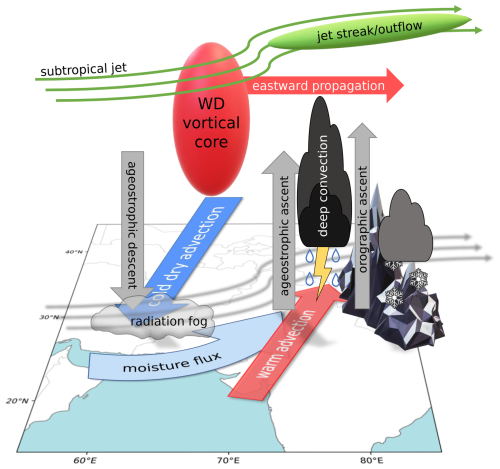
Figure 3Schematic outlining some of the key meteorological features of western disturbances as they approach the western Himalaya. The WD is characterised by a high-vorticity region (red) propagating eastward along the subtropical jet (green). It is associated with a transverse ageostrophic circulation, including broad descent to the west and a broad ascent to the east. Within the latter area, strong orographic ascent occurs along the mountain barrier of the Himalaya. Its outflow forms a jet streak downstream. The cyclonic circulation often also extends to the surface. Northerly advection of cold and dry air occurs to the west, which with the large-scale descent produces ideal conditions for radiation fog. To the east, warm southerly advection brings moisture from the Arabian Sea. Large-scale ascent, orographic forcing, moisture supply, and latent instability promote deep convection, resulting in heavy rain over the lowlands and snow over the foothills (in winter) and mountains.
1.1 What are western disturbances?
WD cyclogenesis occurs to the west of the Hindu Kush and Himalaya (Mull and Desai, 1947; Pisharoty and Desai, 1956), over regions such as the Mediterranean Sea (Madhura et al., 2015). Cyclogenesis occurs as small-scale instabilities in the jet grow into deeper troughs in the presence of baroclinic instability (Singh et al., 1981). They then propagate as troughs embedded within the subtropical westerly jet until they reach South Asia (Singh, 1971). Early studies established that WDs move eastward at speeds of 6–12 m s−1, although they disagreed on their lifetime – usually because of inconsistent definitions of genesis and decay; they typically fall in the range of 2 to 12 d (Datta and Gupta, 1967; Rao and Srinivasan, 1969; Chattopadhyay, 1970; Rao and Rao, 1971; Subbaramayya and Raju, 1982). Rao and Srinivasan (1969) detected an average of six to seven WDs per month in winter; in contrast, WDs are comparatively rare during the summer months (Pisharoty and Desai, 1956), although more recent studies have discussed their importance when interacting with the monsoon trough (e.g. Chevuturi and Dimri, 2016).
WDs typically pass over Iran, Iraq, Afghanistan, Pakistan, and India but occasionally make it as far east as Nepal, Bangladesh, and China (Pisharoty and Desai, 1956; Rao and Srinivasan, 1969). Their impacts are usually greatest over north India and the WH, where they bring cold waves (Mooley, 1957; De et al., 2005), fog (Rao and Srinivasan, 1969; Syed et al., 2012; Dimri and Chevuturi, 2014b), and avalanches (Ganju and Dimri, 2004), and where baroclinic interaction with the orography can result in heavy precipitation (Ramaswamy, 1956; Singh and Agnihotri, 1977; Vellore et al., 2016).
Even with scarce in situ measurements, diligent use of observations – particularly upper-air soundings and satellite data – means that synoptic-scale WD structure has been well understood for some time. The presence of an associated upper-level trough was first identified by Singh (1963). Chitlangia (1976) used a composite of six WDs to show that above the surface low, WDs were associated with vorticity and ascent structures that extended through much of the troposphere, capped by a region of upper-tropospheric divergence. Similar results were reported in subsequent case studies by Singh and Kumar (1977, and others), who further identified that frontal regions were sometimes associated with the passage of a WD, particularly in the upper-troposphere. Furthermore, Rao and Rao (1971) observed that WDs were embedded in a region of strong vertical wind shear, from which they deduce their predominantly baroclinic nature.
WD structure was progressively refined over the following decades as satellite data, reanalyses, and higher-resolution models became increasingly available. The salient features of WDs, summarised by Dimri et al. (2015) and Dimri and Chevuturi (2016), are a strong upper-level trough (i.e. a geopotential height minimum) embedded in the subtropical westerly jet, which is usually associated with a weaker surface low; sometimes with frontal features, which can occur at any level, but which tend to weaken as they approach the Himalaya; broad ascent ahead of the system centres – often associated with heavy precipitation – and broad descent behind them; and strong vorticity at their centre, peaking in the upper levels. Thus, the dynamics of WDs sit somewhere between extratropical cyclones and mid-latitude upper-tropospheric troughs. Their unique proximity to both mountains and a maritime moisture source, however, leads to a unique array of impacts that are not found in other regions. Key characteristics of the WD, along with its environment, are summarised in Fig. 3.
1.2 Why are western disturbances important?
WDs are the dominant synoptic winter weather pattern over the western Himalaya and surrounding region and are associated with a wide range of impacts – including cloudiness, rainfall, snowfall, fog, cold waves, and avalanches. Understanding their evolution is therefore key across all timescales, from weather to seasonal to climate. Growing evidence also suggests that WDs are impacting weather outside the winter, leading to extreme precipitation events, which we discuss in Sect. 3.5.
Winter precipitation brought by WDs is important for both water security (Benn and Owen, 1998; Archer and Fowler, 2004; Thayyen and Gergan, 2010) and agriculture (Yadav et al., 2012). Reservoirs across the region are filled by meltwater from the winter snowfall during the subsequent spring melt season and supply the spring rabi crops (e.g. barley and wheat), which are important components of the food security of the subcontinent. This meltwater is key to mitigating the seasonal drought that occurs in May and June before the arrival of the summer monsoon. Late WDs occurring during the spring or early monsoon can also impact kharif crops (e.g. rice and maize). As a result, WDs both directly and indirectly impact the economies of India, Pakistan, and neighbouring countries. On climate timescales, winter precipitation brought by WDs is a vital component of glacier mass balance in a region known for its anomalous glacial advance (the Karakoram Anomaly; see Sect. 7.2.2).
1.3 Why is an updated review needed?
A comprehensive review of WD literature was carried out by Dimri et al. (2015), which was followed by a short book (Dimri and Chevuturi, 2016). Research since then largely falls into four categories.
Firstly, recent studies have made increased use of high-resolution models, which are becoming cheaper to run, both for regional climate modelling and numerical weather prediction. Research on WDs, especially of their impacts, has benefitted from these developments, not only because the models are now often convection-resolving – and much of the heavy precipitation associated with WDs is convective – but also because of the improved representation of orography and the land surface with which WD-driven flows have a complex relationship (see Sects. 2.3.2 and 5.2.1). The large number of high-resolution experiments also act as a primitive large ensemble. As these models better capture small-scale processes, experiments can more easily establish which physics schemes, forcings, and configurations are most important, reducing the model uncertainty from which earlier studies suffered.
Secondly, the development of automated tracking techniques has allowed recent studies to explore the climatology of WD structure and behaviour in reanalyses and climate model outputs, revealing much about their variability and response to climate change (see Sects. 2.3.3, 7.2, and 7.3). These developments have helped to link the physical processes of individual storms to the larger weather systems they occur in, clarifying the direct influence of climate change on the statistical behaviour of WDs.
Thirdly, recent studies have made use of the increasing availability of isotope analysis, which, when coupled with trajectory analysis and composite moisture flux analysis, can provide valuable insights into the moisture sources of WD precipitation (see Sect. 2.4). This research has led to findings that WDs draw most of their moisture from the Arabian Sea rather than the Mediterranean as often previously thought.
Fourthly, significant advances in paleoclimate proxy techniques and associated modelling have allowed studies to better quantify the behaviour of WDs and winter precipitation during ancient climatic epochs (see Sect. 7.1). These studies have revealed periods of increased and suppressed WD activity, primarily through speleothem and sediment analysis, showing significant variability on centennial timescales.
This review is laid out in a thematic manner that follows previous reviews, covering structure and dynamics (Sect. 2), precipitation and impacts (Sect. 3), large-scale variability (Sect. 4), model evaluation and verification (Sect. 5), extended-range and seasonal forecasting (Sect. 6), and finally climate change (Sect. 7). The section on climate change is further subdivided into paleoclimate (Sect. 7.1), observed responses in the instrumental record (Sect. 7.2), and the projected response to future climate change (Sect. 7.3). Each section starts with a summary of older literature to orientate the reader and to provide context for the newer research. Each section then concludes with a short summary statement, including a measure of confidence in the main points, following the IPCC calibrated uncertainty language. These statements are summarised in Sect. 8.1. Finally, in Sect. 8.2, we outline the important unresolved questions and open research topics that remain for WDs.
We have made a reasonable attempt to include all published literature on WDs, except where manuscripts were not accessible, either due to cost (e.g. being in a book) or language. In some parts of this review, we have included additional papers that cover winter precipitation over the relevant region, as this can be a useful proxy for WD frequency and such papers can add useful evidence to the discussion.
2.1 Summary of earlier research
As discussed in Sect. 1.1, the main structural features of WDs – upper-level troughs (i.e. cyclonic potential vorticity anomalies) in the subtropical jet which often extend to the surface and sometimes have associated frontal features, as well as the large-scale ascent they produce to the east and descent to the west – have been established through multiple case studies collectively using surface observations, upper air soundings, and satellite data. They share some structural and dynamical similarities with extratropical cyclones but differ in scale and intensification mechanisms (Riehl, 1962). Like extratropical cyclones, the available potential energy for WDs comes from the meridional temperature gradient and resulting vertical wind shear (Dimri, 2008). However, many features present in extratropical cyclones, such as frontal fractures, sting jets, and warm seclusions (Dacre, 2020), have not yet been observed in WDs.
As deep troughs, WDs are associated with high vorticity in the mid-troposphere which can be further enhanced through orographic interaction and diabatic heating (Rao, 2003; Hara et al., 2004; Dimri and Chevuturi, 2014b). The high levels of vorticity present in WDs and the lack of other westerly sources in the mid-troposphere and upper troposphere over South Asia makes them ideal candidates for automated tracking algorithms, from which much-needed climatologies can be produced; however, no such work was undertaken prior to 2015, and so there has been a reliance on case studies (Hara et al., 2004; Dimri and Chevuturi, 2014b). In addition, as even fairly primitive reanalyses have been shown to simulate WD structure well (though using an Eulerian rather than Lagrangian method; Mohanty et al., 1998, 1999), despite scarce observations available for assimilation, they can be combined with track databases to improve understanding of WD structure and variability. This has been one of the key foci of recent WD research.
WD precipitation is characterised by rainfall at lower elevations and snowfall at higher elevations (Barros et al., 2004) and has significant impacts on both lowland agriculture (Yadav et al., 2012) and water storage at higher altitudes (Benn and Owen, 1998; Archer and Fowler, 2004; Bolch et al., 2012). The interaction between orography and the structure and dynamics of WDs is key to understanding the mechanisms of precipitation formation over the western Himalaya. In addition, large-scale baroclinic conditions and localised orographic forcing also impact the passage of WDs. The feedback between moist processes and orography constrains both the amount and type of precipitation (Smith, 1979; Houze, 2012).
Moisture is a key component of WDs, as the winter atmosphere over the western Himalaya is typically quite dry. While classical research argued that WDs brought moisture with them from the Atlantic Ocean or Mediterranean Sea (Mull and Desai, 1947; Pisharoty and Desai, 1956; Rao and Srinivasan, 1969), more recent analyses of moisture flux cast doubt on this idea (Dimri and Mohanty, 1999; Raju et al., 2011), implying a more local source such as the Arabian Sea. As we will see, recent developments in isotope analysis, more systematic composite analyses, and the novel development of moisture back-trajectory analysis have helped to resolve this disagreement on WD moisture sources.
2.2 Detection and tracking
Detection of WDs depends on the characteristics of the WD being detected and therefore varies considerably between algorithms. These can be broadly categorised into top-down and bottom-up approaches. Bottom-up approaches include case studies and Eulerian composites, often starting with the impacts of WDs and then working “upwards” to quantify the characteristics that drive these impacts. Top-down approaches use a prescribed WD characteristic (e.g. vorticity), often combined with Lagrangian compositing and then working “downwards” to quantify surface impacts.
Before 2015, bottom-up approaches were the most common. Dimri (2013) used band-pass-filtered precipitation and outgoing longwave radiation (OLR) to build the first composite analysis that showed WDs leading to heavy precipitation were associated with strong southwesterly moisture flux and deep convection. More recently, Baudouin et al. (2021) used more advanced statistical tools (principal component regression) to quantify the link between precipitation and specific atmospheric patterns. They showed, in particular, that 68 % of precipitation variability in the Upper Indus Basin can be explained by the presence of an upper-tropospheric geopotential low (i.e. a WD) and that this number increased to 88 % when taking into consideration the mid- to lower-tropospheric structure of the WD. Using composite analyses and clustering, they further showed how different WD characteristics lead to different impacts in the study area (see Sect. 3.4). While these studies do not directly detect specific WD events, they help to define the characteristics of WDs that lead to precipitation.
In the last decade, numerous studies have instead used top-down approaches, and more specifically tracking algorithms. Automated tracking algorithms allow the relatively quick production of multidecadal catalogues that can then be leveraged to compute basic statistics such as track frequency and density, as well as more advanced Lagrangian statistics, such as the mean and variability of storm-centred structure. Feature tracking is the only way to get storm-centred composites, which reduce large-scale feature smoothing due to differences in WD location, although smaller-scale features – such as fronts – typically disappear from such composites. However, tracking algorithms often simplify the analysis of WDs to just a few parameters, such as location/tracks and intensity of the centre, and so they do not define the entire spectrum of WD characteristics, although these can be largely reconstructed with further analysis.
Tracking has been done successfully for, for example, extratropical cyclones (Dacre et al., 2012) and monsoon depressions (Hurley and Boos, 2015). In fact, depending on the detection criteria used, WDs can appear in global climatologies of extratropical cyclone tracks (Wernli and Schwierz, 2006) and their features (e.g. warm conveyor belts Madonna et al., 2014). However, only recently have authors started to track WDs in reanalysis data. The first systematic attempt came from Cannon et al. (2016), whose methodology loosely followed that used in thunderstorm tracking (e.g. Dixon and Wiener, 1993; Carvalho and Jones, 2001). Using reanalysis data from 1979–2013, they identified regions of negative 500 hPa geopotential height anomaly, using a thresholding approach to allow detection of multiple centres within a large region of negative anomalies. Regions smaller than 5° in length were then removed and the remaining regions linked using a spatial correlation (overlap) technique. They identified 600 WDs in total (∼18 per year), which is less frequent than reported in earlier studies on account of their strict approach.
Hunt et al. (2018b) used a different approach. They computed the T63 spectral truncation of the relative vorticity averaged between 450 and 300 hPa, using ERA-Interim (ECMWF Reanalysis – Interim) reanalysis data from 1979–2015. Using this field to identify local maxima, regions of positive vorticity were then linked using a kd-tree nearest-neighbour approach. Tracks that did not pass over South Asia (defined as 20–36.5° N, 60–80° E) and were shorter than 48 h in duration or that did not propagate eastwards were removed. They identified 3090 WDs in total (∼86 per year). This is more frequent than reported in earlier studies, as this approach detects many very weak systems. More recent studies have suggested the northern boundary of this capture region is too far south to capture all relevant systems and have instead proposed using 42.5° N (Bharati et al., 2025).
Later work, described in Nischal et al. (2022), extended this vorticity-based tracking approach to the newer ERA5 (ECMWF Reanalysis version 5) and IMDAA (Indian Monsoon Data Assimilation and Analysis) reanalyses, returning similar frequencies. Qiu et al. (2022) used a similar method in their study of WDs over southern China: identifying contours of s−1 in 450–300 hPa layer mean relative vorticity, then linking candidates if these areas overlapped more than 60 % in successive 6 h reanalysis time steps and the areas share similar physical properties, and rejecting tracks shorter than 24 h. They identified 2594 WDs passing through their study region (20–40° N, 80–105° E) in December to February 1979–2017, a similar frequency to that reported by Hunt et al. (2018b).
To obtain WD tracks for their analysis of the Karakoram Anomaly, Javed et al. (2022) applied an objective feature-tracking algorithm (Hodges, 1995, 1999) to T63 relative vorticity averaged between 400 and 300 hPa in three reanalyses, finding and linking maxima in regions exceeding s−1. They then rejected tracks shorter than 24 h or 1000 km, as well as those whose genesis was not in their genesis study region (20–50° N, 20° W–60° E). See Sect. 7.2.2 for a full discussion of WDs and the Karakoram Anomaly.
The reader will have noticed that each of these studies uses different criteria – both capture regions and minimum track lengths or durations – to filter their WDs. This is because no standard yet exists, and so authors typically choose their capture regions to reflect the impacts they want to investigate. This makes intercomparison between studies challenging, as even basic statistics such as frequency are sensitive to these choices. Therefore, based on the discussion in this section, we propose basic criteria that could be adopted in future WD-tracking studies in order to standardise results. Firstly, rather than a capture region, where the choice of longitudinal extent can have a significant impact on the characteristics of the WDs in the final catalogue, we propose simply that WDs must cross 70° E (to the east of almost all genesis areas, see Sect. 2.3.1, and to the west of the regions of greatest impact) and do so between 20° N (to filter out tropical systems) and 50° N (to filter out polar systems but retain northward-tracking WDs that can still have an impact over the Karakoram or Pamirs). We also propose a minimum track duration of 48 h to filter out transient systems but no minimum track length, as WD genesis can occasionally be very close to the western Himalaya.
Other studies have used simpler, indirect techniques to approximate WD frequency and related statistics (e.g. intensity), either in reanalyses or climate model output. A list summarising these is given below:
-
850–200 hPa thickness, averaged over 25–40° N, 60–80° E (Midhuna et al., 2020);
-
empirical orthogonal functions (EOFs) of high-pass-filtered daily 500 hPa geopotential height, computed over 25–40° N, 50–100° E (the first two of these EOFs describe a wave train strongly resembling WD propagation and explain more than 50 % of the variance (Madhura et al., 2015));
-
spectral power of 200 hPa geopotential height in the 5–15 d band, motivated by linking upper-level wave trains with heavy winter precipitation over Pakistan and north India (Cannon et al., 2015) (this is averaged over several different regions);
-
variance of 4–15 d band-passed 200 hPa geopotential height, also computed over various regions (Krishnan et al., 2019a);
-
k-means clustering on low-level winds (10 m, 925 hPa, 850 hPa) and mean sea-level pressure over India (this gives 30 distinct weather regimes, four of which are associated with WD-like activity (Neal et al., 2020); a similar approach, applied just to the Karakoram and western Himalaya, was used in Riley et al. (2021)).
Midhuna et al. (2020) showed that their index had a correlation coefficient of only 0.18 with annual WD frequency compiled from weather reports, indicating that proxy techniques like these are not necessarily reliable. In the case of Midhuna et al. (2020), this is because they characterise WDs by a cold troposphere; while this may work well for individual events, seasonal mean tropospheric temperature is more strongly a function of jet position. This wide range of indirect techniques leads to a wide range of estimates of WD statistics, such as frequency. Quantifying these uncertainties is made significantly more challenging by the sensitivity of each method to the cutoff thresholds used to define WD activity (e.g. variance), and so they are difficult to compare directly. However; top-down and bottom-up approaches are complementary, and their combination helps to build new understanding of WD processes. Thus, there is high confidence (robust evidence, medium agreement) that tracking algorithms have improved our understanding of WDs, but there is low agreement on how such algorithms should be implemented.
2.3 The life cycle of WDs
Tracking algorithms provide an objective way to build up large catalogues of WD tracks. These, in turn, can be analysed to provide new information about the climatology (i.e. average behaviour) and variability of WDs. A summary of the typical pathway in which WDs interact with their environment over South Asia is given in Fig. 4, from Baudouin et al. (2021). WDs typically pass along the northern edge of the subtropical jet, and upon arriving over the western Himalaya, they induce moist southwesterlies in their front sector, resulting in cross-barrier flow and precipitation, and cold northerlies in their rear sector, resulting in cold waves and fog.
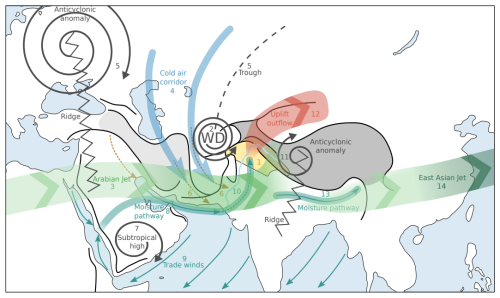
Figure 4From Baudouin et al. (2021). Sketch summarising the atmospheric circulation when a WD interacts with orography to produce precipitation in the Upper Indus Basin (in yellow). The Indus River is shown in pale blue. The black lines indicate major mountain ranges, and the grey-shaded areas indicate high plateaus (the Tibetan Plateau and the Iranian Plateau). The spirals represent tropospheric geopotential anomalies or centres of actions. The thin green arrows are mean lower-troposphere moisture transport. A thicker arrow width indicates higher-altitude transport, and a dotted line indicates transient transport due to the WD. The dotted brown lines indicate advection of dry air by the WD near the surface. The blue arrows denote troposphere-wide cold-air advection. The thick green arrows represent the upper-troposphere jets, with darker colour indicating stronger winds. The red arrow relates to the warm upper-troposphere outflow. The numbers are in-text references to Baudouin et al. (2021).
2.3.1 Cyclogenesis
Although the official India Meteorological Department (IMD) definition of WDs states that they “originate over the Mediterranean Sea, Caspian Sea and Black Sea” (https://www.imdpune.gov.in/Reports/glossary.pdf, last access: 1 January 2025), even very early studies expanded on this, stating that WD cyclogenesis could occur in maritime regions spanning from the North Atlantic to the Persian Gulf (Pisharoty and Desai, 1956).
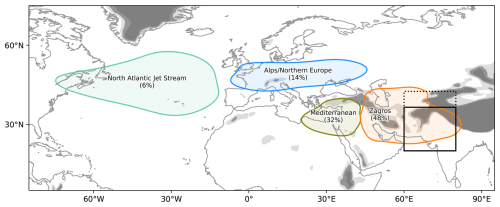
Figure 5Regions of climatological WD cyclogenesis, computed using the WD track dataset described in Hunt (2024). Genesis points from all ∼6000 winter WDs in the dataset are clustered into four groups using a simple k-means approach. A Gaussian kernel density estimate is then applied to each cluster, with the 90 % contour plotted. Regions are labelled after upstream mountain ranges or water sources, with the figures in parentheses indicating the fraction of the total WD population with geneses in that region. Filled grey contours represent smoothed orography at heights of 1, 1.5, and 2 km. The black box indicates the WD capture region used in some tracking studies (20–36.5° N, 60–80° E). The dotted box shows the extension to 42.5° N which has also been used in more recent studies.
However, such studies are built on collections of case studies and are thus not necessarily representative of the whole population of WDs, as they tend to focus on high-impact events – usually driven either by intense WDs or those passing over a certain region. Objectively derived track databases, such as those of Cannon et al. (2015), Hunt et al. (2018b), and Javed et al. (2023), all agree that there is very little geographical preference for WD cyclogenesis except for the following, which are summarised in Fig. 5.
-
All WDs originate from regions to the west of the western Himalaya or occasionally spin up in situ. This occurs largely because most WDs are advected along the subtropical westerly jet, so the flow configurations to advect systems westward or southward into the region virtually never occur.
-
There is no obvious preference for cyclogenesis over bodies of water, with only around a third of WDs spinning up over the Mediterranean (and a much smaller fraction over the Caspian and Arabian seas).
-
The vast majority of WDs spin up quite close to the western Himalaya, with nearly half of all cyclogenesis occurring to the east of the Zagros mountain range in Iran, between one and two WD wavelengths upstream of the western Himalaya.
-
Cyclogenesis tends to occur in regions of dynamic instability, often found downstream from mountain ranges or within the North Atlantic jet stream.
Beyond this, several important questions remain for future research about the relationship between the WD cyclogenesis regions and downstream characteristics and impacts, as well as whether there are different WD cyclogenesis mechanisms.
2.3.2 Intensification and moist thermodynamics
There are three potential pathways through which WDs can intensify. On the largest scale, WDs in the subtropical jet experience strong vertical wind shear and hence can grow through dynamical baroclinic instability (Grotjahn, 1993; Lee and Kim, 2003). As they approach the Himalaya, the orography offers a new source of baroclinic instability, subject to the jet–orography configuration (Roads, 1980; Reinhold, 1990; Brayshaw et al., 2009). Finally, as with all rotating precipitating systems, precipitation – whether free or forced – can lead to intensification through vortex stretching, driven by diabatic heating and updraughts (e.g. Tory and Frank, 2010). This effect is most pronounced for convective precipitation, which often occurs in WDs. Stratiform clouds are also common along the foothills (Fig. 6) and therefore may also play a role in WD intensification, but this has not yet been investigated.
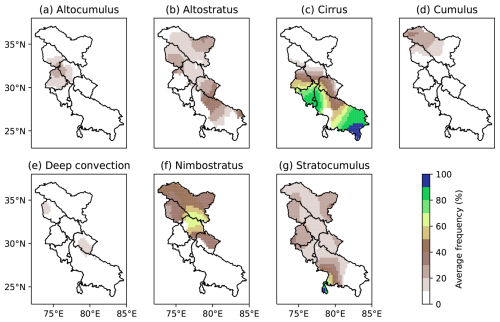
Figure 6Adapted from Kumar et al. (2019a). Mean frequency of seven core cloud types (stratocumulus, cumulus, altocumulus, altostratus, nimbostratus, cirrus, and deep convective) over the northern states of India, between 2007 and 2016. Computed for all days (not just WDs) in winter months (December to March) using CloudSat data.
WDs intensify as they reach the western Himalaya, reaching maximum intensity – both in vorticity and updraught strength – about a day before their centre passes over the orography (Hunt et al., 2018b). Once they have passed the orography (i.e. the western Himalaya), they quickly dissipate and are thus less commonly found over northeastern India or Bangladesh, with comparatively little WD-associated precipitation falling over the central and eastern Himalaya (Dimri et al., 2023). WDs that do reach the eastern Himalaya may also interact constructively with the India–Burma trough (Wang et al., 2011; Li et al., 2017).
The processes linking WD dynamics to ascent are neither linear nor readily separable from the role of orography. To first order, WD-driven ascent is the quasigeostrophic response to a potential vorticity (PV) anomaly in strongly sheared flow, as demonstrated in both case studies (Kumar et al., 2017) and larger composite samples (Sankar and Babu, 2020, 2021). The latter studies demonstrated some interesting corollaries: updraughts can extend from near the surface to as high as 200 hPa (Sankar and Babu, 2020), and there is no significant relationship between convectively available potential energy (CAPE) and WD precipitation (Sankar and Babu, 2021). However, Para et al. (2020) used a case study of extreme precipitation over Jammu and Kashmir in 2017 to show that WD circulation can be coupled with convection, as latent heat release increases the strength of the lower-tropospheric PV anomaly. They also suggested that cold-air intrusions from the midlatitudes destabilise the mid-troposphere, leading to increased baroclinic growth of the WD, later corroborated by Hasan and Pattnaik (2024).
Indeed, it has been through precipitation that most recent studies have approached the topic of WD intensification. Two key ingredients are required for WDs to precipitate: moisture influx and updraughts to raise that moisture aloft. We discuss potential moisture sources later in Sect. 2.4 but review here recent research on the processes that lead to WD precipitation across the western Himalaya and their influence on WD dynamics.
Baudouin et al. (2021) approached this problem using a novel, generalised framework. They decomposed the moisture flux associated with heavy precipitation over the Upper Indus Basin and showed that passing WDs interact baroclinically with – and strengthen – the low-level moist flow from the Arabian Sea moving towards and over the orography. They linked the strength of this interaction – and hence the magnitude of the precipitation – to the intensity and thermal structure of the passing WD, substantiating the work of Hunt et al. (2018b), who established a statistical link between WD intensity and associated precipitation. Baudouin et al. (2021) further showed that if a WD is too far north or too weak, the baroclinic strengthening of the moisture pathway is reduced, limiting precipitation.
A complementary mechanism was proposed by Laskar et al. (2015), who examined two case studies of intense WDs. They argued that moisture influx could also be caused by an extension of the lower-level WD trough into the Arabian Sea, with anomalous southerly moisture transport occurring along its downshear (i.e. eastern) edge. This may simply be a different interpretation of the atmospheric river framework above (see also end of Sect. 2.4), but both theories explain why the heaviest precipitation falls ahead (i.e. to the east) of WDs and why precipitation is so sensitive to WD latitude. On one hand, the WD needs to extend far enough south to interact with the mean moisture flux over the Arabian Sea, and, on the other hand, it should be at the right latitudinal position to interact with the relief. Frontal regions, if they are still present in the WD by the time it reaches the Himalaya, may also play a role in uplifting moisture ahead of the system.
Once moisture arrives over the continent, it must be lifted in order for heavy precipitation to occur. There are two available mechanisms for updraughts in the environment of a WD: ascent can be mechanically forced through interaction between low-level flow and the orography, or it can be quasigeostrophically forced through the dynamics of the WD itself (see Sect. 2.3.4). These two mechanisms can interact constructively, strengthening the WD as it approaches the western Himalaya. Little work (limited to Dimri, 2004) has been done on explicitly quantifying the role of the Himalayan orography in producing WD precipitation, perhaps because underlying processes can be mostly understood through the interaction of moist flow with complex topography in other regions – e.g. the Andes (Insel et al., 2010) and the Rockies (Silverman et al., 2013). Baudouin et al. (2020b) showed that low-level southerly moisture transport explains about 80 % of the variance of winter precipitation over the Himalayan foothills, strongly implying the importance of the forced uplift of cross-barrier (i.e. perpendicular to the orography) flow. They argued that the vertical structure of the moisture flux was important in such cases: moisture transport at 700 hPa had the strongest control on variability, and transport at altitudes higher than 500 hPa typically passed over the orography unimpeded. Battula et al. (2022) corroborated this transport argument in their climatological study, further arguing that strong WDs are the only weather system capable of pushing moisture to high altitudes in the western Himalaya. This transport can result in substantial diabatic heating and subsequent intensification of the WD (Hasan and Pattnaik, 2024).
As we have seen, WDs can intensify via baroclinic feedback between their primary circulation and induced low-level southerlies undergoing forced orographic ascent ahead of the system. Baudouin et al. (2021) showed that this interaction could dramatically slow the passage of a WD by enhancing the divergence maximum downstream of the system. This divergent outflow is then directed against the eastward propagation of the WD. This is supported by Chand and Singh (2015), who used satellite data to find a negative correlation between WD propagation speed and downstream cloud-top height, implying that WDs associated with stronger convection tended to propagate more slowly. Chand and Singh (2015) also showed that WD propagation speeds vary substantially, from 280 to 670 km d−1. They found that convection was typically deepest 1200 km ahead of the WDs and associated with cloud-top temperatures between −50 and −60 °C. In two WD case studies from early 2017, Sankar et al. (2021) estimated the deepest convection occurred much closer to the centre, only about 200–400 km east of the mid-level trough, typically over orography, but agreed on cloud-top temperatures as low as −60 °C. This disagreement on distance between the WD centre and its deepest convection suggests that it is highly variable and driven by both WD dynamics and orographic forcing.
Despite this association with deep convection, WDs are usually more easily recognised by their stratiform footprint. This was highlighted recently by Kumar et al. (2019b), who showed using the DARDAR (radar/lidar) dataset (Ceccaldi et al., 2013, spanning 2007–2016) that during the passage of WDs over northern India, the most common cloud types are nimbostratus (33 %) and cirrus (21 %), with deep convection being comparatively uncommon (2.3 %) even though it is associated with the most severe weather impacts. A companion study (Kumar et al., 2019a) found that about 25 % of all clouds over northern India occur during the passage of WDs. These results are summarised in Fig. 6.
In summary, the deep ascent ahead of WDs primarily occurs due to downstream upper-tropospheric divergence. This is supported by quasigeostrophic differential vorticity advection and mechanical uplift of induced lower-level southerlies as they interact with the orography. As WDs approach the Arabian Sea, these southerlies induce strong lower- to mid-tropospheric southerly moisture flux from the Arabian Sea. As this moisture reaches the orography, it subsequently ascends through a non-linear combination of mechanical orographic forcing, quasigeostrophic ascent, and convection, primarily responding to the central PV anomaly of the WD. This is borne out in the statistical relationship between stronger WDs (i.e. those associated with stronger upper-tropospheric vorticity) and more intense precipitation (Cannon et al., 2016; Hunt et al., 2018b). However, orography is not required for WDs to precipitate, as neither the convection nor quasigeostrophic forcing depends on it. The relative importance of each of the known intensification pathways – large-scale baroclinic, orographic-baroclinic, and convective vortex stretching (and there may be others) – to the growth and maintenance of WDs remains an open question.
2.3.3 Track density
Although the regions impacted by WDs have been known for some time, track databases allow a more rigorous quantification of their frequency and track density, as in Fig. 7, which we use to summarise the results of those track databases outlined above.
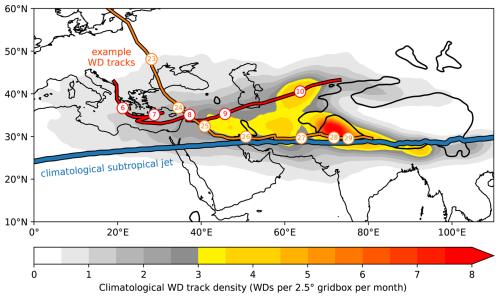
Figure 7Following Hunt (2024). Filled contours show climatological track density of winter (December–March) WDs, computed from 1950–2022 using a track database deriving from ERA5. The thick blue line shows the mean location of the subtropical jet for the same season. The red and orange lines denote tracks of two WDs that occurred in January 2020. Circular markers denote the location of that WD at 00:00 UTC on the given day of the month. The thick black line denotes a 2 km contour of smoothed orography.
The highest track density is around the western Himalaya, Karakoram, and Hindu Kush. WDs (or their trough precursors) are funnelled into this region by the subtropical jet, whose mean location is very slightly to the south of the mountain ranges. There, the systems are amplified by local instability and moist processes, resulting in increased track density as existing WDs strengthen and pre-existing troughs in the subtropical jet intensify into WDs (Baudouin et al., 2021).
There is also a branch of tracks that are deflected to the north of the Tibetan Plateau, with the split happening at approximately the location of the Pamir Mountains. Little is known about this split, except, as we discuss in Sect. 6, that it is very sensitive to the latitude of the subtropical jet and can have significant implications for forecasting WD impacts.
Tracks from two individual, intense WDs, occurring in January 2020, are also plotted in Fig. 7 and demonstrate the significant variability that can be present in WDs occurring just a few weeks apart. The first WD spun up over the Mediterranean on 5 January, initially moving slowly before passing rapidly to the north of the Himalaya. The second WD spun up over northern Europe on 22 January. It then migrated southward, before moving rapidly towards and then over the western Himalaya, where it subsequently resulted in heavy precipitation. This again highlights the very different backgrounds that WDs can have and the forecasting challenges that result.
This difference demonstrates that not all WDs arise as instabilities on the subtropical jet – but also as extratropical cutoff lows that migrate equatorward (Wernli and Sprenger, 2007; Portmann et al., 2021). Cutoff lows arise when tongues of high-PV upper-tropospheric air detach from the extratropical westerlies, and they tend to move more slowly than WDs embedded in the subtropical jet, sometimes leading to severe weather (Kalshetti et al., 2022). They comprise about 10 % of all WDs (Thomas et al., 2023), but this number is uncertain given difference in tracking methodologies. Little is known about the differences between cutoff low WDs and those arising within the subtropical jet itself.
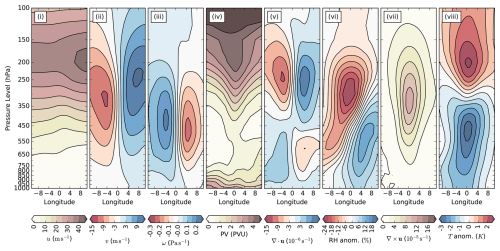
Figure 8From Hunt et al. (2018b). Vertical system-centred composites of WD structure over northern India and Pakistan, taken from west to east through the centre, which is at 0° relative longitude. From left to right, the composite fields are (i) zonal wind speed (m s−1), (ii) meridional wind speed (m s−1), (iii) vertical wind speed (Pa s−1), (iv) potential vorticity (PVU; 10−6 K m2 kg−1 s−1), (v) divergence (10−6 s−1), (vi) relative humidity (%), (vii) relative vorticity (10−5 s−1), and (viii) temperature anomaly (K). The anomalies are computed against a 20 d mean centred on the WD event. Longitude is defined relative to the centre of the WD composite.
2.3.4 Vertical structure
Using either Lagrangian tracking techniques or an Eulerian proxy to develop catalogues of WD events, recent studies have employed compositing techniques to further understanding of WD structure and variability far beyond that provided by earlier case studies. Compositing can provide useful insights into bulk structure, with the caveat that finer structural features are smoothed out. Perhaps the most important example of this caveat is the frontal regions of WDs: early case studies indicated that WDs arriving over Pakistan and north India were sometimes associated with frontal weather (Pisharoty and Desai, 1956; Singh and Agnihotri, 1977), but such discontinuities in meteorological fields were not present in the multivariable composite presented in Hunt et al. (2018b). This is likely because such features are often smeared out in composites as they are located irregularly relative to the centre, and this does not disprove their existence. Extratropical cyclone research has included front-centric compositing using theta/theta-e gradients or wind shifts (e.g. Dacre et al., 2012), but as yet no similar analysis has been done to establish if surface fronts are consistent features in WDs.
Regardless, much has been learned from applying compositing and clustering methods to WD catalogues, which we will now discuss. Hunt et al. (2018b) used the 3090 WDs in their database to produce multivariate composite cross sections, such as the vertical–zonal cross sections in Fig. 8. Many of the features presented there are consistent with earlier case studies and demonstrate the strongly baroclinic structure of WDs: a slight northwestward tilt with height (see, for example, the tilt of the 0 m s−1 meridional wind isotach), displacement of the temperature and geopotential minima, and a quadrupole in divergence, leading them to remark that WDs resemble “immature extratropical cyclones”, consistent with the description in earlier studies (Mull and Desai, 1947; Dimri and Chevuturi, 2014b). Qiu et al. (2022) reported an almost identical structure for a large composite of WDs that had propagated (or formed) further downstream and impacted the southeastern edge of the Tibetan Plateau. Sankar and Babu (2020) found in a smaller composite of 10 WDs that warm-air advection increases with height in WDs – another feature that is present in extratropical cyclones. As in extratropical cyclones, the deep PV anomaly associated with WDs – which extends to the tropopause – can result in substantial transport of ozone through advection of mid-latitude stratospheric air into the tropical upper troposphere (Satheesh Chandran et al., 2022).
Contrary to previous studies (Pisharoty and Desai, 1956; Dimri et al., 2015), whose conceptual models placed the strongest winds upstream of WDs, Hunt et al. (2018b) found that the subtropical jet accelerated downstream of the WDs. This was later corroborated by Baudouin et al. (2021), who argued that while there may be some cases of jet intensification upstream of (or “behind”) the WDs, the climatological picture was the reverse. However, they also showed that while the strongest anomalous upper-level winds occur to the east of the WD, the strongest absolute winds occur to its south. There is an important implication to this arrangement: intensifying the jet downstream means that WDs can trigger jet streaks, whose associated ageostrophic circulation can result in regions of strong ascent. As they often behave like Rossby waves, WDs can also impart this ascent through eddy momentum flux from the extratropics (Kalshetti et al., 2022). We will discuss the impacts of such dynamics later.
In summary, WDs are typically associated with a broad vorticity maximum (or geopotential minimum) between 450 and 250 hPa. They are predominantly baroclinic in nature, leading to ascent ahead of the system and descent behind. In the lower levels they induce moist southerlies to the east of their centre and cold northerlies to the west. High relative humidity leads to a deep cloud structure that results in anomalous heating aloft and anomalous cooling in the mid-troposphere and lower troposphere.
There is thus medium confidence (medium evidence, medium agreement) that WD cyclogenesis occurs primarily in regions of dynamic or thermodynamic instability, i.e. over oceans and downstream from mountain ranges. There is very high confidence (robust evidence, high agreement) that WD intensification arises from baroclinic instability which can be further amplified through coupling with moist or orographic processes. There is very high confidence (robust evidence, high agreement) that WDs primarily impact the western Himalaya and surrounding regions – including the Karakoram, Hindu Kush, foothills, and to some extent the plains of Pakistan and north India. There is very high confidence (robust evidence, high agreement) in the dynamical structure of WDs: a mid- to upper-tropospheric vorticity maximum with deep ascent ahead of the vortex centre.
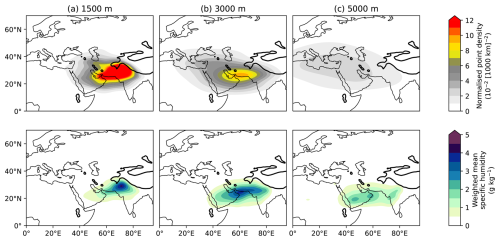
Figure 9Following Hunt et al. (2018c). Potential WD moisture sources identified using trajectory analysis. A 3° × 3° box is drawn around the heaviest precipitation associated with each of the 250 strongest WDs since 1980. From each, 100 uniformly spaced (10×10) particles are released at heights of (a) 1500 m, (b) 3000 m, and (c) 5000 m above the surface. Each particle is integrated backwards in time through 72 h using wind fields from ERA-Interim. The top row shows the spatial probability distribution of such particles at a lead time of 72 h. The bottom row shows the weighted mean specific humidity for particles at all lead times as a function of location, computed by multiplying the normalised point density in the row above by the parcel specific humidity in order to show the relative contributions of different moisture sources at each elevation.
2.4 Moisture sources
As we saw earlier, there has been substantial disagreement among previous studies as to whether WDs draw their moisture from the Mediterranean, the Arabian Sea, Caspian Sea, or local evapotranspiration – each requiring very different synoptic to mesoscale dynamics to support their transport mechanism. Earlier studies also did not agree on how much WD precipitation originated from local sources compared to maritime sources. In this subsection, we discuss how recent studies have attempted to resolve these uncertainties using new methods, including isotope analysis, moisture trajectories, and improved compositing techniques. Compositing – i.e. an Eulerian analysis of the typical circulation associated with WDs and their environment – provides a general view on moisture sources and pathways but cannot provide a quantification of the individual sources, for which isotope and back trajectories are better suited.
All the isotope studies discussed in this section rely on a quantity called deuterium excess (or “D-excess” for short) which we now briefly explain. D-excess (d) is given by
where δ2H is the ratio of deuterium to hydrogen and similarly δ18O is the ratio of δ18O to δ16O, both expressed as a deviation of the isotope ratio from a standard reference representing the isotope ratio of the mean ocean water (Dansgaard, 1964). D-excess shows different signatures for different moisture sources – ocean evaporation, soil evaporation, and plant transpiration (Aemisegger et al., 2014). D-excess also tends to be higher in atmospheric water vapour that has evaporated from surfaces in less humid climates, and thus precipitation arising from moisture originating from different basins can have different D-excess values (Pfahl and Sodemann, 2014), but it can be hard to disentangle this signal from other drivers (Thurnherr et al., 2020).
For example, the Mediterranean has D-excess values of around 22 ‰, much higher than the global average of 10 ‰ (Gat and Carmi, 1970; Natali et al., 2022) and higher than the Arabian Sea (Jeelani et al., 2017; Jeelani and Deshpande, 2017). However, this is complicated by fractionation. Rain forms preferentially from low-D-excess water, and so D-excess increases in moisture in air parcels that have been transported a long distance, orographically lifted, or even locally recycled (Kong et al., 2013). Ideally, therefore, the results of isotope analysis over the western Himalaya should be disambiguated with a complementary moisture trajectory analysis.
Thus, while recent studies all agree that winter precipitation over the western Himalaya and surrounding region have high D-excess, they disagree on the interpretation of that result. Some have attributed this to moisture coming from the Mediterranean, Black, or Caspian seas, where naturally high D-excess is further increased through fractionation during the long journey to South Asia (Jeelani and Deshpande, 2017), and in some cases, this position has been further supported by basic trajectory analysis (Saranya et al., 2018; Lone et al., 2019; Singh et al., 2019a; Lone et al., 2020, 2023). However, these studies are largely flawed, relying on only short sample periods or applying trajectory analysis either only to case studies or for single seasons.
A few studies have attributed this high D-excess to local recycling, in other words, evaporation from the subcontinent itself (Jeelani et al., 2018; Oza et al., 2022; Joshi et al., 2023). Local moisture recycling as the dominant source is unlikely given the volume required to feed the snowpacks and glaciers and support agriculture, as well as the frequency with which heavy precipitation events occur during the winter months. The most likely source of the majority of moisture is the Arabian Sea, as it is the closest body of water to the western Himalaya, and passing WDs are often associated with southerly moisture flux. It is also the only body of water that can supply enough moisture for extreme precipitation events that are occasionally associated with WDs (Rao et al., 2016). This is supported by a few studies combining isotope analysis with back trajectories (Jeelani et al., 2017; Dar et al., 2021), which argue that moisture from the Mediterranean must also play a significant role, even if it is not the major source of moisture. Dar et al. (2021) estimated that the Mediterranean contributes the majority of moisture in only 20 %–30 % of winter precipitation events over Kashmir.
One possible resolution to these disagreements is that winter precipitation moisture sources vary significantly as a function of location – for example, the orientation of some valleys may block southerly moisture flux from the Arabian Sea but allow northwesterly moisture flux from the Caspian Sea – and due to intraseasonal variability of large-scale conditions (Sect. 2.6) – for example, WDs situated further north are less effective at extracting moisture from the Arabian Sea (Baudouin et al., 2021), a feature controlled by variations in latitudinal position of the subtropical jet.
By focusing solely on Lagrangian back-trajectory analysis, studies can be freed of this constraint, as trajectories can be initialised at any location and any altitude, rather than being confined to a particular observation point or catchment. Hunt et al. (2018c) used reanalysis data to compute Lagrangian back trajectories to determine the moisture sources of heavy winter precipitation events over northern India and Pakistan. They also concluded that the Arabian Sea was the major source, with smaller contributions from the Caspian and Mediterranean seas. An updated version of their analysis is shown in Fig. 9, where particles are released at selected altitudes above heavy WD precipitation events and then integrated backwards in time. Particles in or slightly above the boundary layer (1500 m) largely originate from the Arabian Sea or surrounding region and are responsible for the majority of the moisture. Particles released from the mid-troposphere (5000 m) largely originate from near the Mediterranean but are only responsible for a small fraction of the total moisture. The elevation-based dependence for source attribution may be the reason for disagreement in earlier studies. Boschi and Lucarini (2019) also used moisture back trajectories, computed using a modified version of FLEXPART (FLEXible PARTicle dispersion model) (Stohl and James, 2004, 2005). Applied to 20 extreme winter precipitation events over northern Pakistan and Uttarakhand, they found that the main moisture sources were the Red, Mediterranean, and Caspian seas; the Persian Gulf; and the Gulf of Aden.
Beyond isotope and trajectory methods, recent work by Baudouin et al. (2021) highlighted the potential use of Eulerian composite moisture flux analyses in investigating precipitation moisture sources, with the caveat that such analysis only works on seasonal timescales or longer. They identified a mean moisture pathway between the Red Sea and the north Arabian Sea and showed that WDs transiently steer this pathway towards the western Himalaya and surrounding region. Results obtained using this method are very similar to those obtained from large-sample back-trajectory studies (e.g. Fig. 9). These pathways are analogous to the atmospheric rivers that are responsible for winter precipitation and flooding to the west, in Iran (Dezfuli, 2020; Dezfuli et al., 2021; Esfandiari and Lashkari, 2021). Atmospheric rivers have also been explicitly linked to the majority of winter precipitation variability and extremes over the western and central Himalaya (Rao et al., 2016; Thapa et al., 2018; Breeden et al., 2023; Lyngwa et al., 2023), where composite analysis shows circulation that strongly resembles that of a WD. The altitude of these moisture pathways also appears to be important, with the largest moisture transport occurring between 850 and 700 hPa, a higher altitude than usual in the tropics (Baudouin et al., 2020b).
Both isotope and trajectory methods are useful, but each have shortcomings that mean it is better to draw on results from both methods where possible. Trajectory methods give more precise results for moisture sources, and along-trajectory statistics like parcel humidity can be computed. However, large uncertainties can arise from the representation of orographic and boundary layer processes, both of which are crucial ingredients for WD precipitation. Indeed, the evaporative processes that increase parcel humidity are subgrid processes that are not necessarily well simulated in reanalyses and perhaps call for Eulerian moisture-tracking methods with numerical tracers for different sources to be used in future studies. Further to this, trajectory calculations can be computationally expensive as large ensembles are required to reduce uncertainty. Isotope methods can therefore provide more accurate estimates of moisture partitioning, since they do not depend on small-scale processes being adequately represented by a reanalysis model. However, long time series are required to ensure a representative contribution from all moisture sources. Results from studies that depend on just a single year of data, e.g. Lone et al. (2020) and Dar et al. (2021), must therefore be taken cautiously. Many of the studies discussed in this section use both: typically making a first guess of moisture source using isotope analysis and then supporting their hypothesis with trajectory analysis. Further work is needed with isotopic methods to better distinguish between Mediterranean and local recycling sources, both of which are associated with high D-excess (>20 ‰). Composite moisture flux analysis is more robust to orographic and subgrid processes but by definition does not describe the entire distribution of possible sources, as back trajectories can.
In summary, there is high confidence (robust evidence, medium agreement) from multiple lines of evidence that WDs primarily draw moisture from the Arabian Sea, with contributions also coming from the Mediterranean and Eurasian lakes.
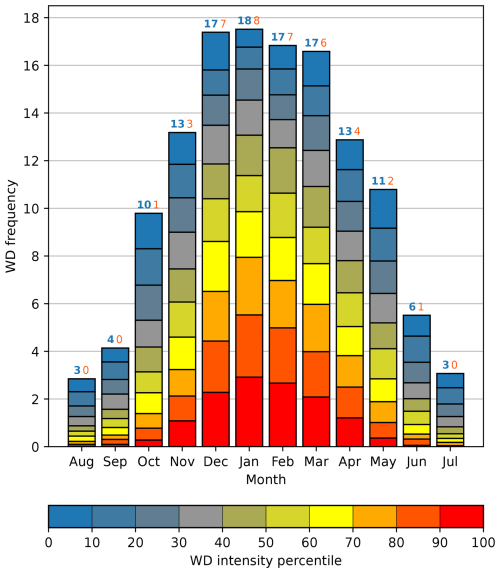
Figure 10The seasonal cycle of WD frequency, computed between 1950 and 2022, following Hunt (2024). Mean WD frequency is given for each month, computed as the number of WDs entering the region (20–40° N, 50–80° E). These are further stratified by overall intensity percentile – based on the full time series – where intensity is defined as the maximum value of 350 hPa ζ that a WD reaches during its lifetime. At the top of each bar, the total WD frequency (rounded to the nearest integer) is given in bold blue and the frequency of WDs exceeding the 80th intensity percentile is given in red. Computed using a WD track database derived from ERA5.
2.5 Seasonality
WDs are often thought of as occurring only during the winter months, but a long list of case studies (e.g. Yadav et al., 2017a; Gupta et al., 2023; Thapliyal and Singh, 2023a) and recorded interactions with the summer monsoon (e.g. Pisharoty and Desai, 1956; Chevuturi and Dimri, 2016; Hunt et al., 2021) show that they can occur at any time of year. In this section, we will quantify that seasonality.
Following Hunt (2024), the seasonal cycle of WDs as a function of their intensity is plotted in Fig. 10. While WDs do occur year-round, they are nearly 10 times more frequent during the winter months (December–March) than during the height of the summer monsoon (July–August). This cycle is controlled almost wholly by the seasonal migration of the subtropical jet (Datta and Gupta, 1967; Hunt et al., 2018b), which typically moves northwards away from the western Himalaya from April to October in response to increased radiative and latent heating of the boreal tropics (Sadler, 1975; Schiemann et al., 2009). Owing to the stability of the jet during the winter months, there is little variation in monthly average WD frequency between December and March (16–18 per month), and this falls only slightly (13 per month) in the extended winter (November and April).
Intense WDs – which we have here taken to be those whose peak vorticity exceeds the 80th percentile of the whole population, in order to match frequency estimates of earlier authors (e.g. Rao and Srinivasan, 1969) – are much more limited to the winter months, appearing only very rarely between May and October. Such intense systems need not only a favourable jet location, but also high upper-tropospheric baroclinic instability, which is uncommon outside of the winter months (Schiemann et al., 2009; Hunt et al., 2018a).
Hunt (2024) also showed that different regions affected by WDs can have different seasonal cycles. During December to March, they predominantly impact the western Himalaya and Hindu Kush. As the jet starts to migrate northward in the spring, these regions are still impacted by WDs, but the northern mountains – the Karakoram and Pamirs – receive considerably more.
Perhaps the most important unanswered question is whether WDs occurring during, or near to, the monsoon have fundamentally different characteristics than typical winter WDs. It is certainly the case that even relatively weak WDs can wreak havoc during the monsoon given the much more plentiful moisture supply, but it is not clear how, if at all, this rectifies onto other characteristics, such as intensification rate and vertical structure. Interactions between WDs and the summer monsoon are discussed in Sect. 3.5.
There is thus high confidence (medium evidence, high agreement) that WDs occur most frequently in the winter months (December to March) but can occur at any time of year, including during the summer monsoon.
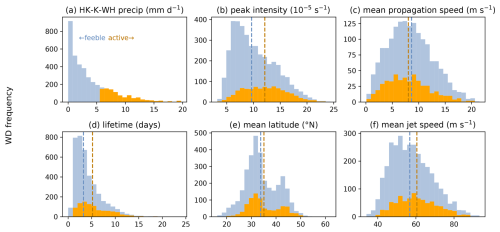
Figure 11Stacked histograms of selected winter (December–March) WD characteristics, drawn from the Hunt (2024) database. (a) Mean daily precipitation averaged over a box including the Hindu Kush, Karakoram, and western Himalaya (30–40° N, 70–80° E); for each WD passing between 60 and 80° E, the maximum of these daily means is taken. (b) The peak intensity – measured as the highest value of 350 hPa relative vorticity recorded at the WD centre as it passes between 60 and 80° E. (c) The mean zonal propagation speed of each WD between 60 and 80° E. (d) The total lifetime of the WD. (e) The mean latitude of the WD centre as it passes between 60 and 80° E. (f) The mean zonal wind speed of the subtropical jet – computed along the jet axis between 50 and 80° E – while the WD is between 60 and 80° E. “Active” WDs (orange) are defined as being in the upper quartile of the precipitation values given in panel (a). On each subplot, the mean of the active and feeble WD distributions are given by vertical dashed orange and blue lines respectively. All data from ERA5.
2.6 Variability
Having discussed the mean state of WDs, we turn now to their variability. As noted in the Introduction, many estimates of WD characteristics come with wide error bars and thus merit more thorough investigation. Figure 11 summarises the variability of six key WD characteristics, based on the database described in Hunt (2024). Distributions for three of the features chosen here – precipitation, lifetime, and intensity – are also shown in Qiu et al. (2022), with which they agree closely on mean, variance, and skew.
There is no formal definition of “active” and “feeble” WDs, despite common use – especially of the former – in literature. We therefore follow consensus usage, that active WDs are responsible for heavy winter precipitation and define them thus: consider mean daily precipitation averaged over box (30–40° N, 70–80° E) that covers the Karakoram, Hindu Kush, and western Himalaya; active WDs are then those that cause that precipitation to exceed the 75th percentile of the distribution associated with all winter WDs. The area-averaged precipitation values have a lower bound of about 5 mm d−1 for active WDs, broadly consistent with observations in Singh et al. (2019b). Although feeble WDs are only associated with light precipitation, they are sufficiently frequent that they comprise a large fraction of the total seasonal precipitation (Riley et al., 2021). This leaves 25 % of the WD population defined as active WDs, a relatively small number, but consistent with earlier definitions (Mohanty et al., 1998; Dimri, 2006).
Peak WD intensity (Fig. 11b) varies considerably, with 350 hPa relative vorticity1 ranging between 5– s−1. Very few active WDs have intensities at the lower end of the distribution and as such have a higher average peak intensity ( s−1) than feeble WDs ( s−1). As with other types of cyclone (e.g. hurricanes; Emanuel, 2000), the intensity distribution is strongly right-skewed.
Mean WD propagation speed (Fig. 11c) also varies considerably, with systems ranging from being almost stationary up to a top speed of about 20 m s−1. The majority of WDs have speeds between 6–12 m s−1, in agreement with the values given in early literature (Datta and Gupta, 1967; Rao and Srinivasan, 1969; Subbaramayya and Raju, 1982). The mean propagation speed of 8 m s−1 (∼7° longitude per day) varies little between active and feeble WDs, with the latter moving slightly quicker, in agreement with Chand and Singh (2015) and Baudouin et al. (2021).
While the full distribution of WD lifetimes (Fig. 11d) ranges from 2–20 d, almost all systems live for between 3 and 10 d, in general agreement with earlier literature on the topic (Datta and Gupta, 1967; Rao and Srinivasan, 1969; Chattopadhyay, 1970; Subbaramayya and Raju, 1982). Active WDs are significantly longer-lived on average (5 d) than feeble WDs (3 d), giving them a longer time to intensify and draw more moisture into the region.
WD latitudes (Fig. 11e) have a bimodal distribution, as we saw in Sect. 2.3.3. They are generally concentrated around a mean value of 35° N but can vary from as far south as 20° N to as far north as 50° N. These latitudes constitute a wide range of conditions, not only thermodynamically, but also in terms of orography and moisture availability, leading to varied WD behaviour and impacts (Baudouin et al., 2020b, 2021).
The mean jet speed (Fig. 11f) associated with active winter WDs is about 60 m s−1, and it is about 4 m s−1 slower for feeble WDs. The value varies from about 40 m s−1 to rare instances of over 80 m s−1. Notably, this is much faster than the WD propagation speeds, implying that WDs travel according to the group velocity, not the phase velocity, of waves within the subtropical jet.
Very little research has covered the intraseasonal variability of WD frequency, as most research focuses on the interannual variability (see Sects. 4 and 7.2). Therefore, quantifying and understanding variability on this timescale remains an important research topic. Two recent studies have published WD databases with monthly counts, from which we can estimate intraseasonal variability. Dimri et al. (2023), drawing their database from IMD weather reports, have a mean WD frequency of 18.3 per season (December–February) over India, with an average monthly range of 2.7 – in other words, for a given season, the average difference between the months with the highest and lowest WD frequencies. For the same metrics, the database from Hunt (2024) gives 52.9 WDs per season, with an average monthly range of 4.7. This falls to 10.1 per season with an average monthly range of 3.3 if we use the same definition of active WDs as above. Crucially, this suggests that the ranges of monthly WD frequency within a given season are not particularly sensitive to the choice of tracking or detection metric, even if the mean frequency is.
In summary, WDs are highly variable across all of their key characteristics (precipitation, intensity, speed, lifetime, latitude). This multidimensionality is seldom reflected in composite or case study analyses of WD behaviour and demonstrates a clear need for a formal classification scheme (such as the categories of North Atlantic hurricanes). Some attempts have been made, either clustering on dynamics and precipitation (Hunt et al., 2018b) or setting thresholds for upper-tropospheric vorticity (Qiu et al., 2022) or wind speed (Javed et al., 2023), but neither has been adopted in wider literature or forecasting. The question thus remains on what the best way to categorise or classify WDs is – impacts (precipitation, location) or dynamics (vorticity, wind speed)?
There is high confidence (medium evidence, high agreement) that WDs exhibit high variability across a range of their characteristics, including intensity, precipitation, latitude, propagation speed, and lifetime.
3.1 Summary of earlier research
Precipitation has long been identified as the main impact of WDs (Veeraraghavan and Nath, 1989; Yadav et al., 2012; Dimri, 2013). We have already discussed how recent advances help to better understand the relationship between WD characteristics and precipitation (see Sect. 2.3.2 and 2.6). In this section, we more broadly explore the characterisation of WD impacts and how recent research has more robustly linked WDs to their associated hazards.
WDs have long been known as the link between large-scale forcing and winter precipitation over the Himalaya, with subseasonal and seasonal precipitation anomalies linked to changes in subtropical jet latitude (Schiemann et al., 2009) and upper-level kinetic energy flux convergence (Yang et al., 2002). However, not all WDs are associated with precipitation of severe intensity (otherwise known as active WDs). Dimri (2006) estimated that only a third of WDs are active (two to three out of six to seven per month during the winter); Mohanty et al. (1998) estimated an even smaller number (four to six per season).
Part of the challenge lies in the accurate measurement of precipitation over the western Himalaya. Precipitation here has high spatial variability because of the complex orography (Andermann et al., 2011), but measuring it is difficult because the gauge network is sparse due to the largely inaccessible terrain (Rajeevan et al., 2005; Kidd et al., 2017) and because gauges have large uncertainty (and can even be buried) when measuring snow (Strangeways, 2010; Rasmussen et al., 2012; Cullen and Conway, 2015). Precipitation estimates from satellites also have high uncertainty not only because passive microwave retrievals perform poorly over snow cover (Ferraro et al., 1998; Tian et al., 2009), but also because the interaction between WDs and orography can produce very heavy local stratiform precipitation (Veeraraghavan and Nath, 1989), and so precipitation rate is not necessarily well correlated with cloud-top temperature. Reanalyses may prove to be useful, as long as they have sufficiently high resolution to accurately capture the orographic convergence that intensifies WD precipitation (Dimri and Niyogi, 2012). WD precipitation is also dependent on underlying land-use characteristics (Semwal and Dimri, 2012; Thomas et al., 2014).
We will also consider advances in the understanding of the role of WDs in water security, showing how recent studies have better quantified how WDs impact the Himalayan water cycle. They are an essential part of this water cycle, even outside winter, since spring snowmelt contributes up to 40 % of Indus discharge and up to 20 % of Ganges discharge (Ramasastri, 1999). Snowmelt also forms a small but important contribution to the discharge of the Brahmaputra River (Hill et al., 2020). This spring runoff is particularly important for agriculture (Biemans et al., 2019), but an abundance can lead to pre-monsoon flooding and landslides (Agrawal, 1999; Thayyen and Gergan, 2010). In the winter months, snowfall from WDs also helps to replenish glaciers (Benn and Owen, 1998; Bolch et al., 2012).
When additional moisture is injected into the WD environment, through extension of the summer monsoon trough (Chevuturi and Dimri, 2016) or through the presence of tropical depressions or tropical cyclones (Kalsi and Halder, 1992), serious flooding can occur. This was the cause of the 2013 Uttarakhand floods, the precursor meteorology of which has since been extensively studied (e.g. Singh and Chand, 2015; Joseph et al., 2015; Ranalkar et al., 2016; Vellore et al., 2016; Houze et al., 2017).
There are several different pathways through which WDs can interact with the monsoon or tropical cyclones. Kalsi and Halder (1992), expanding on Rao (1976), identified four ways in which WDs can interact with tropical air masses: (i) intensification or initiation of tropical depressions, (ii) enhancing precipitation within existing tropical depressions, (iii) causing tropical depressions to recurve northward, and (iv) triggering breaks in the monsoon. Using satellite data, Kalsi and Halder (1992) examined seven case studies of WD–tropical depression interaction and found that these interactions could occur before, during, or after the monsoon, often leading to distortions in the tropical depression cloud field and heavy precipitation. They also found that immature depressions could be intensified by WDs but could also be weakened by the vertical wind shear associated with WDs. In one case, they observed that outflow from a tropical cyclone affected a WD. This research highlighted the need for further investigation of WD–tropical cyclone and WD–monsoon interactions, which would be important for understanding the full range of impacts these storms can have on the region.
Finally, we will look at how recent research has linked other natural hazards to WD activity. Historically, such hazards – including landslides and avalanches, lightning, and fog – have only been anecdotally associated with WDs (Rangachary and Bandyopadhyay, 1987; Ganju and Dimri, 2004; Srinivasan et al., 2005; Das et al., 2006; Thayyen et al., 2013), as have cold waves (Bedekar et al., 1974; Samra et al., 2003; De et al., 2005). However, recent improvements to cataloguing both WDs and hazards means that a more frequentist approach can now be taken.
3.2 Precipitation
3.2.1 Evaluation of precipitation datasets
The quality and variety of precipitation datasets over the western Himalaya and surrounding regions has improved remarkably over the last decade, due to improvements in satellite retrieval, higher-resolution reanalyses with improved parameterisations, and increasing availability of gauge data.
Baudouin et al. (2020a) completed a comprehensive intercomparison of 20 gridded precipitation datasets (satellite, reanalysis, and gridded gauge), including the recently published ERA5 and MERRA-22 reanalyses, over the Indus River basin. Using a cross-validation technique to determine whether sources of variability were internal (e.g. bias within a single dataset or common methodology) or external (e.g. due to WDs or the diurnal cycle), they showed that reanalyses capture daily and seasonal variability well, which has useful implications for regions where gauges are sparse or do not undergo rigorous quality control. They found that gauge products tend to underestimate winter precipitation, which may be due to limitations in detecting snowfall (Strangeways, 2010; Dahri et al., 2018); even so, they concluded that of all datasets, APHRODITE3 (Yatagai et al., 2012, 2017) best captured the daily and intraseasonal variability. Nischal et al. (2022) undertook similar work for winter precipitation over the western Himalaya, focusing particularly on the newly released regional reanalysis, IMDAA (Rani et al., 2021). They showed that, compared with 14 other datasets, the IMDAA reanalysis captured spatial variability well over the region but exhibited a significant wet bias over the western Himalayan orography.
Some studies have also compared gridded precipitation datasets against individual station gauges to try and make the verification more direct, although this often relies on the unsafe assumption that the gauge data are themselves error-free. Hussain et al. (2017) found elevation-dependent positive biases at higher altitudes in both TRMM (Tropical Rainfall Measuring Mission) and APHRODITE when compared with 27 gauges over the Karakoram and Hindu Kush, though they noted this could be due to gauge undercatch. Kanda et al. (2020) compared seven gridded datasets from different sources against 19 station gauges over the northwest Himalaya and found a consistent dry bias, seemingly at odds with Hussain et al. (2017). However, six of the seven datasets (excepting ERA-Interim) strongly agreed with each other, suggesting that the gauge data were perhaps improperly processed before analysis. Nageswararao et al. (2018b) found a very good agreement between 26 gauges over northwest India and both APHRODITE and the IMD 0.25° × 0.25° gridded gauge precipitation dataset, particularly on interannual timescales. However, this argument is somewhat circular, since these gauge data are used to produce both APHRODITE and the IMD dataset. Similarly, Elahi et al. (2023) reported good similarity between gauges in the southern Hindu Kush and gridded-gauge (APHRODITE) and satellite-based (MSWEP – Multi-Source Weighted-Ensemble Precipitation) precipitation datasets.
3.2.2 Contribution to winter precipitation
Neal et al. (2020) derived 30 weather regimes over India using k-means clustering on low-level winds and surface pressure. Four of these regimes were linked to WDs activity, all of which resulted in heavy precipitation over northwest India. Three of the four WD regimes also resulted in precipitation over northeast India, but it is not known whether this arises from downstream remnants of WDs or some other phenomenon.
Midhuna et al. (2020) collated the dates of 582 WDs from IMD weather reports published between 1987 and 2016. They found that days on which a WD was present over the region accounted for over 80 % of the December–March precipitation over the western Himalaya and over 90 % over some parts of central and southern Pakistan; however, they recorded a correlation coefficient of only 0.4 (i.e. explaining about 20 % of the variance) between monthly precipitation and number of WD days over the western Himalaya, likely due to the secondary role of WD intensity. These contribution values were somewhat higher than given in Hunt et al. (2019a), who used a more restrictive attribution method – only counting rainfall occurring within 800 km of a tracked WD – to show that WDs were responsible for about 55 % of winter precipitation over the western Himalaya and surrounding region and over 70 % in central Pakistan, Rajasthan, and Uttar Pradesh. A similar number, 65 %, was reported for the Karakoram by Javed et al. (2022).
These results suggest that the true value of the seasonal winter precipitation contributed by WDs is likely to be somewhere between the values stated by Hunt et al. (2019a) and Midhuna et al. (2020), but it is certainly a majority. The uncertainty likely arises not from the choice of attribution radius – to which attribution fraction has been found to be relatively insensitive for other types of system (e.g. Hunt and Fletcher, 2019) – but from the method used to detect WDs. This is because the other main source of winter precipitation in this region is cloudbursts – very intense but short-lived thunderstorms that drive highly localised extremely heavy precipitation. Cloudbursts are either triggered by WDs or occur independently on other days (Singh and Thapliyal, 2022; Dimri et al., 2023), and so almost all precipitation on WD days arises from the environment created by the WD. What remains less clear, however, is the fraction of precipitation variance across different timescales for which WDs are responsible. On seasonal timescales, Baudouin et al. (2021) estimated WDs are responsible for 70 %–90 % of precipitation variance. There is also evidence that this is probably a large majority on intraseasonal timescales: firstly, because WDs are responsible for most of the seasonal total and their frequency and intensity varies substantially between different years (Sect. 2.6); and secondly, because WDs are responsible for 90 % of extreme winter precipitation events over the western Himalaya and northern Pakistan (falling to only 20 % in the summer). Further work is needed to quantify this explicitly and in so doing validate results from climate and paleoclimate studies that infer changes in WD activity directly from precipitation.
The relationship between WDs and seasonal mean precipitation can be corroborated using isotopic analysis of precipitation over the western Himalaya: Jeelani and Deshpande (2017) showed that WD precipitation contains a higher deuterium excess (D-excess; 20 ‰), a strong indicator of moisture source (Dansgaard, 1964), and therefore that (a) it has a different moisture source to precipitation occurring during the Indian summer monsoon (ISM) and (b) it is the dominant source of precipitation over Kashmir Valley. Maurya et al. (2018) conducted similar analysis on high-altitude snowpack and glaciers over the western Himalaya and came to the same conclusion. Moisture source studies are discussed in greater detail in Sect. 2.4).
There is therefore low confidence (medium evidence, low agreement) in the fraction of winter precipitation that WDs provide to the western Himalaya and surrounding regions, but there is high confidence (medium evidence, high agreement) that it is at least 50 %.
3.3 Water security
Water security and hazards are what make WDs relevant both in everyday life and in future climate scenarios (Mukherji et al., 2019; Kulkarni et al., 2021). In bringing the majority of winter precipitation and the majority of heavy and extreme precipitation events to the western Himalaya and northwest India, WDs are a vital component of water security in these regions. Better knowledge of WDs is thus directly beneficial for decisions around water and disaster management in the western Himalayan region and downstream (i.e. in the Indus and Ganges basins), such as reservoir management, irrigation timing and intensity, efficient water distribution, and disaster mitigation strategies. Advances in these areas also require better observations, forecast skill (particularly on subseasonal and seasonal timescales), and decision-support tools.
3.3.1 Glaciers
As the primary source of precipitation in the western Himalaya, WD activity is strongly linked to glacier growth and ablation. Maurya et al. (2018) analysed δ18O and δ2H excess in glacial meltwater in Punjab and found that a significant fraction of the water originating from these glaciers was attributable to WDs. In an 11-year snow budget of the Chhota Shigri Glacier in the Pir Panjal Range, Mandal et al. (2022) found that the majority of winter precipitation came from WDs. Similarly, a climatological study by Javed et al. (2022) found that WDs supply about 65 % of all snow falling on glaciers in the Karakoram. There is also evidence that WDs play an important role in sustaining central Himalayan glaciers (Sundriyal et al., 2018). See Sect. 7.2.2 for a discussion on how recent trends in WD behaviour have affected glacier mass balance.
3.3.2 Water supply
In the highlands, WD-triggered precipitation replenishes the glaciers and snowpack (Gurung et al., 2017), which in turn recharges the rivers – about two-thirds of the mean annual discharge of the Indus River comes from spring and summer snowmelt (Bookhagen and Burbank, 2010; Biemans et al., 2019) – and replenishes both natural and artificial reservoirs during the spring and early summer (Das and Meher, 2019). The relative contribution of glaciers and snow to the spring meltwater is highly sensitive to location: analysing δ18O and δ2H in the Sutri Dhaka Glacier basin in the western Himalaya, Singh et al. (2019a) found that downstream river discharge there comprised 80 % ice melt and 20 % snowmelt. Recent isotopic analysis has estimated that 70 % of all water discharged by the Indus River comes from precipitation associated with WDs (Jeelani et al., 2021). Similar contributions have been found for other basins in the northwest Himalaya (Lone et al., 2022a; Nabi et al., 2023).
Given that much of the precipitation associated with WDs is solid and serves as seasonal storage, winters with a dearth of WD activity can be particularly devastating. Basu et al. (2017) attributed crop-hampering low rains in November and December 2015 to an anomalously low frequency of WDs, which they associated with strong teleconnections with the El Niño–Southern Oscillation (ENSO) and the North Atlantic Oscillation (NAO; see Sect. 4 for further discussion). In fact, a prolonged weakening of WD activity can cause so-called “flash droughts”. Defining flash droughts as events when soil moisture falls from the above the 40th to below the 20th percentile (defined for each pentad) in a period of less than 20 d, Mahto and Mishra (2020) showed that the Himalaya experienced more flash droughts outside of the ISM than during it and attributed this to fluctuations in WD activity.
There is thus very high confidence (robust evidence, high agreement) that WDs play a vital role in recharging glaciers and snowpack during the winter months and are thus crucial for water security in the Indus and Ganges River basins.
3.4 Other natural hazards
Aside from heavy precipitation and droughts, WDs have been associated with a variety of other natural hazards, including crop damage, fog, cold waves, lightning, hailstorms, avalanches, and landslides. Such hazards can have wide-reaching consequences, including increasing the risk of road traffic accidents (Hammad et al., 2019). In the following subsections, we discuss recent developments linking WDs to each of these hazards.
3.4.1 Crops and flora
In the lowlands, WDs provide the rainfall needed to support the rabi crop, upon which the region is highly dependent (Usman et al., 2018). In bringing cold weather and snowfall to higher elevations, WDs can also have profound effects on local plant life at both weather and climate timescales. Omesh et al. (2015) explored the flowering dates of 46 tree species in the Terai region (foothills of the central Himalaya) in 2014 and 2015 and linked the later flowering dates in 2015 to increased WD activity bringing colder weather to the region. Rashid et al. (2020) highlighted a more extreme example, where intense WDs in the autumns of 2018 and 2019 brought snowfall that decimated Kashmiri apple orchards. Using newspaper reports, Bhat et al. (2024) showed that hailstorms, predominantly associated with WDs occurring between April and June, cause significant damage to agricultural land and fruit orchards in Kashmir, reducing productivity in these sectors by as much as 70 %. In all of these cases, these events occurred at the edges of the winter season, and highlight the susceptibility of Himalayan flora to any changes in the seasonality of WDs. There is already some evidence that such a change is occurring (Valdiya, 2020), and, as we see in Sect. 7.1, this could have disastrous consequences. Indeed, Ravishankar et al. (2015) found that even as far south as Maharashtra, mango crop could be impacted by fluctuations in WD activity.
As we have already seen, WDs are not always destructive to flora, particularly at lower elevations where a constant stream of weaker storms provides the necessary rainfall to irrigate the rabi crop. This positive relationship also sustains the forests of the western Himalaya: Sebastian et al. (2019) found a significant correlation between winter rainfall and forest growth there, which was especially prominent on interannual timescales, highlighting the importance of the contribution of WDs to total annual rainfall in the region. Similarly, Malik et al. (2022) showed that monthly winter precipitation (and hence WD activity) is an important control in the growth of certain conifer tree species in the Hindu Kush. Similar results were reported for the western Himalaya by Chinthala et al. (2022) and for cedars in the Hindu Kush by Bhattacharyya et al. (2023), with the latter arguing that such flora will benefit from increasing WD precipitation (Sect. 7.3.2). The impact of WD activity – predominantly temperature – has been implicated in the flowering time of many species of tree in the lowlands of the central Himalaya (Bajpai et al., 2015).
Bajpai and Kar (2018) quantified the local abundance of Pinus tree pollen deposits in ice blocks taken from three glaciers in the western Himalaya. These glaciers sit well above the tree line, and so the pollen has to be advected there by southerly or southwesterly winds. Combined with the fact that Pinus growth is proportional to winter rainfall, this study neatly highlights how pollen deposits can be used as a proxy for winter precipitation across the western Himalaya and northwest India. This is a tool which we will see leveraged in many studies in our discussion of paleoclimate in Sect. 7.1.
3.4.2 Fog, dust, and pollution
WDs have long been anecdotally associated with fog over northern India (Kundu, 1957), especially over Delhi and the surrounding Indo-Gangetic Plain (Srivastava et al., 2016), where the high density of suitable aerosols is particularly useful as cloud condensation nuclei in fog production, reducing the required supersaturation from around 0.3 % to 0.18 % (Wang and Chen, 2019). Improved observation techniques over the previous decade have allowed these relationships to be more carefully quantified, with studies agreeing that WDs are responsible for about half of winter fog events over north India, and even more so in the foggiest months of December and January (Payra and Mohan, 2014; Sunil and Padmakumari, 2020; Smith et al., 2022). However, only about one-third of WDs passing over Delhi trigger fog there as the presence of a WD alone is not a sufficient condition (Smith et al., 2022), perhaps because weak WDs have little impact at the surface (Sect. 2.3.4).
There are two types of fog associated with WDs, advection fog and radiation fog (summarised in more detail by Sawaisarje et al., 2014; Ghude et al., 2017). Advection fog rarely occurs in winter without a WD present and typically occurs ahead (i.e. to the east) of WDs, where there is southwesterly advection of moist air over cool, wintry ground (Sunil and Padmakumari, 2020). The greater moisture availability means that advection fog is typically thicker than radiation fog. Radiation fog is more commonly associated with the rear sector (i.e. west of the centre) of WDs, where subsidence leads to a stable boundary layer and relatively still and humid conditions. This leads to reduced boundary layer turbulence, either from surface cooling (Patil et al., 2020) or due to descending air masses causing an inversion layer that prevents the downward mixing of momentum. A climatological study of radiation fog events over Delhi by Smith et al. (2022) showed that such events do not seem to be confined to the rear sector and can occur to the east of WDs as well. The reason for this remains unclear.
Fog has increased significantly over north India in recent decades (Hingmire et al., 2019), as much as doubling since 1970 (Srivastava et al., 2016). Most studies agree that this is probably primarily due to increased aerosol loading and urban expansion (Kutty et al., 2020; Smith et al., 2022; Hingmire et al., 2022; Verma et al., 2022). However, WDs may also play a role – a recent reduction in WD frequency, and hence weaker near-surface winds, increased radiative cooling, and reduced precipitation, has also been linked to increased pollution over north India, both in models (Paulot et al., 2022) and observations (Gunturu and Kumar, 2021; Xie et al., 2024; Bharali et al., 2024). Further, as the world warms, WDs are associated with increased moisture flux (see Sect. 7.3), also increasing the likelihood of fog (Verma et al., 2022; Bharali et al., 2024). We discuss in Sect. 7.2 how attributing recent trends to WDs can be challenging, since results are often sensitive to the choice of WD dataset. The influence of WDs on air quality also extends to the central Himalaya – Sundriyal et al. (2018) showed that dust present in the Dokriani Glacier had come from arid regions like the Thar Desert (see Fig. 1) and had likely been advected there by WDs. As such, dust deposition there displayed strong interannual variability. There is observational evidence that the northerlies ahead of WDs can worsen air quality in the western Himalayan lowlands, as dust and pollution is advected from north and central India (Kuniyal, 2015). These studies have all focused on how passing WDs affect aerosol concentrations or fog events, but it is still not known whether there is a relationship in the other direction – i.e. whether regions of high aerosol concentration have a significant effect on WD precipitation. A recent case study by Kemmannu and Manjunatha (2023), for example, suggested that there may be a positive relationship between aerosol optical depth and the precipitation from dissipating WDs over north and northeast India.
In summary, there is strong evidence that WDs affect the frequency of fog events over north India, but the exact nature of the relationship and its driving mechanism are as yet unclear. There is also high confidence (medium evidence, high agreement) that fog events have increased in frequency over recent decades.
3.4.3 Cold waves
WDs can cause cold waves – prolonged periods of below-average temperature – both through cold-air advection by northerlies in the rear sector and through reduced insolation caused by their deep and widespread cloud cover, as well as radiative conditions that favour surface frost (Mooley, 1957). Recent composite studies of WD structure have shown that they are associated with a lower-tropospheric cold anomaly of 2–3 K (Hunt et al., 2018b; Singh et al., 2019b; Midhuna et al., 2020). In case studies, WDs have been associated with considerably more severe cold waves (Gupta et al., 2019). A composite analysis of cold waves over north India by Ratnam et al. (2016) showed that a large subset were associated with upper-level wave activity that strongly resembled a composite WD. Similarly, Sandeep and Prasad (2020) analysed a composite of 21 cold waves between 2000 and 2016, finding they were often associated with a WD-like trough in the upper troposphere. They also provided some statistics: the average duration of their cold waves was 10 d, and the longest – which they confirmed was initiated by the passage of a WD – caused a temperature drop of 4–6 K for 26 d. Athira et al. (2024) separated cold waves into normal and intense (i.e. temperature falling below 2 standard deviations for at least 4 d). Subsequent composite analysis linked normal cold waves to WDs, but the intense cold waves were found to be more commonly associated with omega blocking over Siberia.
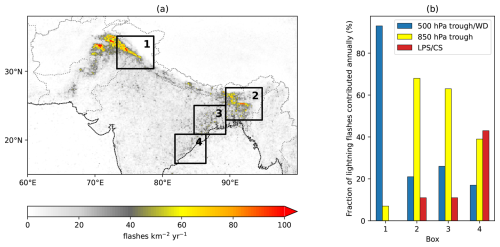
Figure 12Following Unnikrishnan et al. (2021). (a) Annual lightning frequency (flashes km−2 yr−1) in the period 1998–2013, from the TRMM Lightning Imaging Sensor 0.1 Degree Very High Resolution Gridded Climatology (Albrecht et al., 2016). (b) Fraction of lightning strikes in each of the boxes labelled in panel (a) associated with WDs (blue), low-level troughs (yellow), and low-pressure systems or cyclonic storms (red). LPS: low-pressure system; CS: cyclonic storm.
3.4.4 Lightning and hailstorms
Lightning causes a significant fraction of natural hazard-related mortality in India and is most common along the Himalayan foothills (Singh and Singh, 2015; Selvi and Rajapandian, 2016; Yadava et al., 2020). Across Pakistan and northern India, unlike the rest of the subcontinent, the most severe thunderstorms usually occur in the pre-monsoon (March to May), where they lead to many fatalities (Bhardwaj et al., 2017). These storms can also spawn tornadoes (Bhan et al., 2016). Pre-monsoon storms can also occur near the Himalayan foothills in northeast India and Bangladesh, where they are associated with nor'westers, known locally as kalbaisakhi (Roy and Chatterji, 1929; Das et al., 2014). These kalbaisakhi often bring heavy hail and lightning (Midya et al., 2021). The link between nor'westers and WDs remains an open question.
Despite their impact, difficulty in obtaining accurate observations of lightning strikes has resulted in few mechanistic or statistical studies. Unnikrishnan et al. (2021) showed that WDs are responsible for 93 % of lightning strikes in the western Himalaya (mostly over Jammu and Kashmir; see Fig. 12), more than caused by any other type of synoptic-scale weather over any other part of the country. In a similar study, Murugavel et al. (2021) showed that lightning is very frequent along the western Himalayan foothills during the winter and pre-monsoon months, which they attributed to passing WDs. Similarly, a study of hailstorms in Kashmir, compiled from archived newspapers (Bhat et al., 2024), found that most storms occurred between April and June. Victor et al. (2023) argued that the seasonal peak in thunderstorm activity over Kashmir (May to June) was caused by moisture transport from WDs reducing convective inhibition rather than increasing CAPE which can be supplanted by orographic lifting.
Dutta et al. (2016) used a Delhi-based radar to examine four winter hailstorms. Despite a small sample size, they found that those associated with low CAPE and high vertical wind shear (e.g. those associated with WDs) produced smaller hail than those associated with high CAPE and low wind shear (e.g. those associated with cloudbursts). Using 5 years of thunderstorm reports over India, Sharma et al. (2023) showed that WDs occurring before the onset of the summer monsoon (April and May) were often associated with substantial lightning outbursts around the western Himalaya and north India. They argued that this was due to southerly moisture flux associated with WDs passing over the quasi-permanent heat low present over northwest India during these months, leading to large-scale instability. This has been supported by later case studies (Shukla et al., 2022; Gupta et al., 2023), which also highlight the presence of WD-triggered dust storms. A radar-based study by Jaiswal et al. (2023) also showed, for a case study in May 2020, that convection-driven gravity waves associated with WDs may play a role in intensifying lightning and hailstorms.
3.4.5 Landslides and avalanches
In bringing heavy precipitation to large regions of steep orography, WDs present an inherent landslide risk – although this has not been quantified until very recently. Using a global database of precipitation-triggered landslides (Kirschbaum et al., 2010, 2015), Hunt et al. (2021) investigated the synoptic-scale conditions present during 327 landslides that occurred in the Upper Indus Basin between 2007 and 2015. During the extended winter (October to April), such landslides occurred at a rate of about one every 20 d, with 61 % being associated with the passage of a WD. As in Baudouin et al. (2021), they showed that the presence of a WD at the right latitude (30° N) enhances southwesterly moisture flux from the Arabian Sea, resulting in precipitation covering a large area over the Upper Indus Basin, and that the likelihood of a landslide is greatly reduced if the WD is too far north during its passage through the region. Further, they showed that winter landslides in the Upper Indus Basin that could not be linked to WDs were associated with local, smaller-scale, orographically driven cloudbursts. The interaction between a WD and a tropical low brought record-breaking rainfall and widespread landslides to Uttarakhand in October 2021 (Thapliyal and Singh, 2023a).
Similarly, in bringing heavy snowfall to the Himalaya in both the winter and pre-monsoon seasons (and occasionally during the summer monsoon itself), WDs are a potential driver of increased avalanche risk (Hara et al., 2004), especially given most avalanches occur in the western Himalaya between January and May (Ballesteros-Cánovas et al., 2018). So far, there is only an anecdotal link between WDs and avalanches, with a case study by Nischal et al. (2022) citing heavy WD snow as the cause of an avalanche in Uttarakhand in April 2021. Like WDs, fatal avalanches can occur in any season (McClung, 2016). WD-related mountain hazards are thus becoming an increasingly important issue as tourism to the Himalaya grows rapidly (Ziegler et al., 2023), such as in the 2014 Everest avalanche (killing at least 13 people) and the 2014 Annapurna snowstorm (killing 43). Conversely, favourable weather conditions caused by the absence of WDs lead to more successful winter summits of the 8000 m mountains (Szymczak et al., 2021), including the first ever wintertime summit of K2 in 2021 (Matthews et al., 2022).
In summary, recent research has confirmed statistical links between WDs and a range of natural hazards, particularly extreme precipitation events, landslides, fog, cold waves, and lightning. What remains unclear, however, is which WD characteristics – e.g. location or intensity – are most responsible for driving each of these hazards. There is medium confidence (limited evidence, high agreement) that WD rainfall is important for rabi crop growing and high confidence (limited evidence, high agreement) that hailstorms and heavy snow brought by WDs can damage crops. There is very high confidence (robust evidence, high agreement) that WDs provide conditions conducive to widespread fog. There is medium confidence (limited evidence, high agreement) that rainfall and near-surface winds brought by WDs temporarily reduce pollution levels. There is high confidence (medium evidence, high agreement) that some WDs lead to cold waves. There is also high confidence (medium evidence, high agreement) that WDs are the primary source of pre-monsoon lightning across north India. There is medium confidence (limited evidence, high agreement) that landslides in the western Himalaya are frequently triggered by WDs, but only very low confidence (limited evidence, low agreement) that they lead to avalanches.
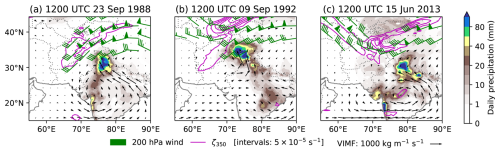
Figure 13Synoptic charts of three cases of severe flooding associated with passing western disturbances: (a) the 1988 Punjab floods, (b) the 1992 India–Pakistan floods, and (c) the 2013 Uttarakhand floods. Charts are shown for the day on which the heaviest daily precipitation occurred. Green barbs show 250 hPa winds (where they are in the top quartile for the domain shown), black arrows show vertically integrated moisture flux, and magenta contours show positive 350 hPa relative vorticity in intervals of s−1, all computed using ERA5 reanalysis (Hersbach et al., 2020). Coloured contours show the daily 03:00–03:00 UTC precipitation, from the APHRODITE gridded gauge product (Yatagai et al., 2012).
3.5 Interaction with the Indian summer monsoon
Extreme rainfall events associated with WDs can be catastrophic. These negative WD impacts most commonly occur near the beginning and end of the ISM, when southward excursions of the subtropical jet result in embedded WDs interacting with moist, monsoonal air masses. Strong southerly moisture flux and large regions of ascending air associated with these WDs create favourable conditions for intense convective and orographic rainfall (Dimri and Niyogi, 2012), which, if sustained, can result in heavy flooding such as that occurring in Punjab in 1988, north India and Pakistan in 1992, Uttarakhand in 2013, and Kashmir in 2014. Figure 13 shows synoptic charts for the first three of these cases, highlighting the role played by WDs (magenta contours), the subtropical jet, and their interaction with the monsoon in causing the precipitation that led to these floods.
WDs are most commonly associated with the winter months, when the subtropical westerly jet is furthest south (Schiemann et al., 2009). However, they can occasionally occur during the ISM (June–September), either due to southward excursions of the jet or as elongated, “digging” troughs or cutoff lows (Kalshetti et al., 2022). Perhaps the most notorious example occurred in June 2013, when a WD interacted with a monsoon low-pressure system, resulting in devastating floods over Uttarakhand (Kotal et al., 2014). Other cases have been noted, with Uttarakhand and Himachal Pradesh seemingly the most common regions impacted (Vellore et al., 2016; Thapliyal and Singh, 2023b; Raghuvanshi and Agarwal, 2024). Majid et al. (2023) showed that floods in the Indus Basin in September 2014 were linked the accumulated effect of several WDs interacting with the summer monsoon. WDs can also interact with tropical storms outside of the monsoon, such as a case in October 2021 which led to record-breaking rainfall in parts of Uttarakhand (Saxena et al., 2023; Thapliyal and Singh, 2023a).
Chevuturi and Dimri (2016) used a high-resolution (3 km), convection-permitting experiment carried out in WRF (Weather Research and Forecasting model) to investigate the meteorological causes of the 2013 Uttarakhand floods in more detail. They found that as the WD and monsoon low-pressure system converged, a sharp frontal region developed between the cold dry air associated with the WD and the warm moist air associated with the low-pressure system, leading to a northwestward extension of the monsoon. These conditions led to convective instability that was supported by deep vertical wind shear associated with the subtropical jet and low-level monsoon easterlies and further enhanced by the orography of the western Himalaya.
The devastating flood in Kashmir Valley in September 2014 was caused by the interaction of a WD with an active ISM trough, leading to record-breaking rainfall occurring over parts of central and north India (Yadav et al., 2017a). This was discussed further in an editorial by Valdiya (2020), which noted that while the southeastward reach of winter WDs seems to have diminished in recent decades, the end of their active season seems to have moved forward from February to April or May. This shift in seasonality was later confirmed by Hunt (2024), as we discuss in Sect. 7.2. In fact, WDs can occur at any time of the year (hence their occasional interaction with the summer monsoon) but are most frequent between November and February (Fig. 10).
Hunt et al. (2021) sought to generalise such interactions, identifying 59 cases of interacting WDs and tropical depressions (TDs) over India and investigating each using synoptic charts, moisture-tracking trajectory analysis, and vorticity budget analysis. They identified four broad types of interaction (summarised in Fig. 14): (i) a vortex merger, where the upper-level PV maximum of the WD and the lower-level PV maximum of the depression superpose to create a single vortex column; (ii) a jet-streak excitation, where the WD causes a downstream jet streak (see earlier discussion) and a depression enters the associated region of ageostrophic ascent to the left of the entrance and intensifies; (iii) a TD-to-WD moisture exchange, where the depression advects moisture towards the WD, intensifying the precipitation associated with the latter; and (iv) a WD-to-TD interaction, the reverse of (iii). They suggested that the 2013 Uttarakhand event was a vortex merger preceded by a TD-to-WD moisture exchange. One shortcoming of this study was the manual identification of interaction types, with the authors highlighting the need for an automatic method for consistent use in operational forecasting or climate model output. Another shortcoming was that the roles of lower- and upper-tropospheric PV maxima were not quantified. Further work might use piecewise PV inversion (e.g. Davis, 1992; Bracegirdle and Gray, 2009) to assess the role of different PV anomalies. Sadaf et al. (2021) showed that these sorts of interactions are a vital source of monsoonal rainfall over Pakistan, estimating that 55 % of summer rainfall is due to interactions between upper-level troughs (i.e. WDs) and monsoon low-pressure systems.
There is very high confidence (robust evidence, high agreement) that the interaction between WDs and the summer monsoon often leads to very heavy rainfall.
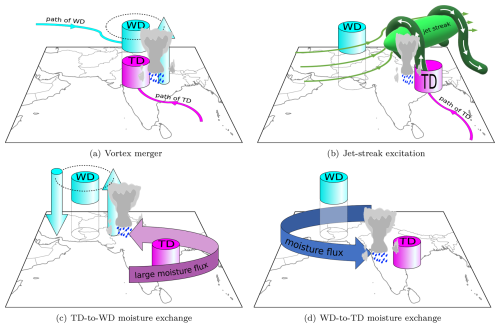
Figure 14From Hunt et al. (2021). Schematics for each of the major types of interaction between WDs and TDs. (a) Vortex mergers occur when the vorticity anomaly associated with a WD superposes that of a TD; (b) jet-streak excitations occur when tropical depressions are stimulated through quasi-geostrophic forcing from a streak entrance region associated with a western disturbance; (c) TD-to-WD moisture exchange occurs when net moisture flux passes from a tropical depression to a western disturbance and (d) vice versa, a WD-to-TD moisture exchange occurs when net moisture flux passes from a WD to a TD.
3.6 A definition of western disturbances
The formal definition of WDs, published by the IMD, is “Weather disturbances noticed as cyclonic circulation/trough in the mid- and lower-tropospheric levels or as a low-pressure area on the surface, which occur in middle-latitude westerlies and originate over the Mediterranean Sea, Caspian Sea and Black Sea and move eastwards across north India.” Much of the research presented in this review shows that this definition4 is now outdated and has led to authors using their own criteria. To aid consistency and reduce ambiguity in future research, we synthesise the research discussed in Sects. 2 and 3 to create a new, up-to-date, unified definition of WDs.
Western disturbances are eastward-travelling upper-level troughs passing over South Asia that arise as instabilities on the jet or as extratropical PV cutoff lows. By drawing moisture primarily from the Arabian Sea, they bring precipitation, and sometimes other impacts, primarily to the western Himalaya, Karakoram, and Hindu Kush, but also to the surrounding regions of Pakistan and north India. They can occur at any time of year but are strongest and most frequent between December and May.
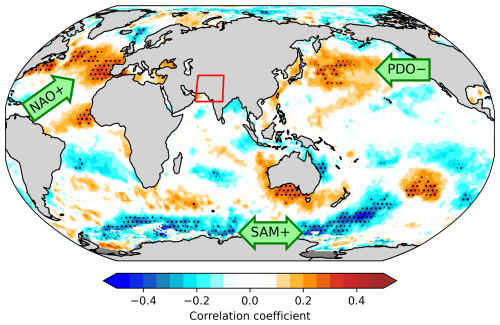
Figure 15Correlation between seasonal mean sea surface temperature (SST) and WD frequency computed for winters (November to March) between 1950 and 2021. Stippling indicates regions where the correlation is significantly different from zero at the 95 % confidence interval. Green labels indicate where large regions of significant correlations correspond to known modes of interannual and interdecadal oscillation, with “+” and “−” indicating positive and negative correlations respectively. Areas of interest are the North Atlantic Oscillation (NAO), Pacific Decadal Oscillation (PDO), and Southern Annular Mode (SAM). The red box marks the region in which WDs are counted, using the ERA5-derived track dataset described in Nischal et al. (2022). SST data are from HadISST (Rayner et al., 2003). Both datasets were detrended before correlation.
4.1 Summary of earlier research
Interest in the teleconnections driving variability in winter precipitation over the Himalaya has only emerged relatively recently and as such has been covered by few studies. Yet, even very simple analysis – correlating monthly WD frequency with SSTs (Fig. 15) – reveals a broad inventory of plausible teleconnections5. Establishing teleconnections with slowly varying modes of variability offers potential improvements to seasonal forecasts of WD frequency and winter precipitation.
Studies have generally agreed that the sign of the correlation between ENSO and seasonal winter precipitation over the western Himalaya is positive (Roy, 2006; Syed et al., 2006; Yadav et al., 2009; Bhutiyani et al., 2010) but typically report weak or insignificant correlations. Competing hypotheses emerged, e.g. a weakening of the Siberian High (Syed et al., 2006), a shift in the jet due to an anomalous Walker circulation (Yadav et al., 2009), and increased moisture supply to the Upper Indus Basin (Mariotti, 2007; Syed et al., 2010), but studies agree that the end result is increased WD activity. Roy (2006) also reported a positive relationship with the Pacific Decadal Oscillation (PDO; an interdecadal mode of climate variability, whose positive mode is associated with cooler SSTs in the western midlatitude North Pacific and warmer SSTs in the eastern North Pacific).
Studies have generally agreed on a positive correlation between the North Atlantic Oscillation (NAO; an interannual mode of climate variability whose positive mode is associated with low sea-level pressure over Iceland and high sea-level pressure over the Azores) and winter precipitation over the western Himalaya that has likely weakened over recent decades (Syed et al., 2006; Yadav et al., 2009; Filippi et al., 2014), with the proposed teleconnection mechanism in each case being an intensification of the subtropical jet leading to increased WD frequency.
As we will see in the following subsections, recent studies have therefore sought to further quantify these teleconnections, but, more importantly, explain the causal processes that support them.
4.2 The North Atlantic and Arctic oscillations
Since Yadav et al. (2009) and Filippi et al. (2014), a number of studies have focused on quantifying or explaining the relationship between the NAO and winter precipitation over India, typically using changes in WDs and the subtropical jet to explain the mechanism. As we will see, these studies generally agree on the sign and significance of the relationship, with exceptions mostly arising due to small sample sizes.
Recent studies have leveraged WD datasets or composite approaches to establish a significant and positive correlation between WD frequency and the NAO (Attada et al., 2019; Hunt and Zaz, 2023) as well as with the Arctic Oscillation (AO)6 (Midhuna and Dimri, 2019). This correlation also holds for precipitation over the western Himalaya, northwest India, and surrounding regions. Studies have identified a significant positive correlation between observed seasonal winter precipitation and the NAO (Nageswararao et al., 2016; Zaz et al., 2019), seasonal winter precipitation and the AO (Midhuna and Dimri, 2019; Zaz et al., 2019; Sanap, 2023), and extreme winter precipitation event frequency and the NAO (Midhuna and Pattanaik, 2023a). Where these studies have investigated causality, all have agreed that a positive NAO or AO increases the upper-tropospheric temperature gradient, increasing baroclinicity – thus resulting in a more intense subtropical jet and more frequent WDs (Attada et al., 2019; Midhuna and Dimri, 2019; Hunt and Zaz, 2023; Sanap, 2023). On longer timescales, Greene and Robertson (2017) found evidence of a link between the NAO and decadal variability of winter and spring precipitation in the Upper Indus Basin in CMIP5 (Coupled Model Intercomparison Project Phase 5) models. There is also some evidence that the strength of the NAO teleconnection is weakening, while the AO teleconnection is strengthening (Nageswararao et al., 2016).
Through its relationship with WDs, the NAO affects many components of the western Himalayan climate system. For example, positive phases of the NAO have been associated with increased rabi crop yield (Nageswararao et al., 2018a) and a reduction in foggy days (Hingmire et al., 2019). In the latter case, the authors linked the reduced fog to increased WD activity, but this interpretation is likely incorrect (Sect. 3.4.2, Sawaisarje et al., 2014; Patil et al., 2020; Smith et al., 2022). This signature also appears in paleoclimate studies, with a positive NAO linked to increased precipitation over the Indus Basin in both fir tree – a type of confiner – cellulose (Chinthala et al., 2022) and Arabian Sea marine sediment paleorecords (Forke et al., 2019).
Even so, some studies have reported a negative or insignificant relationship between the NAO and winter precipitation (Devi et al., 2020; Narasimha Rao et al., 2021a). Basu et al. (2017) attributed a weak winter precipitation in 2015 to reduced WD activity as a result of positive ENSO and positive NAO, stating that the positive NAO set up an unfavourable standing wave in the subtropical jet with a blocking anticyclonic anomaly over the subcontinent. However, that pattern only seems to appear in this particular case and does not hold generally. Several paleoclimate studies have also reported a negative relationship (Munz et al., 2017; Giosan et al., 2018). In attributing wetter winters in the Middle Holocene (4500 to 3000 years ago) to more negative NAOs, Giosan et al. (2018) also noted the subtropical jet was further south during this period, bringing more moisture to the region.
In summary, the relationship between the NAO and WD frequency (and thus also winter precipitation) has been extensively investigated. Almost all recent studies agree that positive phases of the NAO lead to a strengthening of the subtropical jet, increased WD frequency, and hence wetter winters over the western Himalaya. Studies that disagree with this position are uncommon and are either limited to short study periods or are paleoclimate studies with confounding factors, including centennial variability of teleconnection strength.
4.3 The El Niño–Southern Oscillation
The El Niño–Southern Oscillation (ENSO) is a climate pattern defined by anomalous SSTs in the central and eastern tropical Pacific Ocean, varying irregularly with a period ranging from 2 to 7 years. ENSO has a strong effect on the Indian summer monsoon – which is significantly weaker during the positive phase of ENSO (El Niño) and significantly stronger during the negative phase (La Niña) – via modulation of the Walker circulation (Ju and Slingo, 1995; Torrence and Webster, 1999; Turner et al., 2005). However, the relationship between ENSO and the winter weather of India is somewhat less clear, although the rectification of ENSO onto changes in upper-tropospheric temperature gradients over South Asia (Xavier et al., 2007) is a potential pathway.
A number of recent studies have established a positive and significant correlation between ENSO phase and seasonal winter precipitation over the western Himalaya, Karakoram, and surrounding areas (Pal et al., 2015; Nageswararao et al., 2018a; Kamil et al., 2019; Abbas et al., 2023). A positive correlation has also been established between ENSO phase and the frequency of extreme winter precipitation events over the region (Breeden et al., 2023; Bharati et al., 2025). There is still no consensus on the causal pathway, however, with Kamil et al. (2019) arguing for increased WD frequency during El Niño, whereas Bharati et al. (2025) argued that while there is a small increase in WD frequency and intensity during El Niño, the reason for increased precipitation is the southward movement of the subtropical jet bringing WDs closer to their Arabian Sea moisture supply. Even so, there has been some exploration into secondary factors that may affect the relationship between ENSO and winter precipitation. These include the role of different flavours of ENSO, where shifts in the Walker circulation affect the position and strength of the subtropical jet (Dimri, 2017), and the role of stratospheric variability, where the quasi-biennial oscillation (QBO) may additionally modify the Walker circulation and subtropical jet (Shrestha et al., 2019), especially when QBO easterly phases coincide with a sudden stratospheric warming event (Remya et al., 2017).
These results contrast with Basu et al. (2017), who also disagreed with other authors on the sign of the relationship with the NAO. They argued using a case study that the winter drought of November and December 2015 over north India, caused by an anomalously low WD frequency, was partially the result of El Niño warming the Arabian Sea and pushing the subtropical jet further north. Composite analyses by the other studies mentioned here suggest that this interpretation is probably incorrect.
In summary, positive phases of ENSO lead to increased winter precipitation over the western Himalaya, and this relationship may vary in strength according to secondary factors. The casual relationship is not yet well understood.
4.4 The Indian Ocean Dipole
The Indian Ocean Dipole (IOD) describes a pattern of anomalous SSTs in the equatorial Indian Ocean, whose magnitude typically peaks between September and November (Saji et al., 1999; Vinayachandran et al., 2009). A positive IOD event is defined by anomalously warm SSTs in the west of the basin (off the Somali coast) and anomalously cold SSTs in the east of the basin (near the Maritime Continent).
The post-monsoon peak in IOD magnitude suggests that only WDs occurring early in the season (November–December) would likely be affected, with the hypothesis being that a positive IOD event might lead to more moisture availability via a warmer western Indian Ocean. However, very little work has been done on connecting the IOD to WDs or winter precipitation across north India. It is perhaps disregarded as being not only downstream of the region impacted with WDs, but also relatively distant, and whereas ENSO noticeably modulates large-scale dynamics on a global scale, the IOD does not. Pal et al. (2015) included the IOD in a hidden Markov model they devised to understand processes driving winter rainfall in NW India, using observations from 14 stations. Their model identified four regimes: the two associated with precipitation were linked to a zonal wave train across Eurasia, which the authors argued were WDs. Subsequent correlation analysis showed that these modes were more common and more intense in years with a positive IOD or positive ENSO.
In summary, recent studies have established and attempted to explain a wide array of teleconnections between WD frequency and large-scale remote forcings. Studies generally agree that positive phases of the NAO and AO lead to increased WD frequency, and the single study that examines the relationship between the IOD and WD frequency concludes that the correlation is positive here, too. Studies agree on a positive correlation between ENSO and winter precipitation, although different WD mechanisms have been proposed. Furthermore, this relationship may be modulated by ENSO flavour, the QBO, or other secondary influences. No work has yet established a link between WDs and any of the SAM, Madden–Julian Oscillation (MJO), or PDO, and although recent work by Aggarwal et al. (2024) suggested the recent negative PDO may have decreased pre-monsoon (March–May) rains over north India through decreased WD frequency, they did not disentangle this signal from climate change. Despite its distance from the subcontinent, there does appear to be a link between the SAM and the both the East Asian and South Asian summer monsoons (Pal et al., 2017; Fogt and Marshall, 2020), and so a connection with the winter weather of these regions is also plausible.
Therefore, there is high confidence (robust evidence, medium agreement) that WD frequency and intensity increase during positive phases of the NAO but low confidence (limited evidence, medium agreement) that WD frequency increases during EL Niño. There is very high confidence (robust evidence, high agreement), however, that winter precipitation is greater over the western Himalaya during El Niño. There is very low confidence (limited evidence, low agreement) that a positive IOD increases WD frequency.
5.1 Summary of earlier research
The first explicit efforts to model WDs, in which they were initialised in simple numerical weather prediction (NWP) models, showed that even these simple models were able to capture their large-scale structure and propagation (Ramanathan and Saha, 1972; Chitlangia, 1976). Since then, two broad lines of inquiry have emerged, quantifying the respective impacts of improved resolution and physics parameterisations.
Thus far, studies have found that increasing horizontal resolution consistently leads to improved representation of WD behaviour and impacts. At climate model resolutions, this improvement comes from the better-resolved orography – as demonstrated for 90 to 30 km by Dimri (2004) and then down to 10 km by Dimri and Niyogi (2012). At this scale, improvements in resolution have a greater impact on modelled WD precipitation than advancements in convective or boundary layer parameterisation schemes (Dimri, 2006). As resolution increases beyond 10 km, models permit convection to occur explicitly (even if it is not well resolved until kilometre-scale grids), which results in better representation of the interactions between convection and orography (Garvert et al., 2007; Roberts et al., 2009) and a considerable improvement in the simulation of high-intensity WD precipitation (Norris et al., 2015) when compared to models where convection is fully parameterised. Marginal gains have also been observed up to 2.2 km (Norris et al., 2017), and there is evidence that increasing beyond 1.5 km would further allow realistic simulation of orographic updraughts (Kendon et al., 2012), in particular by better simulating mountain crests and wind funnelling, which may be an important feedback in WD–orography interaction (Baudouin et al., 2021).
Studies largely agree that the choice of parameterisation scheme has little impact on WD simulation, except perhaps at very coarse resolutions (Azadi et al., 2002). This is true both for microphysics schemes (Dimri and Chevuturi, 2014a) and land surface schemes (Thomas et al., 2014), although the latter suggested that the choice of land surface scheme may be more important at higher resolutions as the feedbacks between low-level humidity and cloud structure should be better represented. Simulations of WD structure and precipitation are, however, sensitive to initial conditions (Semwal and Dimri, 2012) and choice of data assimilation (Rakesh et al., 2009b, a; Raju et al., 2015).
Three important questions remain unanswered by this earlier research. How good are climate models at representing WDs and their statistics? What can be learned about the structure of WDs and their interaction with the environment from high-resolution convection-permitting models? How important is the choice of convection scheme in simulating WDs? In the next subsection, we will discuss how recent research has tackled these questions.
5.2 New developments
As with previous research, recent developments fall into two categories: (i) testing model sensitivity to the variation of empirical parameters, initialisation datasets, or data assimilation or (ii) evaluating model capability, either in NWP or climate frameworks.
5.2.1 Numerical weather prediction models
Investigating model sensitivity, with the object of improving the representation of the dynamics or impacts of WDs, has remained a popular area of research, particularly in WRF. Patil and Kumar (2016) modelled four case studies in WRF to test the sensitivity of simulated rainfall to different microphysics schemes using a 9 km resolution nested domain with parameterised convection. Even their best-performing experiment showed a southwesterly lower-tropospheric wind bias over the western Himalaya, leading to significant biases in the location of heavy precipitation. They found only a low sensitivity to the choice of microphysics scheme. Sarkar et al. (2019) examined 30 WD case studies and also found that the choice of convection and microphysics scheme had little impact on precipitation biases, with a consistent wet bias over orography. In a parallel study, Sarkar et al. (2020) found that adjusting parameters associated with the boundary layer parameterisation resulted in more realistic humidity and theta profiles in a composite of nine WDs modelled in WRF. Choice of initialisation datasets appears to be much more important than convection or microphysics schemes, as shown by Thomas et al. (2018), who simulated three WD case studies in WRF at 15 km resolution using two different land-use datasets. They found that experiments initialised with AWiFS (Advanced Wide Field Sensor) observations (developed by ISRO (Indian Space Research Organisation)) simulated precipitation significantly better that those initialised with the (somewhat older) USGS dataset.
Recent NWP model evaluation studies have focused on assessing how well extreme precipitation associated with WDs is simulated in various WRF configurations. The key difference with earlier studies of similar focus is increased resolution, which is sometimes sufficiently fine to permit explicit convection. Sarkar et al. (2019), described above, found that the parameterised simulations produced more realistic rainfall and concluded that 9 km was too coarse to turn off convection schemes. Regardless, as we have already seen, studies have shown that WRF can produce fairly realistic heavy WD precipitation even at these grey-zone resolutions. This is because they are still capable of capturing much of the necessary local thermodynamics as well as the synoptic-scale dynamics (Mannan et al., 2017; Patil and Kumar, 2017). Higher-resolution convection-permitting runs improve further on these results, not only due to improved representation of convection, but also due to improved representation of the finer details of the orography, to which the local dynamics can be highly sensitive. Narasimha Rao et al. (2021b) ran two case studies at 3 km resolution to investigate how well WRF, with assimilation, could forecast WD-triggered extreme snowfall in the western Himalaya. They found that their 72 h forecasts simulated the extreme precipitation well, but that the forecasting system benefitted significantly more from 4D-Var assimilation than 3D-Var, highlighting how the former is more sensitive to changes in local stability and circulation. Higher resolutions also permit the evaluation of fog simulations, as Kutty et al. (2021) did for an intense WD-triggered fog event over Delhi that occurred during 15–16 February 2000. Testing a range of resolutions in simulating Himalayan weather for a full year, Karki et al. (2017) showed that convection-permitting resolutions resulted in far better representation of WDs and associated precipitation through better-resolved diurnal and orographic processes.
Apart from WRF studies, Laskar et al. (2015) conducted an in-depth analysis of two intense WDs occurring in March 2015 using data from the IMD operational model, the GFS (Global Forecast System), and local Doppler weather radars. They found that the models underestimated the extreme precipitation associated with these WDs due to a poor representation of deep convection, despite correctly modelling the strong southerly moisture flux from the Arabian Sea. Dutta et al. (2022) further showed that the negative wind bias in these forecasts could be reduced by assimilating wind data from Doppler radars in northern India.
5.2.2 Climate models
Another important aspect of model evaluation is to assess how well WD statistics – including frequency, intensity, teleconnections, and associated seasonal precipitation – are captured in longer model runs. This is important, for example, before using climate models to investigate the response of WDs to climate change (which we cover in Sect. 7.3).
Palazzi et al. (2015b) conducted a comprehensive review of the performance of CMIP5 models in simulating precipitation over the Karakoram, Hindu Kush, and Himalaya. They showed the multi-model mean had a wet bias in each of these mountainous regions in both summer and winter seasons and that most individual models had a wet bias in winter precipitation, consistent with earlier results from Lee et al. (2010) and Su et al. (2013) for global climate models (GCMs) in mountainous or high-elevation regions. Many models also struggled to capture the seasonality correctly. Crucially, they argued, much like Sperber et al. (2013) and Sperber and Annamalai (2014) did for monsoons, that there is no best model or group of models in simulating precipitation over these mountainous regions. These results were extended for CMIP6 models by Meher and Das (2024), who argued that almost all CMIP (Coupled Model Intercomparison Project) models have different strengths and weaknesses in representing the range of mechanisms required to drive precipitation, including from WDs, over the western Himalaya. They identified representation of mid-latitude winds, choice of land-surface dataset, and choice of physical parameterisation schemes as important drivers of model skill. Greene and Robertson (2017) further investigated the interannual and decadal variability of winter and spring precipitation over the Upper Indus Basin in CMIP5 models but found, like Palazzi et al. (2015b), that only a small fraction of the 31 models examined reproduced the seasonal cycle accurately. They showed that in the eight that did, interannual variability was correlated with ENSO behaviour and – to a lesser extent on decadal scales – the PDO and NAO.
Hunt et al. (2019a) applied the tracking algorithm from Hunt et al. (2018b) to the historical experiments (simulating the 20th century) of 26 CMIP5 models. They found that the structure and variability of WDs was generally well captured but that there was a strong dependence on model resolution: higher-resolution models had a narrower jet and correspondingly more intense WDs. They also identified a systematic climatological wet bias for winter precipitation across all models. The authors linked this to positive biases in WD frequency and intensity due to upstream biases in baroclinic instability of the subtropical jet. A similar wet bias was found in a subset of three CMIP6 models by Baudouin et al. (2020b), although they also agreed that the underlying WD dynamics that brought moisture flux into the region were well captured. Ehsan et al. (2020) also found a wet bias in winter precipitation over South Asia in their investigation of 79 members (distributed between six models) of the North American Multimodel Ensemble. They found that the predictability of winter precipitation was weak but showed that expected teleconnection patterns were well captured, suggesting that higher-resolution models may perform better.
Indeed, higher-resolution climate models do perform better: Iqbal et al. (2017) found that models of the CORDEX-SA (Coordinated Regional Climate Downscaling Experiment – South Asia) experiment simulated winter precipitation across the Hindu Kush and Karakoram – most of which is provided by WDs – well. Similarly, Ghimire et al. (2018) evaluated 11 CORDEX-SA experiments and found that precipitation was generally well simulated over the Himalaya, though a slight wet bias persisted over the western Himalaya, and there were elevation-dependent biases elsewhere in the Himalaya.
In summary, recent modelling studies fall largely into two categories: assessing model sensitivity to changes in resolution or parameterisation schemes, or more simply quantifying the skill of NWP or climate models in simulating WDs and their impacts. Recent sensitivity studies have shown there is little, if anything, to be gained by switching or adjusting parameterisations, but they have demonstrated that the choice of the land surface dataset is important. Studies agree that increasing resolution is the most obvious way to improve WD simulation in models. This is reflected in both NWP and climate model evaluation studies, with the coarser resolution of the latter resulting in important biases in moisture transport and precipitation over the western Himalaya. The broad structure of heavy WD precipitation can now be accurately simulated by models with resolutions at the coarser end of the grey zone (∼10 km); however, the complex interactions between convection and orography that modulate finer features of this precipitation need higher-resolution models, ideally with explicit convection, to be resolved.
There is thus high confidence (robust evidence, medium agreement) that simulations of WDs are mostly insensitive to the choice of parameterisation scheme, but also high confidence (medium evidence, high agreement) that the choice of land surface scheme is important. There is very high confidence (robust evidence, high agreement) that WD simulations improve with increased model resolution.
In the next section, we will see how operational weather forecast models perform at predicting WDs over a range of timescales.
6.1 Summary of earlier research
As we saw in Sect. 3, WDs are responsible for high-impact natural hazards including heavy precipitation, lightning, fog, cold waves, and landslides. They are also a key component of water security across the northern subcontinent. This necessitates useful forecasts on a range of scales, from the short term (a few days) to seasonal (several months).
Early forecast models, despite their simple physics and coarse resolutions, were able to predict WD tracks a day in advance (Ramanathan and Saha, 1972) by leveraging the large spatial scale of the subtropical jet and associated WD circulation. Increasing model complexity and finer resolution brought improved forecasts: Dash and Chakrapani (1989) showed that a T21 spectral model with five vertical levels could forecast WD precipitation reasonably well up to 48 h, and Gupta et al. (1999) showed that the NCEP model run operationally by NCMRWF (National Centre for Medium Range Weather Forecasting) (T80, 18 levels) could forecast WD precipitation well up to 72 h. These forecasts suffered from dry biases near and over the Himalaya, driven in part by their inability to resolve the orography. This was also reported in the 1° IMD operational model by Hatwar et al. (2005). It is interesting to note that these dry biases in early NWP forecasts stand in contrast to the prominent wet biases found in CMIP5 models over the same region and season (Sect. 5) – although this could be due to the former simulating heavy precipitation and the latter simulating seasonal means. Das et al. (2003) showed that such forecasts could be improved by dynamical downscaling – in their case using NCMRWF operational forecasts to drive a 10 km nested model. The same method also improved short-term forecasts of high-impact cloudbursts associated with WDs (Das et al., 2006). Developments in data assimilation have also improved WD forecasts, such as by using satellite data to improve estimates of initial conditions (Rakesh et al., 2009b; Dasgupta et al., 2004).
There are thus three prevailing challenges for forecasting. Firstly, although coarser models can forecast WD movement and circulation well, reliable forecasts of their impacts require a much higher resolution – likely convection permitting, as we saw in Sect. 5 – to capture the interactions between convection and the Himalayan orography. Secondly, operational tracking of WDs has only recently become possible, with the recent development of objective WD-tracking algorithms (Sect. 2.2). Before this, forecast verification was largely confined to qualitative case studies, and evaluation of seasonal forecasts, which are essentially used to predict statistics of WDs (e.g. seasonal frequency and the regions likely to be impacted), was particularly challenging. Thirdly, verification of impacts – particularly forecasts of heavy precipitation – is challenging due to the relative sparsity of observations over the western Himalaya, though this has steadily improved over the satellite era.
In this section, we give an outline of the current state of operational forecasts of WD activity at different timescales: short-range (up to about 5 d ahead), medium-range (up to about 15 d ahead), extended-range and sub-seasonal (up to about 30 d ahead).
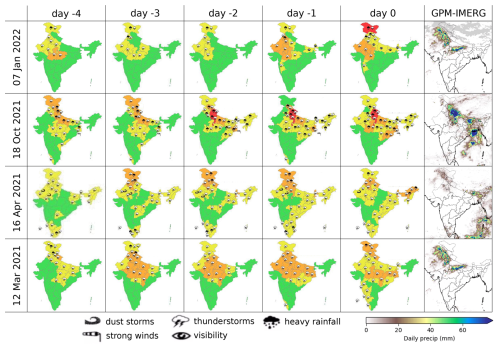
Figure 16Warning bulletins issued by the IMD for four recent dates associated with severe WD weather. For each date, warnings issued up to 4 d in advance are given (first four columns), as well as the warnings issued on the day itself (second column from right) and observed precipitation from GPM-IMERG (last column). The IMD describes the warning colours thus: green – no warning (no action); yellow – watch (be updated); orange – alert (be prepared); red – warning (take action). Areas masked in grey in the GPM-IMERG panels indicate where data are currently unavailable. All bulletins were taken from the official web page of the IMD or their social media (https://internal.imd.gov.in/pages/press_release_mausam.php, last access: 1 January 2025, and https://x.com/Indiametdept, last access: 1 January 2025).
6.2 Short range
There has not yet been an assessment of the skill of short-range operational NWP forecasts in predicting WD tracks or impacts, due in part to the lack of WD-tracking algorithms until recently and the tendency for forecast verification studies over the subcontinent to focus on the summer monsoon. As recent modelling studies have shown that even relatively coarse NWP models simulate the synoptic-scale dynamics associated with the passage of WDs well (Sect. 5), there is little doubt that operational NWPs would produce skilful forecasts of WD tracks several days in advance. However, the lack of systematic analyses remains a major gap in our understanding.
Therefore, in this section, we illustrate the potential value of such analyses with a short case study. As an example, Fig. 16 shows warning bulletin maps released in the 4 d prior to, and on the day of, four recent WDs. Two of these cases resulted in fatalities: the WD on 7 January 2022 led to 22 deaths in Punjab (Pakistan), due to heavy storms and a cold wave; the WD on 18 October 2021 led to 57 deaths in Uttarakhand due to heavy flooding. The skill of the October 2021 forecast warnings is also discussed in more detail in Thapliyal and Singh (2023a).
The following are some preliminary observations.
-
IMD's day 0 warnings, essentially serving as nowcasts, tend to align well with the GPM-IMERG (Global Precipitation Measurement – Integrated Multi-satellitE Retrievals for GPM), especially as these warnings pertain to heavy rain or thunderstorms.
-
Risk forecasts are generally accurate up to lead times of 3–4 d, even in regions with complex orography.
-
However, the general tendency is for warnings to escalate in severity as the event draws closer. While this approach minimises false alarms, rapid escalation of warnings challenges a timely and effective response. This happened in the 7 January 2022 case in Ladakh, where a yellow warning the day before the event was upgraded to a red warning on the day itself.
-
There exists an inconsistency between IMD's high-resolution nowcasts (produced using radar and satellite products; not shown) and their day 0 warning bulletin maps. Such disparities can lead to confusion among stakeholders.
These warnings are derived from deterministic short-range operational forecasts, and so one approach to improve skill may be to use an ensemble forecast – which is often better at capturing extremes – instead (Boucher et al., 2011). However, a full analysis of warning bulletins and nowcasts in the context of WDs is left as an important topic for future work.
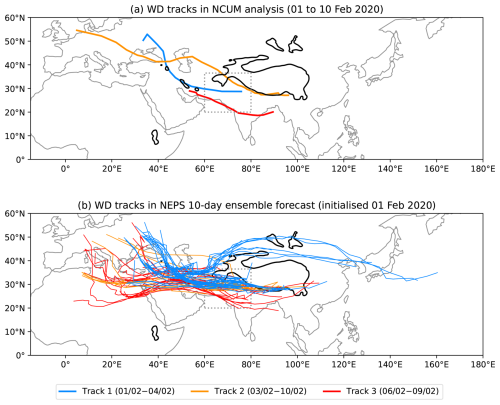
Figure 17Adapted from Arulalan et al. (2020). (a) Tracks of three WDs identified in the NCMRWF analysis between 1 and 10 February 2020. The dotted grey rectangle indicates the capture region, through which tracks must pass to be detected. (b) Tracks of the same three WDs identified in the 23 members (including one control member) of a 10 d forecast of the NCMRWF ensemble prediction system, initialised on 1 February 2020. The thick black line is a smoother 2 km orography contour.
6.3 Medium range
As with short-range forecasting, little work has been done on directly assessing medium-range forecasts in the context of either WD activity or impacts. Arulalan et al. (2020) recently applied the WD-tracking algorithm from Hunt et al. (2018b) to an operational 10 d forecast from the NCMRWF ensemble prediction system (NEPS), as well as the NCMRWF analysis over the same 10 d period (Fig. 17). This is a manual verification of a single ensemble forecast, and so we cannot draw any robust conclusions about the performance of NEPS in forecasting WD tracks. While the tracks are forecast reasonably well in this case, it does highlight some potential challenges for medium-range WD forecasts: the development of spurious tracks (i.e. false positives) and the sensitivity of forecast WD track locations to the relative position of the jet and the Tibetan Plateau. The former problem is not unique to WDs, and we shall not dwell on it further here. The latter is demonstrated by track 1 in Fig. 17 and its analogues in Fig. 17b. Tracks that approach the subcontinent at a latitude south of about 36° N hit the Karakoram or western Himalaya, where the angle of incidence deflects them south into Pakistan and India, where they become WDs. At latitudes above this – about 36 or 37° N – tracks are incident on the Pamirs (see Fig. 1), where they may be deflected south, as before, or north, taking them into Kyrgyzstan and China. This Pamir bifurcation may be sensitive to shifts in the subtropical jet of order 100 km, as well as to the characteristics of the WD itself, presenting a significant forecasting challenge as very similar initial conditions could lead to forecasts predicting either an active WD or no WD at all. This is an important area for future research. This is also compounded by the fact that WDs likely modulate the jet downstream, further degrading predictability of high-impact weather, as has been shown for tropical cyclones undergoing extratropical transition (Keller et al., 2019). An additional issue is the reliability of reanalysis tracks as “truth”. The sensitivity of these results to the tracking algorithm, which could be explored, for example, using the 10-member ERA5 reanalyses ensemble, is also an important consideration for future research in this area.
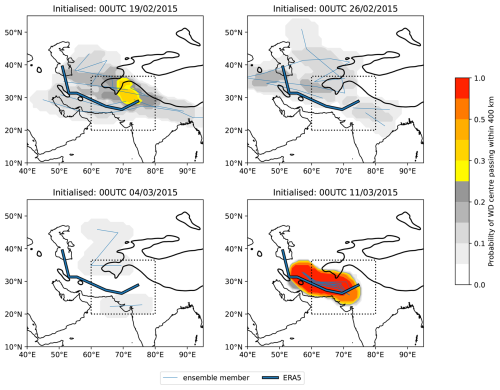
Figure 18Operational forecasts of WD track location from the 16-member IMD extended-range ensemble forecast, for a WD impacting the western Himalaya on 13 March 2015. Each panel represents a different initialisation date, with tracks from the ensemble members given by thin blue lines and the ERA5 track given by a thick blue line. Filled contours represent the probability of an ensemble member having a WD track pass within 400 km between 11 and 13 March. The closest initialisation is in the bottom-right panel (11 March 2015).
6.4 Extended range and subseasonal
Recent work at IMD, summarised here for the first time, has applied a modified version of the Hunt et al. (2018b) algorithm to track WDs in archived forecasts produced by their extended-range forecast system (Pattanaik et al., 2019), with a view to producing such tracks operationally in the future. The modification was required because the forecast output has daily sampling frequency. This included a reduction in the sensitivity of the detection algorithm which mitigates incorrect linkages by increasing the minimum vorticity threshold at which candidate WDs are detected – which in turn reduces aliasing, false positives, and hence incorrect linkages.
Verification has been performed for several of the WD cases listed in Hunt et al. (2020), and here we briefly discuss the results from one selected case (15 March 2015). For this case, IMD operational runs are available with start dates of 11 March, 4 March, 26 February, and 19 February (Fig. 18). Tracks from 16 ensemble members are shown – four members each at two resolutions (T126 and T382) for two models (GFS (Global Forecast System) and CFS (Climate Forecast System)) – for each of these start dates across the four panels of Fig. 18. Only those tracks that are present in the red box between 13 and 17 March are displayed. For verification, we also include the same WD tracked in ERA5, under the same conditions (i.e. using the same modified algorithm, with daily frequency data).
For the 11 March initialisation, all four models predict the track well. However, as we go back through previous start dates, the models diverge, sometimes not producing any tracks at all, although both the 19 February and 26 February initialisations have significantly higher WD activity than in the respective model climatologies.
Midhuna and Pattanaik (2023b) showed that extended-range forecasts of winter precipitation over north India in the NCEP (National Centers for Environmental Prediction) CFSv2 could accurately forecast regional precipitation anomalies as far as 3 weeks in advance, implying that WD tracks and intensities are generally forecast well. These preliminary analyses suggest that more work is needed to produce useful operational forecasts of WDs in subseasonal to seasonal timescales and that a more systematic analysis is needed. One such avenue might be the use of hybrid statistical–dynamical forecasts, such as those already used for the summer monsoon (e.g. Rajeevan et al., 2007), which could leverage additional predictability offered by teleconnections to ENSO, NAO, and other large-scale modes of variability.
There is thus, at present, very low confidence (limited evidence, low agreement) that WD tracks can be skilfully forecast in operational models at any lead time.
One of the most important questions on WDs is how they respond to climate change, and there has been little consensus on this in both earlier and more recent studies. There are, broadly speaking, three lines of inquiry: examination of the paleoclimate record, examination of the historical (i.e. instrumental) record, and use of climate models. As the climate warms, we expect changes to WD dynamics – as inhomogeneous upper-tropospheric warming modifies the subtropical jet. We also expect thermodynamic changes to WDs, as warmer near-surface conditions modify static stability and increase atmospheric moisture content.
The following subsections discuss the extent to which recent studies have been able to leverage longer datasets, the development of tracking algorithms, higher-resolution climate models, dynamical downscaling, and increasing use of advanced paleoclimate techniques to address these uncertainties in the response of WD activity and winter precipitation to changes in past, present, and future climate.
7.1 Paleoclimate proxy measurements and simulations
Paleoclimate research has become increasingly popular over the last few decades, especially as more advanced proxy techniques have been developed and refined. For precipitation, these include speleothems, marine and lake sediments, tree rings, and pollen analysis. As we discussed in Sect. 3.2.2, present-day WDs are responsible for the majority of total winter precipitation over the western Himalaya and surrounding region and likely – through changes in WD frequency and intensity – the majority of its interannual variability as well. For these reasons, precipitation is often used in paleoclimate studies as a proxy for WD activity over the western Himalaya. However, there are several important sources of uncertainty that arise with this approach. Firstly, the relative contributions of winter precipitation (i.e. WDs) and summer precipitation (i.e. the monsoon) to the annual total may change over time. However, this uncertainty can largely be removed by quantifying the D-excess of the sample studied (see Sect. 2.4). Secondly, as mentioned above, some winter precipitation variability must arise from non-WD sources, the primary source of which is cloudbursts. The fraction is unknown but probably small and may also have varied over long time periods. Thirdly, analyses often make do with proxies from winter-precipitation-dominated areas nearby (e.g. Iran, central Asia) and extrapolate the result to the study area (e.g. Petrie and Weeks, 2018). Thus, while we can be reasonably confident that long-term changes in winter precipitation are related to changes in WD activity, we must bear these caveats in mind when discussing the results of the paleoclimate studies that follow.
There are two key reasons to study WDs in paleoclimate: firstly, to understand the impact of external forcing on WDs (e.g. during deglaciation events or Holocene trends); secondly, to characterise the decadal- to centennial-scale variability of WDs and their impact on the evolution of human societies (for which we have two case studies: the Indus Valley Civilisation and the Common Era). For a review of older paleoclimate studies, the reader is referred to Herzschuh (2006), Dimri et al. (2016), and Kar and Quamar (2020).
In this section, we aim to cover the literature approximately in chronological order of the period studied, rather than attempting to group them thematically by proxy type or region, to make the variability over the Late Pleistocene (126–11.7 ka7), the Holocene (11.7 ka onwards), and finally the Common Era (2 ka onwards) stand out more clearly to the reader. Studies based directly on recent observational records (i.e. since about 1900) are discussed in the next section (Sect. 7.2).
7.1.1 Late Pleistocene (80–11.7 ka)
The Late Pleistocene was dominated by cold global temperatures and very low sea levels, which culminated during the Last Glacial Maximum (LGM; approximately 26–20 ka). Of note is that the subsequent deglaciation saw a brief reversal to cold conditions in the Northern Hemisphere (Younger Dryas period, 12.9–11.7 ka)
Mineral analysis of moraines in the Karakoram (Ganju et al., 2018) suggested significant glacial advances at around 60 and 30 ka, with the extent of the latter likely holding until the end of the LGM (18.2±1.8 ka). These advances are associated with periods of increased winter precipitation and colder temperatures, which the authors link to increased WD frequency. In their comprehensive review of the paleoclimate of Ladakh, Phartiyal and Nag (2022) argued that the climate of the NW Himalaya alternated between summer-monsoon-dominated precipitation and WD-dominated precipitation. They argued that the monsoon dominated throughout much of the region in the Late Pleistocene (∼80–35 ka) and that the region was then westerly dominated from 32–17 ka, consistent with the analysis in Ganju et al. (2018). Nag et al. (2023) analysed lake sediments in Ladakh and placed the WD-dominated period slightly later – during the post-glaciation from 19.6 to 11.1 ka. Within this, they identified a particularly wet period from 17.4 to 16.5 ka. Similarly, using fossilised pollen, Kar and Quamar (2020) identified particularly wet winters at the end of the Pleistocene (12.2-11.8 ka), which they attributed to enhanced WD activity, and also agreed with other studies that there was significant variability in both summer and winter precipitation over the western Himalaya since the beginning of the Late Pleistocene.
7.1.2 Early Holocene (12–8 ka)
The Holocene began around 11.7 ka, at the end of the Younger Dryas. The Early Holocene marked significant developments in human civilisation, including in South Asia, as a warmer and more stable climate allowed for the development of agriculture. The period as a whole witnessed a general warming trend and the continued retreat of ice sheets that had expanded during the LGM and Younger Dryas. Studies looking into western Himalayan climate in this period are often extensions of Late Pleistocene studies.
Both Phartiyal and Nag (2022) and Nag et al. (2023) agreed that the summer monsoon dominated the climate of Ladakh throughout almost the whole Early Holocene, with WDs starting to dominate again around the end of this period (8–7.5 ka). This view was not shared in the review by Phartiyal et al. (2022), who argued that the Early Holocene was in fact WD dominated, except for arid periods between 10.8–10 and 8.8–8.6 ka. Kar and Quamar (2020) also supported increased WD frequency during the Early Holocene, but their methodology was unable to clearly differentiate between summer and winter precipitation.
7.1.3 Middle Holocene (8–4 ka)
The Middle Holocene was marked by an increased seasonality in insolation, resulting in colder winters and warmer summers in the Northern Hemisphere as well as significantly reduced Arctic sea ice (Wanner et al., 2008; de Vernal et al., 2013), leading to a weaker Equator-to-pole temperature gradient and hence a weaker winter subtropical jet (Park et al., 2018; Xu et al., 2020). Of particular interest in this period is the so-called 4.2 ka event, an abrupt change in the climate that led to widespread aridity, marking the end of the Middle Holocene. It is speculated that this aridity led to widespread societal collapse across Eurasia and Africa, including contributing to the demise of the Indus Valley Civilisation (Staubwasser et al., 2003; Robbins Schug et al., 2013; Ran and Chen, 2019). This partially explains the large number of studies focused on this period.
Sediment analyses suggest that for most of the Middle Holocene, precipitation over the western Himalaya and surrounding regions was dominated by WDs and that this period was, on average, drier than the present day (Trivedi and Chauhan, 2008; Demske et al., 2009; Leipe et al., 2014; Hamzeh et al., 2016; Phartiyal and Nag, 2022; Phartiyal et al., 2022). These results are supported by model-based analyses, which argue that the drier conditions arose from a significantly weaker subtropical jet (hence fewer WDs) due to greater insolation at higher latitudes causing a shallower meridional temperature gradient (Hunt and Turner, 2019; Kumar et al., 2019d).
There are contrasting views on the impact of the 4.2 ka event on winter precipitation over north India and Pakistan. Although there was consensus among earlier studies agreed that it resulted in a period of increased aridity – i.e. reduced WD frequency – across the western and central Himalaya and surrounding regions, the exact timing of the event has yet to be resolved (Singh et al., 1974; Phadtare, 2000; Staubwasser et al., 2003; Dixit et al., 2014; Leipe et al., 2014; Kar and Quamar, 2020). In their multi-proxy assessment of climate in areas upstream of the Indus Valley, Scroxton et al. (2022) showed that the 4.2 ka event was likely not to have been regionally coherent, although they did find a region-wide drying event at 3.97 ka. They argued that the 4.2 ka event was “transmitted” from the Mediterranean or Middle East and was thus linked to a period of reduced WD frequency. Over the western Himalaya, a range of high-resolution sediment and speleothem studies found strong winter precipitation between 4.5 and 4.3 ka and then a much drier period between 4.2 and 3.97 ka (Giesche et al., 2019; Carolin et al., 2019; Giesche et al., 2023). Following a recovery, there was another dry period across the western and central Himalaya starting in about 3.4 ka and continuing until about 3.0 ka (Joshi et al., 2017; Lone et al., 2022b; Giesche et al., 2023).
The spatiotemporal heterogeneity of the 4.2 ka event identified in Scroxton et al. (2022) may explain why some studies have actually found evidence of increased winter precipitation during this period. Giosan et al. (2018) used plankton fossils as proxies for winter precipitation over India during the later Middle Holocene (4.5–3.0 ka). They found a significant strengthening of winter precipitation and WD activity during this period, contemporaneous with a weaker summer monsoon (Fig. 19). Drawing on other studies, they linked the stronger winter precipitation to southward migration of the jet and a more negative NAO, although the latter is at odds with Forke et al. (2019), who linked increases in runoff in the Arabian Sea since 5 ka to periods of positive NAO and ENSO and found that winter precipitation promptly declined again at the end of this period (3.3–3.0 ka).
In summary, the Middle Holocene was a period of dry winters for the western Himalaya. During the 4.2 ka event, which affected the region for several centuries, some areas experienced much drier winters and low WD frequency, whereas some areas experienced the opposite. This climatic shift may have contributed to the decline of the Indus Valley Civilisation, but there is increasing evidence in both pollen and archaeological records to suggest that they may have simply changed their agriculture techniques over several generations to reflect the new conditions (Bates, 2020; Spate et al., 2022).
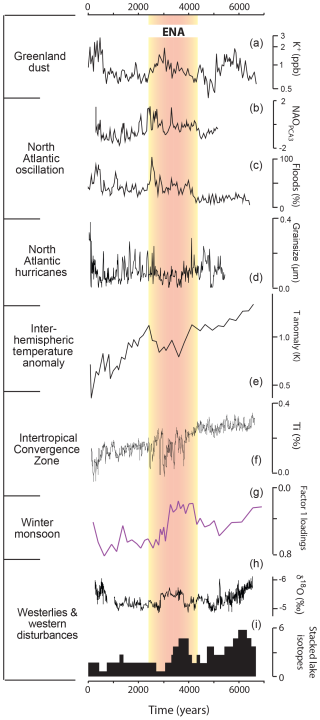
Figure 19From Giosan et al. (2018). Northern Hemisphere hydroclimatic conditions since the Middle Holocene. The period corresponding to the early neoglacial anomalies (ENA) interval is shaded in red hues. From high to low (panels labelled (a) through (i)): (a) Greenland dust from non-sea-salt K+ showing the strength of the Siberian Anticyclone (O'Brien et al., 1995), (b) NAO proxy reconstruction (Olsen et al., 2012) and (c) negative NAO-indicative floods in the southern Alps (Wirth et al., 2013), (d) grain-size-based hurricane reconstruction in the North Atlantic (van Hengstum et al., 2016), (e) interhemispheric temperature anomaly (Marcott et al., 2013), (f) Intertropical Convergence Zone reconstruction at the Cariaco Basin (Haug et al., 2001), (g) winter monsoon ancient DNA-based reconstruction for the northeastern Arabian Sea (Giosan et al., 2018), (h) speleothem δ18O-based precipitation reconstruction for the northern Levant (Cheng et al., 2015), and (i) stacked lake isotope records as a proxy precipitation–evaporation regimes over the Middle East and Iran (Roberts et al., 2011). The present day is on the left, with time going backwards as the x axis increases, as is conventional in paleoclimate studies.
7.1.4 Late Holocene (4 ka–present)
The Late Holocene, which covers from about 4 ka to the present day, has had a generally stable climate with conditions largely similar to the present day. The Common Era (2 ka–present) has been extensively studied due to the ready availability of high-resolution tree-ring records and historical documents. Periods of note in this era include the Roman Warm Period (ca. 250 BCE to 400 CE), the Medieval Warm Period (ca. 900 to 1300 CE), and the Little Ice Age (LIA; ca. 1300 to 1850 CE). The last 100 years, dominated by instrumental records and active weather observations, are left for the next section.
Previous assessments of paleoclimate records have agreed that winter precipitation, and hence WD activity, over the western Himalaya was particularly weak between 4000 and 3500 years ago, following the 4.2 ka event, but disagreed on whether it was weak or strong over the following 2 millennia (Rühland et al., 2006; Mishra et al., 2015; Rawat et al., 2015). However, there is agreement that the intensities of seasonal winter precipitation and the summer monsoon have generally been anticorrelated over the Late Holocene (Kotlia et al., 2012; Sanwal et al., 2013; Kotlia et al., 2015). There is also consensus among older studies that winter and spring precipitation across north India and the western and central Himalaya was significantly enhanced during the Little Ice Age (ca. 500 to 200 years ago; Bamzai and Shukla, 1999; Wu and Qian, 2003; Ye et al., 2005). This was due to the subtropical jet strengthening and migrating anomalously far south (Lückge et al., 2001; Brown and Johnson, 2005; Prasad et al., 2007; Newton et al., 2011; Kotlia et al., 2012; Konecky et al., 2013; Böll et al., 2014).
An important recent development is the discovery that winter precipitation across the Himalaya is strongly anticorrelated with summer monsoon strength on centennial timescales, as demonstrated by two studies comparing speleothems and lake deposits from central India and the western Himalaya (Kotlia et al., 2017; Mishra et al., 2018). As we will see, this leads to better understanding of changes in circulation but also means that proxies sensitive to the summer monsoon can be used to learn about winter precipitation and vice versa.
Recent studies based on organic and lake sediment analyses have shown that winters in the Pamirs and NW Himalaya were very warm and dry from 500 BCE–20 CE and then again from about 400–1400 CE (Aichner et al., 2015; Lone et al., 2022b), implying very weak WD activity. These periods coincide almost exactly with the Roman Warm Period and the Medieval Warm Period respectively.
As we have already discussed, the LIA brought a period of significant growth in Himalayan glaciers between 1300 and 1600 CE, slightly earlier than the coldest period of Northern Hemisphere air temperatures Rowan (2017); Mehta et al. (2021). This was in part due to a colder climate over the region but also due to increased precipitation from WDs between about 1400 and 1800 CE. This has been explained through a southward migration of the intertropical convergence zone, which prevented the summer monsoon from reaching much of north India and also allowed for the subtropical jet to pass unusually far south (Banerji et al., 2019). Thapa et al. (2022) suggested, using a model ensemble, that this was due to changes in solar and orbital forcing.
Despite the clear overall impact of the LIA in significantly increasing winter precipitation across the western Himalaya and surrounding regions, recent studies have shown a great deal of variability embedded within this. Decadal-scale droughts in this period appear to have emerged at completely different times in different regions, from the Hindu Kush to the central Himalaya (Shekhar et al., 2017; Yadav et al., 2017c; Shekhar et al., 2018; Ahmad et al., 2020). These studies explain these droughts through WD variability in response to the Arctic Multidecadal Oscillation (AMO) and ENSO. There is general agreement in tree-ring studies that the enhanced winter precipitation associated with the LIA concluded around 1820 CE (Kotlia et al., 2017; Chinthala et al., 2023).
However, several studies, such as the glacial sediment analysis by Bali et al. (2015) and the tree-ring chronology by Yadav et al. (2017b) have argued that moist winters have continued since the end of the LIA, but these may well be regionally confined as studies covering broader areas suggest that the gradual post-LIA warming is likely to have resulted in weakening winter precipitation subject to strong interannual variability (Munz et al., 2017). Using plankton fossils as a proxy for temperature, Munz et al. (2017) quantified western Himalayan winter precipitation climatology with biennial resolution from 1750 CE onwards. They found three intense winter seasons in ca. 1800, ca. 1890, and ca. 1930 and linked them to strong negative NAO events – in contrast to the positive correlation between NAO and winter precipitation intensity that has been found in more recent data (Sect. 4.2). They noted that teleconnections with ENSO and the PDO have weakened over the last century, which they postulated as due to global warming.
We conclude this section on paleoclimate with a broad summary, given in Table 1. Two items are of particular interest. Firstly, the disagreements – notably the timing and regions affected by the 4.2 ka event and the LIA – may indeed be caused by significant heterogeneity in regional responses to large-scale forcings, but as we will see later, such a pattern is not necessarily supported in observations or climate models. Roy et al. (2022) reported similar inter-study discrepancies in their review of western Himalayan climate over the last 2000 years, and these may also be due the difficulty in disentangling impacts from the summer monsoon, which, as we have seen, is often anticorrelated with winter precipitation over north India on centennial timescales. Secondly, the pattern that has emerged over the Pleistocene and Holocene – i.e. a warmer planet leads to drier winters with reduced WDs and vice versa – will be a very useful piece of evidence when it comes to understanding how WDs respond to global warming, as we see in Sect. 7.3.
There is medium confidence (limited evidence, high agreement) that WD frequency was higher than present during most of the Late Pleistocene (60–12 ka) and much lower than present during the Early Holocene (12–8 ka). There is very high confidence (robust evidence, high agreement) that WD frequency was lower than present during the Middle Holocene (8–4 ka) and medium confidence (robust evidence, low agreement) that it was much lower during the 4.2 ka event. There is high confidence (medium evidence, high agreement) that WD frequency was lower during the Roman Warm Period (2.5–1.9 ka) and Medieval Warm Period (1.5–0.7 ka), and there is very high confidence (robust evidence, high agreement) that WD frequency was higher during the Little Ice Age (0.7–0.2 ka).
Table 1Summary of paleoclimate study results from Sect. 7.1, indicating the estimated strength of winter precipitation (and hence WD frequency) over the western Himalaya and the level of consensus among recent studies for selected geological periods.
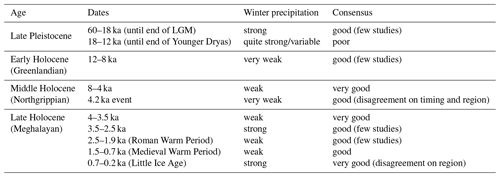
7.2 Instrumental and reanalysis records
Before 2015, only two studies had attempted to count WDs in the historical record (i.e. since the beginning of the 20th century). Both did so by associating station-based precipitation with upper-level troughs, and neither found a significant trend in frequency for their respective choice of season and region (Das et al., 2002; Shekhar et al., 2010). Shekhar et al. (2010) noted that this could therefore not explain the observed decline in snowfall days over the same period. A similar recent decline in snowfall over the western Himalaya was also reported by Gusain et al. (2014) and Singh et al. (2015), with both noting a significant elevation dependence (the observed decline was much stronger at lower elevations). This elevation-dependent snowfall trend is consistent with other mountainous regions across the globe (Pepin et al., 2022). However, accurate measurements of snowfall are challenging to obtain, and so such results must be considered cautiously (Winiger et al., 2005; Palazzi et al., 2013).
There are several methods by which studies have quantified WD variability or trends over the last century or so, and they can broadly categorised as follows.
-
Direct counting using national, state, or station-based reports. This typically involves identifying events where heavy precipitation has co-occurred with an upper-level trough. These are given in blue in Fig. 20.
-
Feature-based tracking in reanalysis data, as discussed in Sect. 2.2. These are given in red in Fig. 20.
-
Using filtered variance or clustering of an appropriate meteorological field in reanalysis. These are given in green in Fig. 20.
-
Inferring changes in WD frequency from trends in other winter weather variables, typically precipitation, in the western Himalaya or surrounding region. This is discussed in Sect. 7.2.2.
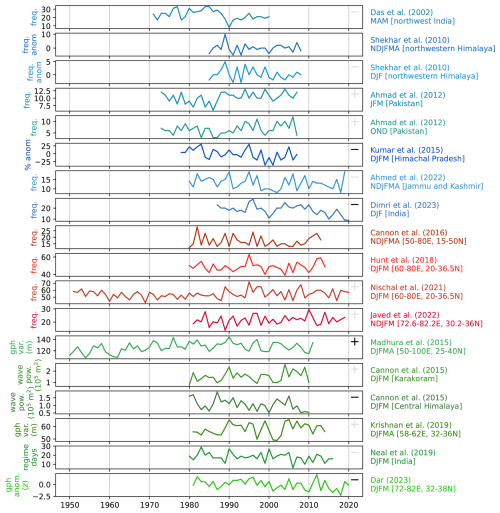
Figure 20Adapted from Hunt (2024). Trends of WD activity since 1950 from the studies discussed in Sect. 7.2 that have available data. For each study, data are presented in the original units, with the stated season and impact region given. Those in blue derive their time series from observational records, such as IMD bulletins; those in red use tracking techniques applied to reanalysis data; those in green use variance-based methods applied to reanalysis data. For each time series, a black +/− in the upper-right corner indicates the trend is significantly different from zero at the 95 % confidence level; a grey +/− is used for the 50 % confidence level. Different shades of blue, red, and green are used only to differentiate between studies.
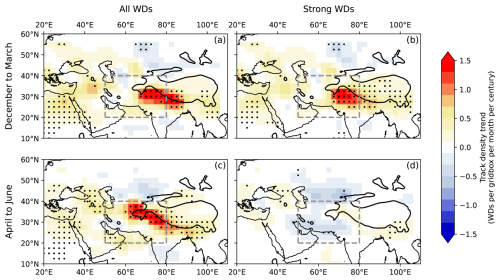
Figure 21From Hunt (2024). Trends in WD frequency from 1950 to 2022. The number of unique tracks passing through each 2.5° × 2.5° grid box per month is counted, aggregated by season, and the trend is computed. Two seasons are shown: (a, b) December to March and (c, d) April to June. To determine whether trends are sensitive to WD detection thresholds, trends for “strong WDs”, i.e. those whose peak 350 hPa ζ is in the top half of the population, are given in panels (b) and (d). Stippling indicates where trends have p<0.05. The dashed grey box (20–40° N, 50–80° E) indicates the region typically used for aggregating WD statistics.
7.2.1 Counting WDs
A handful of studies – shown in blue in Fig. 20 – have leveraged national or local weather reports to aggregate WD counts. These reports typically attach WD status to storms passing over north India in the winter months that are associated with both heavy precipitation and an upper-level trough. Such studies have either reported a decline (Kumar et al., 2015; Midhuna et al., 2020; Dimri et al., 2023) or no trend (Das et al., 2002; Shekhar et al., 2010; Ahmed et al., 2022) in winter WD frequency over India across their study periods, which typically start in the 1970s or 1980s.
The strength of the decline appears to vary depending on the season, with both Ahmed et al. (2022) and Das et al. (2002) showing that the trend in WD frequency often becomes significantly negative at the edges of the season (November and March in the former; May in the latter). However, these studies are thus at odds with Valdiya (2020), who argued for a lengthening of the WD season over northern India – i.e. increased frequency in the spring months. Where declines in WD frequency have been reported, they have often been linked to falling winter precipitation as well (e.g. Kumar et al., 2015; Ahmed et al., 2022). Further west, Ahmad and Sadiq (2012) provided a comprehensive overview of WD frequency and variability, grouped by season and latitude, derived from reports issued by the Pakistan Meteorological Department between 1973 and 2007. They found a significant increase in annual WD frequency over Pakistan but noted it was subject to strong interdecadal variability: a significant negative trend in frequency until 1985 was followed by a significant positive trend. They also found that the sign of the trend was sensitive to latitude: strongly positive in the north of the country but negative in the south.
The WD track databases compiled by Cannon et al. (2016), Hunt et al. (2018b), Javed et al. (2022), and Nischal et al. (2022), based on feature-tracking algorithms (see Sect. 2.2), can also be used to investigate WD trends and variability – noting the usual caveats that apply to using reanalyses to quantify climatological trends. None of these databases, which are shown in red in Fig. 20, contain a statistically significant trend. Javed et al. (2022) investigated WD trends over four adjacent regions – the Karakoram and the western, central, and eastern Himalaya (we use data for the western Himalaya in Fig. 20) – and recorded a steady WD frequency in each. However, they did find a 10 % increase in precipitation intensity over the Karakoram since 1980, which they attributed to an increase in WD intensity driven by increased baroclinic instability. In a follow-up study, Javed and Kumar (2024) also attributed this increased WD intensity and precipitation over the Karakoram to increased moisture availability in recent decades. Nischal et al. (2022) extended the work of Hunt et al. (2018b) to the newer ERA5 and IMDAA reanalyses (at both T42 and T63 spectral truncations). Similarly, they did not find a significant trend in any of their four datasets: the lowest p value, 0.15, applied to a weak positive trend in the T42-truncated ERA5 track frequency – this is the one shown in Fig. 20. Motivated by earlier disagreements, Hunt (2024) used the T42 ERA5 database to explore WD frequency trends as a function of location and season (Fig. 21). They found that winter WDs have increased significantly over the western and central Himalaya and Hindu Kush in the last 70 years, likely due to increasing strength of the subtropical westerly jet (Pena-Ortiz et al., 2013). They also found that due to delayed northward retreat of the subtropical jet, WDs have also become significantly and substantially more frequent in the pre-monsoon and early monsoon. This has led to a large increase in the frequency of potentially dangerous monsoon–WD interactions (Sect. 3.5) and corroborates the earlier work of Valdiya (2020). Hunt (2024) found no significant trend in the intensity of winter WDs, as measured by their upper-tropospheric vorticity, and so the response of WD intensity to climate change remains an open question.
Where authors want to quantify WD frequency (or related statistics, e.g. of their intensity or precipitation) without using a tracking algorithm, they typically turn to using some measure of upper-level geopotential variability. These are shown in green in Fig. 20 and discussed below. For detailed information on the methods used in each of these studies, refer to Sect. 2.2.
Studies using methods based on geopotential variance or anomaly show an increasing trend in WD activity over the Karakoram and decline over the western Himalaya (Cannon et al., 2015; Krishnan et al., 2019a; Dar, 2023), with the latter agreeing with many of the report-based (blue) studies. Those using alternative methods tend to disagree, with Madhura et al. (2015) reporting a significant positive trend in their WD-like EOFs measuring activity over the western Himalaya and an insignificant negative trend in the frequency of the four WD-like weather regimes identified over India by Neal et al. (2020).
In summary, there is disagreement among recent studies on the sign and significance of the trend in WD frequency over the past 70 years. There is thus no confidence in the overall sign of the trend of WD frequency over the western Himalaya in the instrumental record. In other words, there is medium confidence (robust evidence, low agreement) that there is no clear trend in WD frequency or intensity over the instrumental period.
The trend is sensitive to the study region chosen, with studies generally reporting positive trends in frequency over the Hindu Kush, Karakoram, and north Pakistan and generally reporting negative trends elsewhere (south Pakistan, western Himalaya, and India in general). Indices including both regions tend to show an absence of change. The frequency trend is also sensitive to the definition used for the winter season. Not only this, but WD frequency displays significant interdecadal variability (Hunt and Zaz, 2023), so the trend is also sensitive to the dataset period used. There are many competing factors at play, for example, the subtropical jet has strengthened over the last 70 years (Pena-Ortiz et al., 2013; Hunt, 2024), but static stability over the region, an important precursor to deep convection, has decreased (Yuval and Kaspi, 2020). Finally, caution must be exercised when intercomparing these studies. While some directly compute trends in WD frequency, others use different WD characteristics, such as geopotential variance, which is a function of both frequency and intensity.
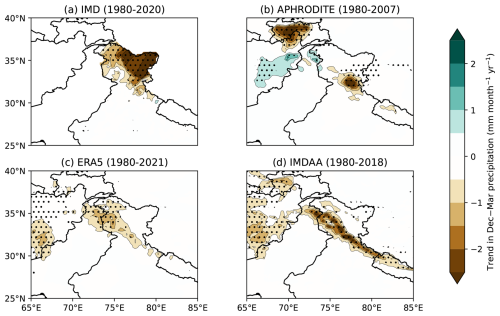
Figure 22Trends in winter (DJFM) precipitation (millimetres per month per year) over Pakistan and northern India since 1980, as recorded in four datasets, two gridded gauge-based products (IMD and APHRODITE), and two reanalyses (ERA5 and IMDAA). Stippling indicates where trends are significantly different from zero at the 95 % confidence interval.
7.2.2 Changing impacts
With variability in WD frequency and intensity comes variability in their local impacts, many of which – such as precipitation and cold waves – are of considerable importance to the communities based in and around the western Himalaya. We can also infer recent trends in WD frequency and intensity through changes in their regional impacts, such as precipitation (Gurung et al., 2017) and cold waves (Das and Meher, 2019). Although WD frequency is highly correlated with winter precipitation (Dimri et al., 2023), such inferences over climate timescales must be considered with additional caution, since they are also sensitive to changes in the synoptic environment (e.g. atmospheric moisture content). This highlights one of the most important questions – which has the greater impact on precipitation trends, trends in thermodynamics or atmospheric circulation?
The dominant focus on changing WD impacts has been on precipitation, typically using in situ gauge measurements. However, this area is very poorly resolved by the gauge network, and there are large biases in measuring snowfall using standard rain gauge equipment (Adam and Lettenmaier, 2003; Strangeways, 2006). This means a proper intercomparison must be conducted carefully, since the heterogeneous behaviour of precipitation means that trends may differ between datasets (Chevuturi et al., 2018) and even between neighbouring basins (Fig. 22). Once datasets or regions with spurious behaviour are removed from the analysis, the key issue is decadal variability – meaning the results are sensitive to the choice of analysis period. This was highlighted by Baudouin et al. (2020a), who found a regional minimum in winter precipitation between 1995 and 2010. This interdecadal variability has also been found in regional river discharge (Gardelle et al., 2012; Mukhopadhyay and Khan, 2014). These caveats are highlighted by contrasting results in recent studies. In Fig. 22, trends in seasonal winter precipitation from four widely used datasets are shown for the last 40 years. The IMD has almost no stations in the only area where the trend is significantly negative, but the other three sources largely agree that there has been significant drying in the Himalayan foothills and over Afghanistan.
Most studies report either a negative or an insignificant trend in winter precipitation across the western Himalaya and surrounding region. In a comprehensive assessment of precipitation trends in different datasets over Leh (Ladakh), stratified by season, Chevuturi et al. (2018) found that significant interdecadal variability was present across all long-term datasets, making assessment of underlying trends challenging, although the trend in gauge-measured precipitation there was significantly negative. Waqas and Athar (2019) reached a similar conclusion, finding that a weak negative trend in winter rainy days recorded at stations across northern Pakistan and the Karakoram and a weak positive trend at stations across the western Himalaya were statistically insignificant in the context of large decadal-scale variability. Other studies have reported similar results – i.e. a weak trend dominated by interdecadal variability – for the central Himalaya and Nepal Shrestha et al. (2019), states of north India (Rajasthan, Gujarat, Punjab Narayanan et al., 2016), Jammu (Khan et al., 2023), and Kashmir (Dar, 2023).
The role of decadal-scale variability is most clearly highlighted by opposing results of long- and short-term studies. Using gridded gauge data from 1901 until present, both Nageswararao et al. (2016) and Chauhan et al. (2022) found generally positive trends in winter precipitation over north India. However, as in Fig. 22, those studies that have measured their trends over comparatively short periods (∼40 years) (Shekhar et al., 2010; Zaz et al., 2019; Ullah et al., 2022; Abbas et al., 2023; Safdar et al., 2023) instead report a significant decline in winter precipitation across north India and Pakistan. These studies are therefore likely to be detecting a mode of interdecadal variability. Long-term studies of aridity during the rabi season (i.e. the winter months) have also indicated a trend towards wetter conditions over northern Pakistan in the regions typically affected by WDs (Ahmed et al., 2018, 2019), although this too appears to be subject to significant interdecadal variability (Ullah et al., 2022).
Those studies reporting declining trends in winter precipitation typically invoke declining WD frequency and shifts in subtropical jet position as the cause, as did Gunturu and Kumar (2021), who argued that a recent decline in WDs has been responsible for reduced cloud cover and increased fog over the recent decades.
Studies agree that climate change has led to earlier snowmelt across the western Himalaya and Hindu Kush and that this has a negative impact on rabi crop production (Biemans et al., 2019) and ecosystems (Isaak and Luce, 2023). In a global assessment of extreme wet conditions, Sreeparvathy and Srinivas (2022) found an increasing trend in the variability of short-duration heavy rainfall events over northwest India, which they partially attributed to WDs. Similarly, using gauge, satellite, and reanalysis data, Nischal et al. (2023) reported a significant increase in extreme winter precipitation event frequency across large regions of the western Himalaya and Karakoram since 1980, which they attributed to increased WD frequency through observed increases in upper-level baroclinicity and vertical wind shear and a decrease in static stability over the region. Nash et al. (2024) attributed a recent increase in extreme precipitation in the western Himalaya to greater moisture availability and an increased freezing level, meaning that atmospheric rivers associated with WDs produce more rainfall than in the past. Bhat et al. (2024) reported a significant and very large increase in reported pre-monsoon hailstorms in Kashmir between 2007 and 2022. This is likely due to WDs, as the predominant source of non-monsoonal convective activity in the region.
Some studies have investigated changes in cold wave frequency and their relationship to changes in WD characteristics. Both Gupta et al. (2018) – for the Indo-Gangetic Plain – and Dash and Mamgain (2011) – for Jammu and Kashmir – found a significant decrease in cold waves since the 1980s, albeit again with substantial decadal variability. Both studies attributed their respective trends to decreasing WD frequency or intensity, but such interpretations must be treated with caution given the positive trend in background climatological temperatures. Athira et al. (2024) also identified a significant decrease in cold wave frequency, duration, and intensity over north India.
Interestingly, two studies have discussed the changing impact of WDs on summer precipitation across north India. Prathipati et al. (2019) and Kumar et al. (2020) showed an increase in summer precipitation since 1991 and since 1953 respectively. Because summer monsoon precipitation is reduced elsewhere, both studies suggest that the trend is explained by an increase in WD activity in summer (as hypothesised by Valdiya, 2020).
While the general decline in snowfall is attributed to a warming climate, the spatial variability is thought to be linked to elevation-dependent warming (Dimri et al., 2022), where trends in near-surface warming increase as a function of surface elevation. There is a large body of evidence in support of elevation-dependent warming across the Tibetan Plateau, Himalaya, Karakoram, and Hindu Kush, though understanding is limited by the sparse observational network (Pepin et al., 2015; You et al., 2020; Doblas-Reyes et al., 2021), all the more so for precipitation (Palazzi et al., 2015a). There are thought to be a number of important drivers, depending on season and location, with changes in albedo (Ghatak et al., 2014; Byrne et al., 2024), snow depth, cloud cover (Duan and Wu, 2006), near-surface humidity (Rangwala et al., 2009), lapse rate (Qin et al., 2024), and radiative forcing (Palazzi et al., 2017) chief among them. High-resolution modelling has recently ruled out cloud cover and humidity, arguing that albedo and circulation are the primary drivers (Byrne et al., 2024). These point not only to local feedbacks, but also the importance of synoptic-scale meteorological drivers (Guo et al., 2021), such as WDs, highlighted by the fact that the elevation-dependent warming signal is strongest in the winter months (Dimri et al., 2022; Hu and Hsu, 2023). Regardless of the sign of change of WD frequency and intensity, the strength of the elevation-dependent warming signal is likely to continue to drive reduced winter snowfall over the western Himalaya in at least the short term. Still, elevation-dependent warming appears to saturate, or even reverse, at altitudes above about 5 km (Gao et al., 2018; Guo et al., 2019; Salerno et al., 2023) and appears to have significant interdecadal and spatial variability in long-term in situ observations (Li et al., 2020; Doblas-Reyes et al., 2021). Even so, it is well simulated in CMIP5 (Palazzi et al., 2017) and CMIP6 (Zhu and Fan, 2022) models, as well as at a wide range of resolutions (Palazzi et al., 2019).
Such results on elevation-dependent warming beg the question as to whether WD activity is playing a role in the Karakoram Anomaly, in which glaciers in that region are either growing or stably increasing, counter to the expected impacts of global warming (Hewitt, 2005) and against the trend of glaciers in neighbouring ranges such as the western Himalaya. Although a handful of articles dispute whether it is a real phenomenon (e.g. Negi et al., 2021), it is generally widely accepted (Gardelle et al., 2013; Miles et al., 2021; Li et al., 2023). The relative growth and shrinking of glaciers across the Himalayan and Tibetan regions over the last 2 decades is summarised in Fig. 23, from Farinotti et al. (2020). Glacial mass balance in the Himalaya and Karakoram is very sensitive to seasonal snowfall variability (Kumar et al., 2019c) and hence probably also to changes in WD frequency; indeed, WDs contribute approximately 50 % of the annual precipitation over Karakoram (Fowler and Archer, 2006; Barros et al., 2006).
The Karakoram Anomaly is typically explained through increased cloud cover and snowfall and their associated radiative feedbacks (Bashir et al., 2017; Dimri, 2021; Li et al., 2023) over the region. These are largely explained by changes in local and large-scale circulation, with studies noticing the atmosphere has become more cyclonic over the Karakoram (sometimes referred to as the Karakoram vortex; Forsythe et al., 2017; Norris et al., 2019). This increased cyclonicity is almost certainly related to changes in WD behaviour; however, as we have discussed earlier in this section, studies do not really agree on the sign of change of WD frequency over the Karakoram, although it is probably increasing (Hunt, 2024). Instead, Javed et al. (2022) argued that while there was no significant trend in WD frequency over the Karakoram, WDs have become significantly more intense over the last few decades and thus have led to a significant increase in winter precipitation over the Karakoram. This mechanism has also led to increased high-altitude snow cover across the Upper Indus Basin (Bilal et al., 2019).
de Kok et al. (2018) argued that glaciers in the Karakoram have likely been growing due to increased regional irrigation during the pre-monsoon and summer monsoon, which has accelerated significantly in the last few decades. They hypothesised that the positive anomaly will disappear in the unlikely scenario that this irrigation stabilises or reduces but that this reduction could be amplified, offset, or even reversed by any trends in WD-related snowfall over the Karakoram. Disentangling these two effects may be challenging, as irrigation also provides a local source of moisture for precipitating WDs. Potential sensitivity to WDs has been highlighted in the western Himalaya too: using measurements of glaciers there starting in 1901, Mehta et al. (2021) showed that trends in glacial ablation are most closely associated with increasing temperature, but trends in glacial accumulation are more closely associated with increased winter precipitation, particularly due to WD activity, which they state is the primary source of glacier recharge in this region. Over a short study period (2016–2019), they also found a strong sensitivity to altitude, showing that glaciers below ∼5200 m lost mass but gained mass above that altitude, perhaps due to increased precipitation in a warming environment. Overall, however, glaciers in the western Himalaya lost mass at a rate of 0.36 m.w.e. (metres of water equivalent) per year between 2016 and 2019. The relationship between WD-related precipitation and surface elevation was also described over Pakistan by Kattel et al. (2019), who showed higher elevations experienced significantly more winter precipitation and cloud cover than lower elevations.
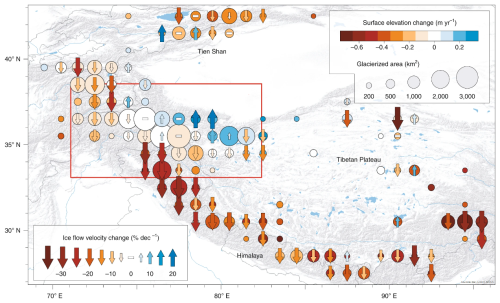
Figure 23From Farinotti et al. (2020). The rate of glacier surface-elevation change (Brun et al., 2017) is shown together with changes in ice flow velocity (Dehecq et al., 2019) for the period 2000–2016. The size of the circles is proportional to glacier area. Data are aggregated on a 1° × 1° grid. The red box marks the Karakoram and surrounding area.
Despite these advances, further research is urgently needed to understand how climate change in the Himalaya, Karakoram, and Hindu Kush regions will affect downstream wetlands, agriculture, and ecosystems more broadly (Chettri et al., 2023).
In summary, recent work shows that trends in winter precipitation over the western Himalaya are a complex function of period, location, and dataset, though most measurements indicate a strong decadal-scale mode of variability. Inference of WD behaviour from these trends is thus also very challenging, additionally being sensitive to changes in seasonality and subtropical jet location, with different methods yielding a wide range of answers. In their review of the topic, Krishnan et al. (2019b) stated that the implications of warming on the hydrological cycle of the Hindu Kush are “not yet clear”. Following Forsythe et al. (2017), they did note that local increases in WD activity were linked to increases in glacial mass in the Karakoram and western Himalaya, but they carefully stated the uncertainty in their summary for policymakers: “The Hindu Kush Himalaya is experiencing increasing variability in western disturbances and a higher probability of snowfall in the Karakoram and western Himalaya, changes that will likely contribute to increases in glacier mass in those areas. This finding runs counter to many expectations in the scientific community, and more research is needed to understand the reasons for this and its potential future implications.” The IPCC (Intergovernmental Panel on Climate Change) AR6 working group 1 report (Doblas-Reyes et al., 2021) summarised the region thus: “Over most of the Hindu Kush Himalayan region, snow cover has reduced since the early 21st century, and glaciers have retreated and lost mass since the 1970s. The Karakoram glaciers have remained either in a balanced state or slightly gained mass. During the 21st century, snow-covered areas and snow volumes will decrease in most of the Hindu Kush Himalayan, and snow line elevations will rise and glacier volumes will decline (high confidence). A general wetting across the whole Tibetan Plateau and the Himalaya is projected, with increases in heavy precipitation in the 21st century.”
There is, therefore, high confidence (robust evidence, medium agreement) that winter precipitation has declined over the western Himalaya, Hindu Kush, and Karakoram during the instrumental period.
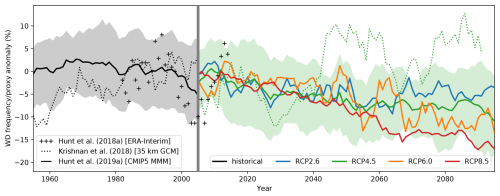
Figure 24Projected future trends of WD activity under historical (black), RCP2.6 (blue), RCP4.5 (green), RCP6.0 (yellow), and RCP8.5 (red) emissions scenarios. Three studies are incorporated: Hunt et al. (2019b), who tracked WDs in 22 CMIP5 models, the multi-model means for which are denoted by solid coloured lines (black for historical experiment); Hunt et al. (2018b), who used the same tracking algorithm on the ERA-Interim reanalysis, denoted with crosses; and Krishnan et al. (2019a), who used band-passed geopotential variance as a proxy for WD activity applied to a single 35 km GCM, denoted by dotted lines. All values are computed as 5-year running means and are given as anomalies to their respective 1980–2000 means. Colour-shaded regions indicate the inter-model interquartile ranges for the historical and RCP4.5 track frequencies in Hunt et al. (2019b).
7.3 Future projections
In the final section of the main body of this review, we turn our attention to the projected behaviour of WDs in future climate scenarios. As we saw in the previous section, quantifying trends in historic WD frequency is a challenging endeavour, and one which is compounded as we look towards the future. Here, weather reports and reanalyses are replaced with climate models that come with their own, much larger, uncertainties. Therefore, because direct counting from bulletins and reports cannot be used, and because one must either derive and apply a proxy to, or use a tracking algorithm on, climate model output, very few studies have attempted to estimate WD activity in the coming century.
Studies of global extratropical cyclone behaviour in CMIP5 future climate scenarios have suggested a poleward movement of storm tracks (Catto et al., 2011; Chang et al., 2012). Such a trend would result in a decline in WD frequency as an increasing fraction would be deflected north of the Tibetan Plateau. However, Ridley et al. (2013) disagreed with this; in a study using cluster analysis to quantify changes in WD frequency, they found WD-like regimes increased significantly in the RCP4.5 future scenario.
Earlier studies have also reported large uncertainties in projected changes to winter precipitation over the western Himalaya, due in part to the typically coarse resolution of CMIP5 models not resolving crucial convective and orographic processes (see Sect. 5). These uncertainties involve disagreement between individual models (Palazzi et al., 2015b) as well as high spatial inhomogeneity (Su et al., 2016). A focused study by Panday et al. (2015) found a small but significant increase in winter precipitation over the western Himalaya in the RCP8.5 future scenario and projected that extremes would become less frequent but more intense. Either way, it is likely that snowpack depth will continue to decline due to the strong signal in increasing temperature (Terzago et al., 2014). There was thus no consensus on whether climate change would cause WD frequency to increase or decrease and only low confidence that winter precipitation would increase.
7.3.1 Counting WDs
Prior to the review of Dimri et al. (2015), this was explored only in Ridley et al. (2013). They applied a clustering technique to output from a 25 km regional climate model forced at the boundaries by output from coarser RCP4.5 experiments conducted with the ECHAM5 and HadCM3 models. They identified four weather regimes associated with anomalous snowfall over the Karakoram and Himalaya, linking one – associated with low surface pressure and anomalous southerlies – to western disturbances. In both experiments, this cluster increased significantly in frequency over the coming century and was associated with an increase in both seasonal snowfall and intensity of snowfall events.
Krishnan et al. (2019a) employed a different proxy technique, following the earlier work of Madhura et al. (2015) in using band-passed variance of upper-level geopotential height. They found (Fig. 24) that this metric of activity increased in a single-model RCP4.5-forced regional projection, though with a similarly strong decadal variability to that found during the previous century. They attributed this to an enhanced upper-level quasi-stationary trough over central Eurasia which occurs as a result of differential heating over the Tibetan Plateau. Also deploying a proxy method, Midhuna et al. (2023) developed a regression model for predicting precipitation over the western Himalaya. Using a single CORDEX-SA model, they used days with precipitation exceeding 3.5 mm as a proxy for WD frequency and seasonal precipitation as a proxy for WD intensity. They found both had insignificant declining trends across the 21st century.
To date, only one study has applied a tracking algorithm to climate model output in an attempt to quantify the future activity of WDs. Hunt et al. (2019b) applied the feature-tracking algorithm developed in Hunt et al. (2018b) to the output of 24 CMIP5 models. Compared to a 1980–2005 baseline, they found that the 2080–2100 WD multi-model mean frequency decreased by 11 % and 17 % in RCP4.5 and RCP8.5 experiments respectively (Fig. 24), both statistically significant and in contrast to the trend found in recent WDs in Hunt (2024). The decline in frequency was accompanied by significant declines in the WD multi-model mean intensity (12 % in RCP8.5) and seasonal precipitation (15 % in RCP8.5 over north India). They attributed these changes in frequency and intensity to a widening and weakening of the subtropical jet, which is subsequently associated with a decrease in meridional wind shear and baroclinic vorticity tendency, resulting in less efficient WD development. Aside from the obvious implications for water security, reduced WD frequency would likely result in worsening pollution over north India and Pakistan (Paulot et al., 2022; Xie et al., 2024).
Therefore, there is very low confidence (limited evidence, low agreement) that future WD frequency will decline due to climate change.
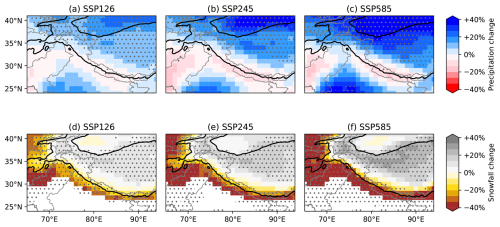
Figure 25Projected change in winter (DJFM) precipitation (a–c) and snowfall (d–f) from the current climate (1960–2000) to the end of the century (2060–2100) as a function of emissions scenario. Computed using 37 CMIP6 models, each regridded to 1° × 1°. Stippling indicates where at least two-thirds of the models agree on the sign of change. The 2500 m surface-elevation contour is denoted with a thick black line.
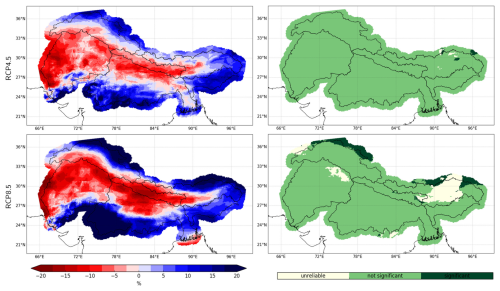
Figure 26From Jury et al. (2020). Median climate change of precipitation for December–April (left column) under the RCP4.5 (top) and the RCP8.5 (bottom) scenarios at the end of the 21st century and the respective levels of agreement between the models (right column; unreliable: ≤50 % of models show significant changes but agree with <80 % on the sign of change; not significant: <50 % of models show significant changes (but ≥80 % agree on the sign); significant: ≥50 % of models show significant changes and agree with ≥80 % on the sign of change; significance has been derived using the Wilcoxon–Mann–Whitney test). There are no areas where <80 % of models agree on the sign but ≥50 % of models show significant changes. This is a multi-model ensemble comprising output from CORDEX-SA and dynamically downscaled regional models derived from CMIP5-class GCMs.
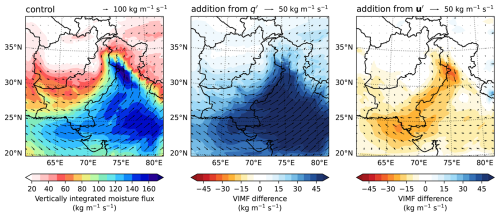
Figure 27Adapted from Fig. 11 of Hunt et al. (2020), where 40 historical WDs were simulated in WRF at a resolution of 20 km. The left panel shows their composite vertically integrated moisture flux. The next two panels show the change in composite vertically integrated moisture flux from changes to tropospheric moisture content (centre) and dynamics (right) when the same WDs are simulated in an RCP8.5 PGW framework – i.e. by perturbing the boundary conditions to reflect projected changes in atmospheric and surface variables.
7.3.2 Changing impacts
Understanding the role of WDs in both mean and extreme precipitation over the western Himalaya and surrounding region in the face of climate change is clearly of paramount importance, given the sensitivity of the local and downstream population to changes in the many aspects of water security (e.g. Mishra et al., 2020). CMIP5- and CMIP6-class models alone are not suitable tools for this task: their horizontal resolutions – usually of order 100–200 km – are incapable of resolving either the complex orography or even the most basic of convective processes (Sect. 5.2.2). Well-simulated dynamics, a good land surface model, and a good parameterisation scheme for convection mean that the projected changes to seasonal mean precipitation may be reasonably accurate, but this does not extend to extreme precipitation – often brought about by sub-grid-scale convective storms – for which more advanced tools are needed.
Studies based on CMIP5 and CMIP6 model output have agreed that higher elevations are very likely to receive significantly more precipitation in a warmer climate, with insignificant drying in the foothills and increased rainfall over the northern Indian and Pakistani plains (Almazroui et al., 2020; Banerjee and Singh, 2022; Singh et al., 2024). Banerjee and Singh (2022) also found a shift in precipitation seasonality, with the peak snowfall month moving from February to March by the end of the 21st century. They attributed this to changes in WD frequency. Looking at the Hindu Kush, Rahman et al. (2022) reported significantly increasing trends for projected winter precipitation, strongest in the lower and mid-elevations, in contrast to the recent drying they found in station-based observations for the region. Deng and Ji (2023) also found significant winter wetting of the western Tibetan Plateau in dynamically downscaled CMIP6 experiments. This general wetting trend appears to be at odds with the projected decline in WD frequency and intensity (Hunt et al., 2019b), but these studies agree that the region that receives the heaviest WD precipitation – the foothills – is projected to undergo insignificant drying. The changes described in these studies are summarised in Fig. 25, where we use a slightly longer baseline of 40 years to smooth out decadal variability.
The Coordinated Regional Climate Downscaling Experiment (CORDEX) offers an opportunity to move beyond these coarse resolutions, with the regional experiments over South Asia (CORDEX-SA) reaching horizontal resolutions of between 25 and 50 km. While this is still inadequate to resolve convection, the considerable improvement in orographic representation at these resolutions has made CORDEX-SA the tool of choice for recent studies investigating the fate of winter precipitation over the Himalaya.
Jury et al. (2020) constructed an ensemble from 36 CMIP5 models and 13 CORDEX-SA regional climate models, based on their ability to reproduce circulation patterns – including WDs – and elevation-dependent warming signals (e.g. Palazzi et al., 2017, 2019). They found considerable spatial heterogeneity in projected changes to extended winter (December–April) precipitation but noted a tendency to decrease along the foothills but increase at both higher and lower elevations (Fig. 26), a pattern similar to that recovered from simple CMIP6 analysis (Fig. 25), and a value consistent with the expected decline in WD frequency of 11 %–17 % reported by Hunt et al. (2019b). As the heaviest precipitation falls along the foothills, it is this projected decrease that potentially has the greatest impact. However, these trends in total precipitation were not significant at any elevation, although the authors reported a strong and significant decline in snowfall across the region by the end of the century. Such results reflect the fact that regardless of the overall trend, snowfall will tend to decline at the expense of increasing rainfall across the foothills and Himalaya but will tend to increase over the Karakoram and Tibetan Plateau (Fig. 25; Rajbhandari et al., 2015; Hunt et al., 2020). Glaciated regions with a projected increase in snowfall in Fig. 25, namely much of the Karakoram, are the same regions experiencing anomalous glacier growth in the present climate (Fig. 23).
Other studies using CORDEX-SA or statistical downscaling have come to very similar conclusions (Midhuna and Dimri, 2020; Ballav et al., 2021; Midhuna and Dimri, 2022; Dimri et al., 2022; Meher and Das, 2022), typically attributing the overall increase in precipitation in the region to a strengthening of the subtropical jet and increased southerly moisture flux over the Arabian Sea. These studies only really differ in which regions they find statistically significant trends. Among these, Midhuna and Dimri (2022) and Meher and Das (2022) also reported changing seasonality, finding a relatively larger increase in the mean and standard deviation of precipitation respectively during the late winter (February) and spring (March and April), and thus providing further evidence for a lengthening of the active WD season due to climate change. These results show that trends in mean precipitation are not particularly sensitive to model resolution and thus appear to be adequately captured in coarser CMIP5 and CMIP6 models. However, given the importance of convective-scale processes in this region, convection-permitting climate models may yet overturn this conclusion.
Although the relationship between climate change and WD frequency remains unclear, Hunt et al. (2020) used the recently developed technique of pseudo-global warming (PGW; Kimura and Kitoh, 2007; Prein et al., 2017) to quantify the relationship between climate change and WD intensity, as well as the subsequent impact on WD-related precipitation. The authors isolated 40 case studies of WDs from earlier literature, simulating each in a high-resolution (4.4 km) setup of WRF to ensure that both convection and orography were well represented. In addition to this control experiment, they also ran additional RCP4.5 and RCP8.5 experiments. In each case, the boundary conditions of the experiment were perturbed using CMIP5 multi-model means to reflect the different large-scale environments that such WDs would encounter if they developed within those respective climates. They found significant increases in net WD-related precipitation in the future climate scenarios, despite a weakening of WD intensity. For example, in their RCP8.5 composite (Fig. 27), they found that WD-induced moisture flux incident on the western Himalaya was reduced 20 % through weakened WD circulation but increased 28 % through increased moistening of the tropical atmosphere. They also found significant decreases in the return periods (i.e. significant increases in the frequencies) of extreme WD-related precipitation events in both RCP4.5 and RCP8.5 future scenarios.
These studies all lean towards a wet–dry–wet trend with increasing elevation in the western Himalaya, as shown in Fig. 25. The wetting trend to the south is mostly over the southern Indo-Gangetic Plain and northern Deccan Plateau, where WDs provide little precipitation. Over the foothills, where the mean winter precipitation is greatest, there is a projected drying trend. This drying is perhaps related to the projected decline in WD frequency and intensity reported in Hunt et al. (2019b), but most studies find that it is statistically insignificant. At higher elevations, across much of the western and central Himalaya, there is a projected increase in precipitation, likely driven by a combination of elevation-dependent warming and the Clausius–Clapeyron relationship8. There is also a nonlinear component (Baudouin et al., 2021) whereby WDs are intensified through enhanced moist baroclinic instability associated with the orography; i.e. changes in specific humidity lead to local circulation changes. This pattern underscores the complex nature of precipitation trends over the winter Himalaya, where it is likely that large-scale circulation is weakening the transport of moisture (Fig. 27c), and this is counterbalanced by increasing specific humidity. The uncertainty that remains lies in determining which of the two processes will win and where.
There is high confidence (robust evidence, medium agreement) that climate change will cause winter precipitation to increase over the western Himalaya but decrease in the foothills. There is very high confidence (robust evidence, high agreement) that climate change will cause the ratio of snowfall to rainfall across the region in winter to decrease.
8.1 Summary
Western disturbances (WDs) are baroclinic upper-tropospheric lows that travel eastward along the subtropical westerly jet in the Northern Hemisphere. WDs have a positive vorticity anomaly that extends through much of the troposphere, with a maximum at about 350 hPa, and are often associated with surface lows. They are most common during the boreal winter months (December to March), during which they bring heavy precipitation to the mountain ranges of Pakistan, north India, northern Afghanistan, and Tajikistan: the western Himalaya, the Karakoram, the Hindu Kush, and the Pamirs. As a result, they are a crucial component of the water security of both the Indus and Ganges River basins. This paper reviews the large volume of recent research on WDs. That research, summarised in Table 2, has leveraged a wealth of novel computational, modelling, and experimental techniques that have significantly improved our understanding of WD behaviour and impacts, making this updated review necessary.
The recent development of tracking algorithms for WDs, and their subsequent application to reanalyses and climate model output, has allowed the production of WD track catalogues. These catalogues have been a key step in building robust WD statistics, such as their climatology and variability – with the total number of WDs per year varying between about 45 and 65 and the number of strong (also referred to as active) WDs per year varying between 15 and 25. Composite studies based on these catalogues have revealed much about the three-dimensional structure of WD: a vorticity maximum at 350 hPa, with a cold anomaly below, deep ascent to the east, and descent to the west.
Similarly, robust links to moisture supply, large-scale forcings, and natural hazards have been established through the use of WD catalogues. Moisture trajectory and isotopic analyses have revealed the dominant role of the Arabian Sea as a moisture source for typical WDs, although extreme WD rainfall is typically produced through interaction with elements of the summer monsoon. Crucially, these mean impacts associated with WDs will be highly sensitive to any future changes in mean WD track latitude, as they move closer to or further from the Arabian Sea.
Forcing of WD activity by teleconnections remains relatively unexplored, with the exceptions of the NAO and ENSO. Studies have demonstrated the NAO can vary monthly WD frequency by about 20 % through upstream modulation of the subtropical jet. Studies have not been able to agree on the role of ENSO, although it has been proposed that different flavours of ENSO, such as El Niño Modoki, may be important. The reason for the ENSO teleconnection and the variability of its strength need further investigation. A better understanding of these, and other, teleconnections will lead to improved seasonal forecasts of WD frequency and winter precipitation.
Climatological studies have now linked WDs to increased risk in winter of landslides in the Upper Indus Basin (∼60 % associated with WDs), fog over northern India (over 50 %), and extreme precipitation events over India and Pakistan (over 90 %). They have not yet been statistically linked to an increased risk of cold waves or avalanches, although a small body of case studies exists to support these claims.
High-resolution models (<4 km) can now explicitly resolve certain convective and local orographic processes. Recent studies have shown that accurate representation of these processes and their interaction with each other is vital for the accurate simulation of heavy precipitation events associated with WDs. Operational forecasts are not typically run at these fine scales, thus accurate predictions of hazardous WD precipitation still present a challenge. This lack of reliable forecasts of WD tracks and impacts means it is difficult for those affected to prepare adequately in advance.
Measured trends in WD frequency over the last 50 years depend strongly on the choice of study region and season. Studies have used a range of valid techniques to estimate WD frequency, such as counting them in weather bulletins, tracking them in reanalyses, and using proxy variables such as band-passed geopotential variance. Trends in WD frequency tend to be positive if measured over the Hindu Kush, Karakoram, and north Pakistan, and they tend to be negative elsewhere, although there is disagreement between studies. Crucially, there is an emerging consensus that WD frequency has increased significantly in the spring (March to June). This shift in seasonality will have a significant impact on the livelihood of those living in north India and Pakistan.
There is, as yet, insufficient evidence to determine whether future climate change will increase or reduce WD frequency as the two studies investigating this disagree with each other. However, the study projecting a decline noted that the decline was consistent and significant across a range of CMIP5 models, with the multi-model mean projecting declines in WD frequency and intensity of 15 % and 12 % respectively by the end of the 21st century.
Future projections of winter precipitation only weakly agree on a decline over the foothills of the western Himalaya but agree on a significant increase in precipitation at higher elevations. The proposed causes of these changes include, but are not limited to, poleward movement of the subtropical jet, bringing WDs to higher latitudes, and an increase in moisture flux associated with WDs brought about by the greater moisture-carrying capacity of a warmer troposphere. Either way, it is likely that extreme precipitation events associated with WDs will become more severe, with serious implications for the populations of the Indus and Ganges basins.
So, despite significant progress having been made in the research and understanding of WDs over the last decade, many important challenges remain. Of these, two are particularly important. Firstly, accurate forecasts of their tracks and associated precipitation are hindered by the complex interactions with orography and convective processes. Secondly, there is still no consensus on the historical or future response of WD activity to climate change, partially due in the former to inconsistent definitions of WDs and partially due in the latter to insufficient model resolution. The likely pathway to resolving both of these challenges is high-resolution convection-permitting models, both in operational forecasting and climate science.
8.2 Future research questions and challenges
Despite the large volume of research on WDs, a number of important questions remain about their behaviour and impacts – either because they have not yet been investigated or because studies have arrived at divergent results. We conclude this review by summarising these important unknowns, based on the discussions throughout this paper. For each entry, the relevant section or subsection is given in square brackets.
-
What is the sensitivity of WD tracks and their statistics to the choice of reanalysis? This could be explored between reanalyses or by using multi-member reanalyses (e.g. the 10-member ERA5 ensemble). [“Detection and tracking” (Sect. 2.2)]
-
Do WDs occur in clusters, arriving in rapid serial succession with longer gaps between groups? If so, why? This behaviour has been found in extratropical cyclones, e.g. over the North Atlantic (Priestley et al., 2017; Dacre and Pinto, 2020). Such work would need to overcome the limitations of tracking algorithms, where WDs in rapid succession may not be easily separable, degrading the quality of detection. [“Detection and tracking” (Sect. 2.2)]
-
Can WDs merge or split? It is certainly possible given the behaviour of similar PV streamers and cutoffs (Kew et al., 2010; Portmann et al., 2021) as well as the discussion in Cannon et al. (2016). How would such events affect associated precipitation? As in the previous question, this work would need to overcome the limitations of current tracking algorithms. [“Detection and tracking” (Sect. 2.2)]
-
What is the relationship between the different regions of WD cyclogenesis and their downstream behaviour and impacts? Do those forming away from the subtropical jet have inherently different characteristics? Are there different WD cyclogenesis mechanisms beyond simply dynamical (baroclinic) instability? [“Cyclogenesis” (Sect. 2.3.1)]
-
Do conditional instability of the second kind (CISK; heating rate proportional to relative vorticity) or wave-CISK (heating rate proportional to vertical velocity) instabilities play a role in the PV–convection relationship as WDs intensify near the Himalayan orography? This could be complementary to the baroclinic instability mechanism proposed by Baudouin et al. (2021). [“Intensification and moist thermodynamics” (Sect. 2.3.2)]
-
Why do some WDs contain frontal regions while others do not? This is one of the major characteristics separating WDs from extratropical cyclones, which almost invariably contain frontal structures (Browning, 1999; Catto, 2016; Schemm et al., 2018). Such analysis would benefit from front-centred composites, e.g. using gradients in equivalent potential temperature (e.g. Dacre et al., 2012), which has not yet been carried out for WDs. [“Vertical structure” (Sect. 2.3.4)]
-
Related to the previous question, some case studies have identified that bands of very high vorticity can develop near the surface during the passage of WDs, stretching from the Sulaiman Range in central Pakistan to the Himalaya. What causes these bands, and what are their impacts? [“Vertical structure” (Sect. 2.3.4)]
-
A rigorous quantification of the moisture sources of WD precipitation has not yet been performed. Even though it is known that the majority comes from the neighbouring Arabian Sea, what fractions are carried over longer distances from the Mediterranean, Black, or Caspian seas? Do different source ratios affect the resulting precipitation? How sensitive are these ratios to WD characteristics such as latitude? Such research could build on the comprehensive case studies by Boschi and Lucarini (2019). [“Moisture sources” (Sect. 2.4)]
-
WDs vary considerably in their intensity and impacts. What is the best way of classifying or categorising them? Should we base a classification on WD impacts (e.g. precipitation), characteristics (e.g. latitude), or dynamics (e.g. upper-level vorticity or wind speed)? [“Variability” (Sect. 2.6)]
-
Are there differences between WDs that arise as PV cutoff lows and those that arise as dynamical instabilities in the subtropical jet? [“Variability” (Sect. 2.6)]
-
What are the primary causes of intraseasonal variability in WD frequency and other characteristics? [“Variability” (Sect. 2.6)]
-
Neal et al. (2020) showed that weather regimes associated with WDs also lead to increased winter precipitation over northeast India. It is not known whether this is caused by WDs propagating into the region, instability caused by the position of the jet, or some other weather phenomenon. [“Precipitation and natural hazards” (Sect. 3)]
-
How can better knowledge of WDs translate to decisions around water management? This is a multidisciplinary topic which also requires better observations, operational prediction, forecast skill assessment, and decision-support tools. [“Water security” (Sect. 3.3) and “Instrumental and reanalysis records” (Sect. 7.2)]
-
What are the socioeconomic losses associated with historic WD activity? How are these best quantified? What kind of WD characteristics are associated with them? [“Other natural hazards” (Sect. 3.4) and “Water security” (Sect. 3.3)]
-
Which WD characteristics, such as location, intensity, and timing, is associated natural hazard occurrence most sensitive to? It is likely that this will be different for different hazards. [“Other natural hazards” (Sect. 3.4)]
-
Cold waves have thus far only been anecdotally linked to WDs. Is there a climatological link between the two? If so, which is more important: northerly advection of cold air in the rear flank or sustained heavy cloud cover? [“Cold waves” (Sect. 3.4.3)]
-
What role do WDs play in driving nor'westers (storms that impact northeast India and Bangladesh), if any? Understanding this link could be crucial to reducing lightning-related mortalities in this region. [“Lightning and hailstorms” (Sect. 3.4.4)]
-
Do WDs occurring during the summer monsoon have different characteristics than typical winter WDs? Monsoonal WDs have access to an abundant moisture supply that is not present in the winter months, but does this significantly affect their structure or evolution? There is also evidence that WDs occurring during the summer arise from high-latitude PV cutoff lows, rather than arising as instabilities within the subtropical jet. [“Interaction with the Indian summer monsoon” (Sect. 3.5)]
-
Is the seasonality of WDs changing, as Valdiya (2020) suggests? [“Interaction with the Indian summer monsoon” (Sect. 3.5) and “Instrumental and reanalysis records” (Sect. 7.2)]
-
More work is needed to establish the link (if any) between WD characteristics and slowly varying teleconnections. Many of these have been investigated in detail in previous studies, but the connections with the MJO, SAM, PDO, and IOD are still not well understood. These would in turn improve seasonal forecasts. [“Large-scale forcing and teleconnections” (Sect. 4)]
-
The Pamir bifurcation occurs when the subtropical jet passes near the Pamir Mountains. These mountains act like a wedge, deflecting WDs south into India or north into central Asia with only small variation in jet latitude. How can the impact of the Pamir bifurcation be reduced in forecasts? Is there a critical subtropical jet latitude at which forecast uncertainty increases significantly? [“Forecasting” (Sect. 6)]
-
It would be beneficial to conduct quantitative analysis on the quality of IMD warning bulletins so that stakeholders know at what lead times and in which locations they are most reliable. [“Short range” (Sect. 6.2)]
-
More quantitative research is also needed on extended-range and seasonal WD forecasting. This includes, but is not limited to, quantification of track, frequency, and intensity errors. Also, can skill be improved through hybrid statistical techniques that leverage connections to large-scale forcing such as the NAO and ENSO? [“Extended range and subseasonal” (Sect. 6.4)]
-
Aside from better understanding of teleconnections, how might subseasonal and seasonal prediction of WD activity be improved? [“Extended range and subseasonal” (Sect. 6.4)]
-
Studies of western Himalayan paleoclimate over the last 10 millennia often disagree considerably on periods of surplus or deficient winter precipitation (and hence WD activity). To what extent is this due to contamination from the summer monsoon signal, and to what extent can it be explained by the large spatial variability in climatic trends of Himalayan precipitation? [“Paleoclimate proxy measurements and simulations” (Sect. 7.1)]
-
What is the relationship between observed elevation-dependent warming and changes in WD behaviour? [“Instrumental and reanalysis records” (Sect. 7.2)]
-
What role do aerosols play in the moist thermodynamics of WDs? Are regions or months of high aerosol optical depth associated with increased WD precipitation? How have observed trends in different aerosol species affected WD activity? [“Instrumental and reanalysis records” (Sect. 7.2)]
-
What are the respective roles of interdecadal variability and climate change in recent observations of seasonal and regional trends in WD frequency? [“Instrumental and reanalysis records” (Sect. 7.2)]
-
What is the impact of climate change on WD intensity, and why? [“Instrumental and reanalysis records” (Sect. 7.2) and “Future projections” (Sect. 7.3)]
-
What role have WDs played in supporting or destroying the Karakoram Anomaly in the last century? How is this likely to change over the coming century? [“Instrumental and reanalysis records” (Sect. 7.2) and “Future projections” (Sect. 7.3)]
-
Only two studies have investigated in detail how WD behaviour will change in future climate scenarios, and they are in disagreement. More refined studies, looking at a broad range of WD characteristics, are needed to resolve this. [“Future projections” (Sect. 7.3)]
-
Which has the greater impact on trends in winter precipitation over the western Himalaya and surrounding region – changes to thermodynamics or changes in atmospheric circulation? [“Future projections” (Sect. 7.3)]
-
There is also only a weak consensus on the projected future decrease of winter precipitation in the western Himalaya. Studies leveraging high-resolution models that are capable of resolving orographic feedbacks are needed to make more robust estimates of these changes, both in the near future and far future. [“Future projections” (Sect. 7.3)]
For more open research questions relating to the hydroclimate of the Indus region, the reader is encouraged to read Orr et al. (2022).
The WD track data used for summary figures throughout are available from https://doi.org/10.5281/zenodo.8208019 (Hunt, 2023).
KMRH and APD conceptualised the paper. KMRH conducted the literature review, wrote the initial manuscript draft, and prepared most of the figures. JPB and AGT provided extensive detailed feedback throughout, leading to substantial improvements in structure, fine detail, and figures. JPB also helped with the literature review. GJ helped to write the section on moisture sources. P and EP reviewed the draft manuscript. RC provided the outline for the subseasonal forecasting section and the data used for Fig. 18, as well as helpful feedback on parts of the manuscript. FC provided useful early feedback on the structure and water security sections. TA provided the data for Fig. 17 and helped with the development of this figure. MSS provided useful feedback on parts of the manuscript relating to precipitation impacts. STP provided some of data needed for Fig. 24.
The contact author has declared that none of the authors has any competing interests.
Publisher's note: Copernicus Publications remains neutral with regard to jurisdictional claims made in the text, published maps, institutional affiliations, or any other geographical representation in this paper. While Copernicus Publications makes every effort to include appropriate place names, the final responsibility lies with the authors.
Kieran M. R. Hunt and Andrew G. Turner were funded in part through the Weather and Climate Science for Service Partnership (WCSSP) India, a collaborative initiative between the Met Office, supported by the UK Government's Newton Fund, and the Indian Ministry of Earth Sciences (MoES). KMRH is now supported by a NERC Independent Research Fellowship (MITRE; NE/W007924/1). Andrew G. Turner is additionally supported by the National Centre for Atmospheric Science through the NERC National Capability International Programme Award (NE/X006263/1). Jean-Philippe Baudouin would like to acknowledge financial support from the German Federal Ministry of Education and Research (BMBF), which funded the PalMod project (subproject no. 01LP1926C). KMRH wishes to thank Ashis Kumar (Aryabhatta Research Institute of Observational Sciences) for providing the data for Fig. 6.
This research has been supported by the Natural Environment Research Council (grant no. NE/W007924/1) and the Weather and Climate Science for Service Partnership (WCSSP) India, a collaborative initiative between the Met Office, supported by the UK Government's Newton Fund, and the Indian Ministry of Earth Sciences (MoES).
This paper was edited by Juliane Schwendike and reviewed by four anonymous referees.
Abbas, A., Ullah, S., Ullah, W., Zhao, C., Karim, A., Waseem, M., Bhatti, A. S., Ali, G., Jan, M. A., and Ali, A.: Characteristics of winter precipitation over Pakistan and possible causes during 1981–2018, Water, 15, 2420, https://doi.org/10.3390/w15132420 , 2023. a, b
Adam, J. C. and Lettenmaier, D. P.: Adjustment of global gridded precipitation for systematic bias, J. Geophys. Res.-Atmos., 108, 4257, https://doi.org/10.1029/2002JD002499, 2003. a
Aemisegger, F., Pfahl, S., Sodemann, H., Lehner, I., Seneviratne, S. I., and Wernli, H.: Deuterium excess as a proxy for continental moisture recycling and plant transpiration, Atmos. Chem. Phys., 14, 4029–4054, https://doi.org/10.5194/acp-14-4029-2014, 2014. a
Aggarwal, D., Chakraborty, R., and Attada, R.: Investigating bi-decadal precipitation changes over the Northwest Himalayas during the pre-monsoon: role of Pacific decadal oscillations, Clim. Dynam., 62, 1203–1218, 2024. a
Agrawal, K. C.: An introduction of avalanche forecasting and future trends, 71–78, New Age International Pvt. Ltd New Delhi, 1999. a
Ahmad, I. and Sadiq, N.: Spatio-Temporal analysis of Western Disturbances over Pakistan, The Nucleus, 49, 329–338, 2012. a
Ahmad, S., Zhu, L., Yasmeen, S., Zhang, Y., Li, Z., Ullah, S., Han, S., and Wang, X.: A 424-year tree-ring-based Palmer Drought Severity Index reconstruction of Cedrus deodara D. Don from the Hindu Kush range of Pakistan: linkages to ocean oscillations, Clim. Past, 16, 783–798, https://doi.org/10.5194/cp-16-783-2020, 2020. a
Ahmed, K., Shahid, S., and Nawaz, N.: Impacts of climate variability and change on seasonal drought characteristics of Pakistan, Atmos. Res., 214, 364–374, 2018. a
Ahmed, K., Shahid, S., Wang, X., Nawaz, N., and Khan, N.: Spatiotemporal changes in aridity of Pakistan during 1901–2016, Hydrol. Earth Syst. Sci., 23, 3081–3096, https://doi.org/10.5194/hess-23-3081-2019, 2019. a
Ahmed, M., Das, B., Lotus, S., and Ali, M.: A study on frequency of western disturbances and precipitation trends over Jammu & Kashmir, India: 1980–2019, Mausam, 73, 283–294, 2022. a, b, c
Aichner, B., Feakins, S. J., Lee, J. E., Herzschuh, U., and Liu, X.: High-resolution leaf wax carbon and hydrogen isotopic record of the late Holocene paleoclimate in arid Central Asia, Clim. Past, 11, 619–633, https://doi.org/10.5194/cp-11-619-2015, 2015. a
Albrecht, R. I., Goodman, S. J., Buechler, D. E., Blakeslee, R. J., and Christian, H. J.: Where are the lightning hotspots on Earth?, B. Am. Meteorol. Soc., 97, 2051–2068, 2016. a
Almazroui, M., Saeed, S., Saeed, F., Islam, M. N., and Ismail, M.: Projections of precipitation and temperature over the South Asian countries in CMIP6, Earth Syst. Environ., 4, 297–320, 2020. a
Andermann, C., Bonnet, S., and Gloaguen, R.: Evaluation of precipitation data sets along the Himalayan front, Geochem. Geophys. Geosyst., 12, Q07023, https://doi.org/10.1029/2011GC003513, 2011. a
Archer, D. R. and Fowler, H. J.: Spatial and temporal variations in precipitation in the Upper Indus Basin, global teleconnections and hydrological implications, Hydrol. Earth Syst. Sci., 8, 47–61, https://doi.org/10.5194/hess-8-47-2004, 2004. a, b
Arulalan, T., AchutaRao, K., Hunt, K. M. R., Turner, A. G., Mitra, A. K., and Sarkar, A.: Prediction of western disturbances tracks using NEPS, https://www.ncmrwf.gov.in/event/emmda/Abstracts/TArulalan_Abstract_EMMDA.pdf (last access: 1 January 2025), 2020. a, b
Athira, K., Attada, R., and Rao, V. B.: Synoptic dynamics of cold waves over north India: Underlying mechanisms of distinct cold wave conditions, Weather and Climate Extremes, 43, 100641, https://doi.org/10.1016/j.wace.2024.100641, 2024. a, b
Attada, R., Dasari, H. P., Chowdary, J. S., Yadav, R. K., Knio, O., and Hoteit, I.: Surface air temperature variability over the Arabian Peninsula and its links to circulation patterns, Int. J. Climatol., 39, 445–464, 2019. a, b
Azadi, M., Mohanty, U. C., Madan, O. P., and Padmanabhamurty, B.: Prediction of precipitation associated with a western disturbance using a high-resolution regional model: role of parameterisation of physical processes, Meteorol. Appl., 9, 317–326, 2002. a
Bajpai, O., Pandey, J., and Chaudhary, L. B.: Consequences of western disturbance-triggered cooling on the flowering of tree species in the Himalayan Terai region, Current Sci., 109, 1781–1782, 2015. a
Bajpai, R. and Kar, R.: Modern pollen deposition in glacial settings in the Himalaya (India): abundance of Pinus pollen and its significance, Palynology, 42, 475–482, 2018. a
Bali, R., Nawaz Ali, S., Bera, S. K., Patil, S. K., Agarwal, K. K., and Nautiyal, C. M.: Impact of Anthropocene vis-a-vis Holocene climatic changes on central Indian Himalayan glaciers, in: Engineering Geology for Society and Territory-Volume 1: Climate Change and Engineering Geology, 467–471, Springer, https://doi.org/10.1007/978-3-319-09300-0_89, 2015. a
Ballav, S., Mukherjee, S., Gosavi, V., and Dimri, A. P.: Projected changes in winter-season wet days over the Himalayan region during 2020–2099, Theor. Appl. Climatol., 146, 883–895, 2021. a
Ballesteros-Cánovas, J. A., Trappmann, D., Madrigal-González, J., Eckert, N., and Stoffel, M.: Climate warming enhances snow avalanche risk in the Western Himalayas, P. Natl. Acad. Sci. USA, 115, 3410–3415, 2018. a
Bamzai, A. S. and Shukla, J.: Relation between Eurasian snow cover, snow depth, and the Indian summer monsoon: An observational study, J. Climate, 12, 3117–3132, 1999. a
Banerjee, D. and Singh, C.: An appraisal of seasonal precipitation dynamics over the North-West Himalayan region under future warming scenarios, Int. J. Climatol., 42, 2328–2350, 2022. a, b
Banerji, U. S., Bhushan, R., and Jull, A. J. T.: Signatures of global climatic events and forcing factors for the last two millennia from the active mudflats of Rohisa, southern Saurashtra, Gujarat, western India, Quaternary Int., 507, 172–187, 2019. a
Barros, A. P., Kim, G., Williams, E., and Nesbitt, S. W.: Probing orographic controls in the Himalayas during the monsoon using satellite imagery, Nat. Hazards Earth Syst. Sci., 4, 29–51, https://doi.org/10.5194/nhess-4-29-2004, 2004. a
Barros, A. P., Chiao, S., Lang, T. J., Burbank, D., and Putkonen, J.: From weather to climate-seasonal and interannual variability of storms and implications for erosion processes in the Himalaya, Special Papers-Geological Society of America, 398, 17, 2006. a
Bashir, F., Zeng, X., Gupta, H., and Hazenberg, P.: A hydrometeorological perspective on the Karakoram anomaly using unique valley-based synoptic weather observations, Geophys. Res. Lett., 44, 10–470, 2017. a
Basu, S., Bieniek, P. A., and Deoras, A.: An investigation of reduced western disturbance activity over Northwest India in November–December 2015 compared to 2014 – A case study, Asia-Pac. J. Atmos. Sci., 53, 75–83, 2017. a, b, c
Bates, J.: Kitchen gardens, wild forage and tree fruits: A hypothesis on the role of the Zaid season in the Indus civilisation (c. 3200–1300 BCE), Archaeological Research in Asia, 21, 100175, https://doi.org/10.1016/j.ara.2019.100175, 2020. a
Battula, S. B., Siems, S., and Mondal, A.: Dynamical and thermodynamical interactions in daily precipitation regimes in the Western Himalayas, Int. J. Climatol., 42, 4909–4924, 2022. a
Baudouin, J.-P., Herzog, M., and Petrie, C. A.: Cross-validating precipitation datasets in the Indus River basin, Hydrol. Earth Syst. Sci., 24, 427–450, https://doi.org/10.5194/hess-24-427-2020, 2020a. a, b
Baudouin, J.-P., Herzog, M., and Petrie, C. A.: Contribution of cross-barrier moisture transport to precipitation in the upper Indus River Basin, Mon. Weather Rev., 148, 2801–2818, 2020b. a, b, c, d
Baudouin, J.-P., Herzog, M., and Petrie, C. A.: Synoptic processes of winter precipitation in the Upper Indus Basin, Weather Clim. Dynam., 2, 1187–1207, https://doi.org/10.5194/wcd-2-1187-2021, 2021. a, b, c, d, e, f, g, h, i, j, k, l, m, n, o, p, q, r
Bedekar, V. C., Dekate, M. V., and Banerjee, A. K. (Eds.): Heat and cold waves in India, India Meteorological Department Forecasting Manual, 4, 63, 1974. a
Benn, D. I. and Owen, L. A.: The role of the Indian summer monsoon and the mid-latitude westerlies in Himalayan glaciation: review and speculative discussion, J. Geol. Soc., 155, 353–363, 1998. a, b, c
Bhan, S. C., Paul, S., Chakravarthy, K., Saxena, R., Ray, K., and Gopal, N. K.: Climatology of tornadoes over northwest India and Pakistan; and meteorological analysis of recent tornadoes over the region, Journal of Indian Geophysical Union, 20, 75–88, 2016. a
Bharali, C., Barth, M., Kumar, R., Ghude, S. D., Sinha, V., and Sinha, B.: Role of atmospheric aerosols in severe winter fog over the Indo-Gangetic Plain of India: a case study, Atmos. Chem. Phys., 24, 6635–6662, https://doi.org/10.5194/acp-24-6635-2024, 2024. a, b
Bharati, P., Deb, P., Hunt, K., Orr, A., and Dash, M. K.: ENSO-induced latitudinal variation of the subtropical jet modulates extreme winter precipitation over the Western Himalaya, Adv. Atmos. Sci., in press, 2025. a, b, c
Bhardwaj, P., Singh, O., and Kumar, D.: Spatial and temporal variations in thunderstorm casualties over India, Singapore J. Trop. Geo., 38, 293–312, 2017. a
Bhat, M. S., Mir, S., Parrey, H. A., Thoker, I. A., and Shah, S. A.: Climate change, hailstorm incidence, and livelihood security: a perspective from Kashmir valley India, Nat. Hazards, 120, 2803–2827, 2024. a, b, c
Bhattacharyya, A., Dhyani, R., Joshi, R., Shekhar, M., Kuniyal, J. C., Ranhotra, P. S., and Singh, S. P.: Is survival of Himalayan Cedar (Cedrus deodara) threatened? An evaluation based on predicted scenarios of its growth trend under future climate change, Sci. Total Environ., 882, 163630, https://doi.org/10.1016/j.scitotenv.2023.163630, 2023. a
Bhutiyani, M. R., Kale, V. S., and Pawar, N. J.: Climate change and the precipitation variations in the northwestern Himalaya: 1866–2006, Int. J. Climatol., 30, 535–548, 2010. a
Biemans, H., Siderius, C., Lutz, A. F., Nepal, S., Ahmad, B., Hassan, T., von Bloh, W., Wijngaard, R. R., Wester, P., Shrestha, A. B., and Immerzeel, W. W.: Importance of snow and glacier meltwater for agriculture on the Indo-Gangetic Plain, Nat. Sustain., 2, 594–601, 2019. a, b, c
Bilal, H., Chamhuri, S., Mokhtar, M. B., and Kanniah, K. D.: Recent snow cover variation in the upper Indus basin of Gilgit Baltistan, Hindukush Karakoram Himalaya, J. Mount. Sci., 16, 296–308, 2019. a
Bolch, T., Kulkarni, A., Kääb, A., Huggel, C., Paul, F., Cogley, J. G., Frey, H., Kargel, J. S., Fujita, K., Scheel, M., Bajracharya, S., and Stoffel, M.: The state and fate of Himalayan glaciers, Science, 336, 310–314, 2012. a, b
Böll, A., Lückge, A., Munz, P., Forke, S., Schulz, H., Ramaswamy, V., Rixen, T., Gaye, B., and Emeis, K.-C.: Late Holocene primary productivity and sea surface temperature variations in the northeastern Arabian Sea: Implications for winter monsoon variability, Paleoceanography, 29, 778–794, 2014. a
Bookhagen, B. and Burbank, D. W.: Toward a complete Himalayan hydrological budget: Spatiotemporal distribution of snowmelt and rainfall and their impact on river discharge, J. Geophys. Res.-Earth Surf., 115, F03019, https://doi.org/10.1029/2009JF001426, 2010. a
Boschi, R. and Lucarini, V.: Water pathways for the Hindu-Kush-Himalaya and an analysis of three flood events, Atmosphere, 10, 489, https://doi.org/10.3390/atmos10090489, 2019. a, b
Boucher, M.-A., Anctil, F., Perreault, L., and Tremblay, D.: A comparison between ensemble and deterministic hydrological forecasts in an operational context, Adv. Geosci., 29, 85–94, https://doi.org/10.5194/adgeo-29-85-2011, 2011. a
Bracegirdle, T. J. and Gray, S. L.: The dynamics of a polar low assessed using potential vorticity inversion, Q. J. Roy. Meteor. Soc., 135, 880–893, 2009. a
Brayshaw, D. J., Hoskins, B., and Blackburn, M.: The basic ingredients of the North Atlantic storm track. Part I: Land–sea contrast and orography, J. Atmos. Sci., 66, 2539–2558, 2009. a
Breeden, M. L., Hoell, A., Albers, J. R., and Slinski, K.: The monthly evolution of precipitation and warm conveyor belts during the central southwest Asia wet season, Weather Clim. Dynam., 4, 963–980, https://doi.org/10.5194/wcd-4-963-2023, 2023. a, b
Brown, E. T. and Johnson, T. C.: Coherence between tropical East African and South American records of the little ice age, Geochem. Geophys. Geosyst., 6, Q12005, https://doi.org/10.1029/2005GC000959, 2005. a
Browning, K. A.: Mesoscale Aspects of Extratropical Cyclones: An Observational Perspective, in: The Life Cycles of Extratropical Cyclones, edited by: Shapiro, M. A. and Grønås, S., American Meteorological Society, Boston, MA, https://doi.org/10.1007/978-1-935704-09-6_18, 1999. a
Brun, F., Berthier, E., Wagnon, P., Kääb, A., and Treichler, D.: A spatially resolved estimate of High Mountain Asia glacier mass balances from 2000 to 2016, Nat. Geosci., 10, 668–673, 2017. a
Byrne, M. P., Boos, W. R., and Hu, S.: Elevation-dependent warming: observations, models, and energetic mechanisms, Weather Clim. Dynam., 5, 763–777, https://doi.org/10.5194/wcd-5-763-2024, 2024. a, b
Cannon, F., Carvalho, L. M. V., Jones, C., and Bookhagen, B.: Multi-annual variations in winter westerly disturbance activity affecting the Himalaya, Clim. Dynam., 44, 441–455, 2015. a, b, c
Cannon, F., Carvalho, L. M. V., Jones, C., and Norris, J.: Winter westerly disturbance dynamics and precipitation in the western Himalaya and Karakoram: a wave-tracking approach, Theor. Appl. Climatol., 125, 27–44, 2016. a, b, c, d
Carolin, S. A., Walker, R. T., Day, C. C., Ersek, V., Sloan, R. A., Dee, M. W., Talebian, M., and Henderson, G. M.: Precise timing of abrupt increase in dust activity in the Middle East coincident with 4.2 ka social change, P. Natl. Acad. Sci. USA, 116, 67–72, 2019. a
Carvalho, L. M. V. and Jones, C.: A satellite method to identify structural properties of mesoscale convective systems based on the maximum spatial correlation tracking technique (MASCOTTE), J. Appl. Meteorol. Climatol., 40, 1683–1701, 2001. a
Catto, J. L.: Extratropical cyclone classification and its use in climate studies, Rev. Geophys., 54, 486–520, 2016. a
Catto, J. L., Shaffrey, L. C., and Hodges, K. I.: Northern Hemisphere extratropical cyclones in a warming climate in the HiGEM high-resolution climate model, J. Climate, 24, 5336–5352, 2011. a
Ceccaldi, M., Delanoë, J., Hogan, R. J., Pounder, N. L., Protat, A., and Pelon, J.: From CloudSat-CALIPSO to EarthCare: Evolution of the DARDAR cloud classification and its comparison to airborne radar-lidar observations, J. Geophys. Res.-Atmos., 118, 7962–7981, 2013. a
Chand, R. and Singh, C.: Movements of western disturbance and associated cloud convection, J. Ind. Geophys. Union, 19, 62–70, 2015. a, b, c
Chang, E. K. M., Guo, Y.-J., and Xia, X.-M.: CMIP5 multimodel ensemble projection of storm track change under global warming, J. Geophys. Res., 117, D23118, https://doi.org/10.1029/2012JD018578, 2012. a
Chattopadhyay, J.: Power spectrum analysis of atmospheric ozone content over north India, Pure Appl. Geophys., 83, 111–119, 1970. a, b
Chauhan, A. S., Maurya, R. K. S., Rani, A., Malik, A., Kisi, O., and Danodia, A.: Rainfall dynamics observed over India during last century (1901–2020) using innovative trend methodology, Water Supply, 22, 6909–6944, 2022. a
Cheng, H., Sinha, A., Verheyden, S., Nader, F. H., Li, X. L., Zhang, P. Z., Yin, J. J., Yi, L., Peng, Y. B., Rao, Z. G., Ning, Y. F., and Edwards, R. L.: The climate variability in northern Levant over the past 20,000 years, Geophys. Res. Lett., 42, 8641–8650, 2015. a
Chettri, N., Adhikari, B., Chaudhary, S., and Wangchuk, K.: Changing discourses in the third pole: A systematic review of climate change impact on biodiversity in the Hindu Kush Himalaya, Ecol. Indic., 155, 111 046, 2023. a
Chevuturi, A. and Dimri, A. P.: Investigation of Uttarakhand (India) disaster-2013 using weather research and forecasting model, Nat. Hazards, 82, 1703–1726, 2016. a, b, c, d
Chevuturi, A., Dimri, A. P., and Thayyen, R. J.: Climate change over Leh (Ladakh), India, Theor. Appl. Climatol., 131, 531–545, 2018. a, b
Chinthala, B. D., Grießinger, J., Ranhotra, P. S., Tomar, N., Singh, C., and Bräuning, A.: Tree-Ring Oxygen Isotope Variations in Subalpine Firs from the Western Himalaya Capture Spring Season Temperature Signals, Forests, 13, 437, https://doi.org/10.3390/f13030437, 2022. a, b
Chinthala, B. D., Ranhotra, P. S., Grießinger, J., Singh, C. P., and Bräuning, A.: Himalayan fir reveals moist phase during Little Ice Age in the Kashmir region of the western Himalayas, Quaternary Sci. Rev., 312, 108167, https://doi.org/10.1016/j.quascirev.2023.108167, 2023. a
Chitlangia, P. R.: Mean model of western depression, Indian Journal of Meteorology, Hydrol. Geophys., 87, 157–162, 1976. a, b
Cullen, N. J. and Conway, J. P.: A 22 month record of surface meteorology and energy balance from the ablation zone of Brewster Glacier, New Zealand, J. Glaciol., 61, 931–946, 2015. a
Dacre, H. F.: A review of extratropical cyclones: Observations and conceptual models over the past 100 years, Weather, 75, 4–7, 2020. a
Dacre, H. F. and Pinto, J. G.: Serial clustering of extratropical cyclones: A review of where, when and why it occurs, NPJ Climate and Atmospheric Science, 3, 1–10, 2020. a
Dacre, H. F., Hawcroft, M. K., Stringer, M. A., and Hodges, K. I.: An Extratropical Cyclone Atlas: A Tool for Illustrating Cyclone Structure and Evolution Characteristics, B. Am. Meteorol. Soc., 93, 1497–1502, https://doi.org/10.1175/BAMS-D-11-00164.1, 2012. a, b, c
Dahri, Z. H., Moors, E., Ludwig, F., Ahmad, S., Khan, A., Ali, I., and Kabat, P.: Adjustment of measurement errors to reconcile precipitation distribution in the high-altitude Indus basin, Int. J. Climatol., 38, 3842–3860, 2018. a
Dansgaard, W.: Stable isotopes in precipitation, Tellus, 16, 436–468, 1964. a, b
Dar, J.: Western disturbances alter the trend of winter precipitation and its extremes over Northwest Himalayas: Kashmir Himalaya, Environ. Sci. Pollut. Res., 30, 83439–83451, 2023. a, b
Dar, S. S., Ghosh, P., and Hillaire-Marcel, C.: Convection, terrestrial recycling and oceanic moisture regulate the isotopic composition of precipitation at Srinagar, Kashmir, J. Geophys. Res.-Atmos., 126, e2020JD032853, https://doi.org/10.1029/2020JD032853, 2021. a, b, c
Das, L. and Meher, J. K.: Drivers of climate over the Western Himalayan region of India: A review, Earth-Sci. Rev., 198, 102935, https://doi.org/10.1016/j.earscirev.2019.102935, 2019. a, b
Das, M. R., Mukhopadhyay, R. K., Dandekar, M. M., and Kshirsagar, S. R.: Pre-monsoon western disturbances in relation to monsoon rainfall, its advancement over NW India and their trends, Current Sci., 82, 1320–1321, 2002. a, b, c
Das, S., Singh, S. V., Rajagopal, E. N., and Gall, R.: Mesoscale modeling for mountain weather forecasting over the Himalayas, B. Am. Meteorol. Soc., 84, 1237–1244, 2003. a
Das, S., Ashrit, R., and Moncrieff, M. W.: Simulation of a Himalayan cloudburst event, J. Earth Syst. Sci., 115, 299–313, 2006. a, b
Das, S., Mohanty, U. C., Tyagi, A., Sikka, D. R., Joseph, P. V., Rathore, L. S., Habib, A., Baidya, S. K., Sonam, K., and Sarkar, A.: The SAARC STORM: a coordinated field experiment on severe thunderstorm observations and regional modeling over the South Asian Region, B. Am. Meteorol. Soc., 95, 603–617, 2014. a
Dasgupta, M., Das, S., and Ashrit, R.: MM5 3D-Var data assimilation and forecast system over Indian Subcontinent: Results from recent experiments, in: 5th WRF/14th MM5 Users' Workshop NCAR, 22–25 June 2004, Boulder, Colorado, USA, 2004. a
Dash, S. K. and Chakrapani, B.: Simulation of a winter circulation over India using a global spectral model, Proceedings of the Indian Academy of Sciences-Earth and Planetary Sciences, 98, 189–205, 1989. a
Dash, S. K. and Mamgain, A.: Changes in the frequency of different categories of temperature extremes in India, J. Appl. Meteorol. Climatol., 50, 1842–1858, 2011. a
Datta, R. K. and Gupta, M. G.: Synoptic study of the formation and movements of western depressions, Indian J. Meteorol. Geophys, 18, 45–50, 1967. a, b, c, d
Davis, C. A.: Piecewise potential vorticity inversion, J. Atmos. Sci., 49, 1397–1411, 1992. a
De, U. S., Dube, R. K., and Rao, G. S. P.: Extreme weather events over India in the last 100 years, J. Ind. Geophys. Union, 9, 173–187, 2005. a, b
de Kok, R. J., Tuinenburg, O. A., Bonekamp, P. N. J., and Immerzeel, W. W.: Irrigation as a potential driver for anomalous glacier behavior in High Mountain Asia, Geophys. Res. Lett., 45, 2047–2054, 2018. a
de Vernal, A., Gersonde, R., Goosse, H., Seidenkrantz, M.-S., and Wolff, E. W.: Sea ice in the paleoclimate system: the challenge of reconstructing sea ice from proxies–an introduction, Quaternary Sci. Rev., 79, 1–8, 2013. a
Dehecq, A., Gourmelen, N., Gardner, A. S., Brun, F., Goldberg, D., Nienow, P. W., Berthier, E., Vincent, C., Wagnon, P., and Trouvé, E.: Twenty-first century glacier slowdown driven by mass loss in High Mountain Asia, Nat. Geosci., 12, 22–27, 2019. a
Demske, D., Tarasov, P. E., Wünnemann, B., and Riedel, F.: Late glacial and Holocene vegetation, Indian monsoon and westerly circulation in the Trans-Himalaya recorded in the lacustrine pollen sequence from Tso Kar, Ladakh, NW India, Palaeogeogr. Palaeocl., 279, 172–185, 2009. a
Deng, H. and Ji, Z.: Warming and Wetting will continue over the Tibetan Plateau in the Shared Socioeconomic Pathways, Plos one, 18, e0289589, https://doi.org/10.1371/journal.pone.0289589, 2023. a
Devi, U., Shekhar, M. S., Singh, G. P., and Dash, S. K.: Statistical method of forecasting of seasonal precipitation over the Northwest Himalayas: North Atlantic Oscillation as precursor, Pure Appl. Geophys., 177, 3501–3511, 2020. a
Dezfuli, A.: Rare atmospheric river caused record floods across the Middle East, B. Am. Meteorol. Soc., 101, E394–E400, 2020. a
Dezfuli, A., Bosilovich, M. G., and Barahona, D.: A dusty atmospheric river brings floods to the Middle East, Geophys. Res. Lett., 48, e2021GL095441, https://doi.org/10.1029/2021GL095441, 2021. a
Dimri, A. P.: Impact of horizontal model resolution and orography on the simulation of a western disturbance and its associated precipitation, Meteorol. Appl., 11, 115–127, 2004. a, b
Dimri, A. P.: Surface and upper air fields during extreme winter precipitation over the western Himalayas, Pure Appl. Geophys., 163, 1679–1698, 2006. a, b, c
Dimri, A. P.: Diagnostic studies of an active western disturbance over western Himalaya, Mausam, 59, 227–236, 2008. a
Dimri, A. P.: Intraseasonal oscillation associated with the Indian winter monsoon, J. Geophys. Res.-Atmos., 118, 1189–1198, 2013. a, b
Dimri, A. P.: Warm pool/cold tongue El Niño and Indian winter Monsoon, Meteorol. Atmos. Phys., 129, 321–331, 2017. a
Dimri, A. P.: Decoding the Karakoram Anomaly, Sci. Total Environ., 788, 147864, https://doi.org/10.1016/j.scitotenv.2021.147864, 2021. a
Dimri, A. P. and Chevuturi, A.: Model sensitivity analysis study for western disturbances over the Himalayas, Meteorol. Atmos. Phys., 123, 155–180, 2014a. a
Dimri, A. P. and Chevuturi, A.: Model sensitivity analysis study for western disturbances over the Himalayas, Meteorol. Atmos. Phys., 123, 155–180, 2014b. a, b, c, d
Dimri, A. P. and Chevuturi, A. (Eds.): Western Disturbances-An Indian Meteorological Perspective, Springer, Switzerland, https://doi.org/10.1007/978-3-319-26737-1, 2016. a, b
Dimri, A. P. and Mohanty, U. C.: Snowfall statistics of some SASE field stations in J&K, Defence Science Journal, 49, 437, 1999. a
Dimri, A. P. and Niyogi, D.: Regional climate model application at subgrid scale on Indian winter monsoon over the western Himalayas, Int. J. Climatol., 33, 2185–2205, 2012. a, b, c
Dimri, A. P., Niyogi, D., Barros, A. P., Ridley, J., Mohanty, U. C., Yasunari, T., and Sikka, D. R.: Western disturbances: a review, Rev. Geophys., 53, 225–246, 2015. a, b, c, d
Dimri, A. P., Yasunari, T., Kotlia, B. S., Mohanty, U. C., and Sikka, D. R.: Indian winter monsoon: Present and past, Earth-Sci. Rev., 163, 297–322, 2016. a
Dimri, A. P., Palazzi, E., and Daloz, A. S.: Elevation dependent precipitation and temperature changes over Indian Himalayan region, Clim. Dynam., 59, 1–21, 2022. a, b, c
Dimri, A. P., Pooja, Jeelani, G., and Mohanty, U. C.: Western disturbances vs Non-western disturbances days winter precipitation, Clim. Dynam., 61, 4825–4847, 2023. a, b, c, d, e
Dixit, Y., Hodell, D. A., and Petrie, C. A.: Abrupt weakening of the summer monsoon in northwest India 4100 yr ago, Geology, 42, 339–342, 2014. a
Dixon, M. and Wiener, G.: TITAN: Thunderstorm identification, tracking, analysis, and nowcasting – A radar-based methodology, Journal of atmospheric and oceanic technology, 10, 785–797, 1993. a
Doblas-Reyes, F., Sörensson, A., Almazroui, M., Dosio, A., Gutowski, W., Haarsma, R., Hamdi, R., Hewitson, B., Kwon, W.-T., Lamptey, B., Maraun, D., Stephenson, T., Takayabu, I., Terray, L., Turner, A., and Zuo, Z.: Linking Global to Regional Climate Change, 1363–1512, Cambridge University Press, Cambridge, United Kingdom and New York, NY, USA, https://doi.org/10.1017/9781009157896.012, 2021. a, b, c
Duan, A. and Wu, G.: Change of cloud amount and the climate warming on the Tibetan Plateau, Geophys. Res. Lett., 33, L22704, https://doi.org/10.1029/2006GL027946, 2006. a
Dutta, D., Kasimahanthi, A. J., Devarajan, P. K., George, J. P., and Rajagopal, E. N.: Winter hailstorms signatures by C-band polarimetric radar at Delhi, J. Appl. Remote Sens., 10, 026022, https://doi.org/10.1117/1.JRS.10.026022, 2016. a
Dutta, D., Routray, A., Lodh, A., George, J. P., and Mitra, A. K.: Impact of DWR radial wind on simulation of western disturbances using NCUM-R modeling system, Meteorol. Atmos. Phys., 134, 81, https://doi.org/10.1007/s00703-022-00919-0, 2022. a
Ehsan, M. A., Kucharski, F., and Almazroui, M.: Potential predictability of boreal winter precipitation over central-southwest Asia in the North American multi-model ensemble, Clim. Dynam., 54, 473–490, 2020. a
Elahi, E., Abro, M. I., Khaskheli, M. A., Kandhro, G. A., Zehra, T., Ali, S., Shaikh, M. N., Laghari, B. A., Hassan, M., and Memon, M. A.: Long-term evaluation of rainfall in the arid region of Pakistan using multi-source data, Theor. Appl. Climatol., 155, 2819–2840, 2023. a
Emanuel, K.: A statistical analysis of tropical cyclone intensity, Mon. Weather Rev., 128, 1139–1152, 2000. a
Esfandiari, N. and Lashkari, H.: The effect of atmospheric rivers on cold-season heavy precipitation events in Iran, J. Water Clim. Change, 12, 596–611, 2021. a
Farinotti, D., Immerzeel, W. W., de Kok, R. J., Quincey, D. J., and Dehecq, A.: Manifestations and mechanisms of the Karakoram glacier Anomaly, Nat. Geosci., 13, 8–16, 2020. a, b
Ferraro, R. R., Smith, E. A., Berg, W., and Huffman, G. J.: A screening methodology for passive microwave precipitation retrieval algorithms, J. Atmos. Sci., 55, 1583–1600, 1998. a
Filippi, L., Palazzi, E., von Hardenberg, J., and Provenzale, A.: Multidecadal variations in the relationship between the NAO and winter precipitation in the Hindu Kush–Karakoram, J. Climate, 27, 7890–7902, 2014. a, b
Fogt, R. L. and Marshall, G. J.: The Southern Annular Mode: variability, trends, and climate impacts across the Southern Hemisphere, Wiley Interdisciplinary Reviews: Climate Change, 11, e652, https://doi.org/10.1002/wcc.652, 2020. a
Forke, S., Rixen, T., Burdanowitz, N., Lückge, A., Ramaswamy, V., Munz, P., Wilhelms-Dick, D., Vogt, C., Kasten, S., and Gaye, B.: Sources of laminated sediments in the northeastern Arabian Sea off Pakistan and implications for sediment transport mechanisms during the late Holocene, The Holocene, 29, 130–144, 2019. a, b
Forsythe, N., Fowler, H. J., Li, X.-F., Blenkinsop, S., and Pritchard, D.: Karakoram temperature and glacial melt driven by regional atmospheric circulation variability, Nat. Clim. Change, 7, 664–670, 2017. a, b
Fowler, H. J. and Archer, D. R.: Conflicting signals of climatic change in the Upper Indus Basin, J. Climate, 19, 4276–4293, 2006. a
Ganju, A. and Dimri, A. P.: Prevention and mitigation of avalanche disasters in western Himalayan region, Nat. Hazards, 31, 357–371, 2004. a, b
Ganju, A., Nagar, Y. C., Sharma, L. N., Sharma, S., and Juyal, N.: Luminescence chronology and climatic implication of the late quaternary glaciation in the Nubra valley, Karakoram Himalaya, India, Palaeogeogr. Palaeocl., 502, 52–62, 2018. a, b
Gao, Y., Chen, F., Lettenmaier, D. P., Xu, J., Xiao, L., and Li, X.: Does elevation-dependent warming hold true above 5000 m elevation? Lessons from the Tibetan Plateau, npj Climate and Atmospheric Science, 1, 19, https://doi.org/10.1038/s41612-018-0030-z, 2018. a
Gardelle, J., Berthier, E., and Arnaud, Y.: Slight mass gain of Karakoram glaciers in the early twenty-first century, Nat. Geosci., 5, 322–325, 2012. a
Gardelle, J., Berthier, E., Arnaud, Y., and Kääb, A.: Region-wide glacier mass balances over the Pamir-Karakoram-Himalaya during 1999–2011, The Cryosphere, 7, 1263–1286, https://doi.org/10.5194/tc-7-1263-2013, 2013. a
Garvert, M. F., Smull, B., and Mass, C.: Multiscale mountain waves influencing a major orographic precipitation event, J. Atmos. Sci., 64, 711–737, 2007. a
Gat, J. R. and Carmi, I.: Evolution of the isotopic composition of atmospheric waters in the Mediterranean Sea area, J. Geophys. Res., 75, 3039–3048, 1970. a
Ghatak, D., Sinsky, E., and Miller, J.: Role of snow-albedo feedback in higher elevation warming over the Himalayas, Tibetan Plateau and Central Asia, Environ. Res. Lett., 9, 114008, https://doi.org/10.1088/1748-9326/9/11/114008, 2014. a
Ghimire, S., Choudhary, A., and Dimri, A. P.: Assessment of the performance of CORDEX-South Asia experiments for monsoonal precipitation over the Himalayan region during present climate: part I, Clim. Dynam., 50, 2311–2334, 2018. a
Ghude, S. D., Bhat, G. S., Prabhakaran, T., Jenamani, R. K., Chate, D. M., Safai, P. D., Karipot, A. K., Konwar, M., Pithani, P., Sinha, V., Rao, P. S. P., Dixit, S. A., Tiwari, S., Todekar, K., Varpe, S., Srivastava, A. K., Bisht, D. S., Murugavel, P., Ali, K., Mina, U., Dharua, M., Jaya Rao, Y., Padmakumari, B., Hazra, A., Nigam, N., Shende, U., Lal, D. M., Chandra, B. P., Mishra, A. K., Kumar, A., Hakkim, H., Pawar, H., Acharja, P., Kulkarni, R., Subharthi, C., Balaji, B., Varghese, M., Bera, S., and Rajeevan, M.: Winter fog experiment over the Indo-Gangetic plains of India, Current Sci., 112, 767–784, 2017. a
Giesche, A., Staubwasser, M., Petrie, C. A., and Hodell, D. A.: Indian winter and summer monsoon strength over the 4.2 ka BP event in foraminifer isotope records from the Indus River delta in the Arabian Sea, Clim. Past, 15, 73–90, https://doi.org/10.5194/cp-15-73-2019, 2019. a
Giesche, A., Hodell, D. A., Petrie, C. A., Haug, G. H., Adkins, J. F., Plessen, B., Marwan, N., Bradbury, H. J., Hartland, A., French, A. D., and Breitenbach, S. F. M.: Recurring summer and winter droughts from 4.2–3.97 thousand years ago in north India, Commun. Earth Environ., 4, 103, https://doi.org/10.1038/s43247-023-00763-z, 2023. a, b
Giosan, L., Orsi, W. D., Coolen, M., Wuchter, C., Dunlea, A. G., Thirumalai, K., Munoz, S. E., Clift, P. D., Donnelly, J. P., Galy, V., and Fuller, D. Q.: Neoglacial climate anomalies and the Harappan metamorphosis, Clim. Past, 14, 1669–1686, https://doi.org/10.5194/cp-14-1669-2018, 2018. a, b, c, d, e
Greene, A. M. and Robertson, A. W.: Interannual and low-frequency variability of Upper Indus Basin winter/spring precipitation in observations and CMIP5 models, Clim. Dynam., 49, 4171–4188, 2017. a, b
Grotjahn, R.: Global atmospheric circulations – Observations and theories, Oxford University Press, New York, 1993. a
Gunturu, U. B. and Kumar, V.: Weakened Baroclinic Activity Causes an Abrupt Rise in Fog in the Indo-Gangetic Plain, Geophys. Res. Lett., 48, e2021GL096114, https://doi.org/10.1029/2021GL096114, 2021. a, b
Guo, D., Sun, J., Yang, K., Pepin, N., and Xu, Y.: Revisiting recent elevation-dependent warming on the Tibetan Plateau using satellite-based data sets, J. Geophys. Res.-Atmos., 124, 8511–8521, 2019. a
Guo, D., Pepin, N., Yang, K., Sun, J., and Li, D.: Local changes in snow depth dominate the evolving pattern of elevation-dependent warming on the Tibetan Plateau, Sci. Bull., 66, 1146–1150, 2021. a
Gupta, A., Rathore, L. S., Singh, S. V., and Mendiratta, N.: Performance of a global circulation model in predicting the winter systems and associated precipitation over North West India during 1994–97, in: The Himalayan Environment, 123–138, New Age Int. Publ., New Delhi, 1999. a
Gupta, J. P., Warsi, A. H., and Sharma, P.: Severe dust storm/thunderstorm activity over Uttar Pradesh on 13th May, 2018-A case study, Mausam, 74, 1141–1154, 2023. a, b
Gupta, P., Bhatla, R., Payra, S., Yadava, P. K., and Verma, S.: Cold Wave and Severe Cold Wave Events Over Indo-Gangetic Plain: Analysis and Comparison for Decadal Variability, Int. J. Earth Atmos. Sci., 5, 101–109, 2018. a
Gupta, P., Verma, S., Bhatla, R., Payra, S., and Yadava, P. K.: Long term (1984-2013) winter temperature variability and cold wave analysis over Varanasi City, J. Ind. Geophys. Un., 23, 347–355, 2019. a
Gurung, D. R., Maharjan, S. B., Shrestha, A. B., Shrestha, M. S., Bajracharya, S. R., and Murthy, M. S. R.: Climate and topographic controls on snow cover dynamics in the Hindu Kush Himalaya, Int. J. Climatol., 37, 3873–3882, 2017. a, b
Gusain, H. S., Mishra, V. D., and Bhutiyani, M. R.: Winter temperature and snowfall trends in the cryospheric region of north-west Himalaya, Mausam, 65, 425–432, 2014. a
Hammad, H. M., Ashraf, M., Abbas, F., Bakhat, H. F., Qaisrani, S. A., Mubeen, M., Fahad, S., and Awais, M.: Environmental factors affecting the frequency of road traffic accidents: a case study of sub-urban area of Pakistan, Environ. Sci. Pollut. Res., 26, 11674–11685, 2019. a
Hamzeh, M. A., Gharaie, M. H. M., Lahijani, H. A. K., Djamali, M., Harami, R. M., and Beni, A. N.: Holocene hydrological changes in SE Iran, a key region between Indian Summer Monsoon and Mediterranean winter precipitation zones, as revealed from a lacustrine sequence from Lake Hamoun, Quaternary Int., 408, 25–39, 2016. a
Hara, M., Kimura, F., and Yasunari, T.: The generation mechanism of the western disturbances over the Himalayas, in: 6th international Study Conference on GEWEX in Asia and GAME, Kyoto, Japan, https://www.researchgate.net/profile/Tetsuzo-Yasunari/publication/267828781_The_Generation_Mechanism_ of_the_Western_Disturbances_over_the_Himalayas/links/5540b 9c40cf2718618da8ef4/The-Generation-Mechanism-of-the-Western-Disturbances-over-the-Himalayas.pdf (last access: 8 January 2025), 2004. a, b, c
Hasan, M. R. and Pattnaik, S.: Characteristics of Western Disturbance intensification and associated induced circulations over the Indian Region, Nat. Hazards Res., 4, 486–497, https://doi.org/10.1016/j.nhres.2023.12.002, 2024. a, b
Hatwar, H. R., Yadav, B. P., and Rao, Y. V. R.: Prediction of western disturbances and associated weather over Western Himalayas, Current Sci, 88, 913–920, 2005. a
Haug, G. H., Hughen, K. A., Sigman, D. M., Peterson, L. C., and Röhl, U.: Southward migration of the intertropical convergence zone through the Holocene, Science, 293, 1304–1308, 2001. a
Hersbach, H., Bell, B., Berrisford, P., Hirahara, S., Horányi, A., Muñoz-Sabater, J., Nicolas, J., Peubey, C., Radu, R., Schepers, D., Simmons, A., Soci, C., Abdalla, S., Abellan, X., Balsamo, G., Bechtold, P., Biavati, G., Bidlot, J., Bonavita, M., De Chiara, G., Dahlgren, P., Dee, D.,Diamantakis, M., Dragani, R., Flemming, J., Forbes, R., Fuentes, M., Geer, A., Haimberger, L., Healy, S., Hogan, R. J., Hólm, E., Janisková, M., Keeley, S., Laloyaux, P., Lopez, P., Lupu, C., Radnoti, G., de Rosnay, P., Rozum, I., Vamborg, F., Villaume, S., and Thépaut, J.-N.: The ERA5 global reanalysis, Q. J. Roy. Meteor. Soc., 146, 1999–2049, 2020. a
Herzschuh, U.: Palaeo-moisture evolution in monsoonal Central Asia during the last 50,000 years, Quaternary Sci. Rev., 25, 163–178, 2006. a
Hewitt, K.: The Karakoram anomaly? Glacier expansion and the `elevation effect', Karakoram Himalaya, Mount. Res. Develop., 25, 332–340, 2005. a
Hill, A. F., Rittger, K., Dendup, T., Tshering, D., and Painter, T. H.: How important is meltwater to the Chamkhar Chhu headwaters of the Brahmaputra River?, Front. Earth Sci., 8, 81, https://doi.org/10.3389/feart.2020.00081, 2020. a
Hingmire, D., Vellore, R. K., Krishnan, R., Ashtikar, N. V., Singh, B. B., Sabade, S., and Madhura, R. K.: Widespread fog over the Indo-Gangetic Plains and possible links to boreal winter teleconnections, Clim. Dynam., 52, 5477–5506, 2019. a, b
Hingmire, D., Vellore, R., Krishnan, R., Singh, M., Metya, A., Gokul, T., and Ayantika, D. C.: Climate change response in wintertime widespread fog conditions over the Indo-Gangetic Plains, Clim. Dynam., 58, 2745–2766, 2022. a
Hodges, K. I.: Feature tracking on the unit sphere, Mon. Weather Rev., 123, 3458–3465, 1995. a
Hodges, K. I.: Adaptive constraints for feature tracking, Mon. Weather Rev., 127, 1362–1373, 1999. a
Houze, R. A.: Orographic effects on precipitating clouds, Rev. Geophys., 50, RG1001, https://doi.org/10.1029/2011RG000365, 2012. a
Houze, R. A., McMurdie, L. A., Rasmussen, K. L., Kumar, A., and Chaplin, M. M.: Multiscale Aspects of the Storm Producing the June 2013 Flooding in Uttarakhand, India, Mon. Weather Rev., 145, 4447–4466, 2017. a
Hu, S. and Hsu, P.-C.: Drivers of elevation-dependent warming over the Tibetan Plateau, Atmos. Ocean. Sci. Lett., 16, 100289, https://doi.org/10.1016/j.aosl.2022.100289, 2023. a
Hunt, K. M. R.: Tracks of western disturbances (1950–2022) impacting South Asia (1.0), Zenodo [data set], https://doi.org/10.5281/zenodo.8208019, 2023. a
Hunt, K. M. R.: Increasing frequency and lengthening season of western disturbances are linked to increasing strength and delayed northward migration of the subtropical jet, Weather Clim. Dynam., 5, 345–356, https://doi.org/10.5194/wcd-5-345-2024, 2024. a, b, c, d, e, f, g, h, i, j, k, l, m, n, o, p
Hunt, K. M. R. and Fletcher, J. K.: The relationship between Indian monsoon rainfall and low-pressure systems, Clim. Dynam., 53, 1–13, 2019. a
Hunt, K. M. R. and Turner, A. G.: The Role of the Subtropical Jet in Deficient Winter Precipitation Across the Mid-Holocene Indus Basin, Geophys. Res. Lett., 46, 5452–5459, 2019. a
Hunt, K. M. R. and Zaz, S. N.: Linking the NAO to winter precipitation over the Western Himalaya through disturbances of the subtropical jet, Clim. Dynam., 60, 2389–2403, https://doi.org/10.1007/s00382-022-06450-7, 2023. a, b, c
Hunt, K. M. R., Curio, J., Turner, A. G., and Schiemann, R.: Subtropical westerly jet influence on occurrence of Western Disturbances and Tibetan Plateau Vortices, Geophys. Res. Lett., 45, 8629–8636, 2018a. a
Hunt, K. M. R., Turner, A. G., and Shaffrey, L. C.: The evolution, seasonality, and impacts of western disturbances, Q. J. Roy. Meteor. Soc., 144, 278–290, https://doi.org/10.1002/qj.3200, 2018b. a, b, c, d, e, f, g, h, i, j, k, l, m, n, o, p, q, r, s, t
Hunt, K. M. R., Turner, A. G., and Shaffrey, L. C.: Extreme daily rainfall in Pakistan and north India: scale-interactions, mechanisms, and precursors, Mon. Weather Rev., 146, 1005–1022, 2018c. a, b
Hunt, K. M. R., Turner, A. G., and Shaffrey, L. C.: Representation of western disturbances in CMIP5 models, J. Climate, 32, 1997–2011, https://doi.org/10.1175/JCLI-D-18-0420.1, 2019a. a, b, c
Hunt, K. M. R., Turner, A. G., and Shaffrey, L. C.: Falling trend of western disturbances in future climate simulations, J. Climate, 32, 5037–5051, 2019b. a, b, c, d, e, f
Hunt, K. M. R., Turner, A. G., and Shaffrey, L. C.: The impacts of climate change on the winter water cycle of the western Himalaya, Clim. Dynam., 55, 2287–2307, 2020. a, b, c, d
Hunt, K. M. R., Turner, A. G., and Schiemann, R. K. H.: How interactions between tropical depressions and western disturbances affect heavy precipitation in South Asia, Mon. Weather Rev., 149, 1801–1825, 2021. a, b, c, d
Hurley, J. V. and Boos, W. R.: A global climatology of monsoon low pressure systems, Q. J. Roy. Meteor. Soc., 141, 1049–1064, https://doi.org/10.1002/qj.2447, 2015. a
Hussain, S., Song, X.-f., Ren, G.-y., Hussain, I., Han, D.-m., and Zaman, M. H.: Evaluation of gridded precipitation data in the Hindu Kush–Karakoram–Himalaya mountainous area, Hydrol. Sci. J., 62, 2393–2405, 2017. a, b
Insel, N., Poulsen, C. J., and Ehlers, T. A.: Influence of the Andes Mountains on South American moisture transport, convection, and precipitation, Clim. Dynam., 35, 1477–1492, 2010. a
Iqbal, W., Syed, F. S., Sajjad, H., Nikulin, G., Kjellström, E., and Hannachi, A.: Mean climate and representation of jet streams in the CORDEX South Asia simulations by the regional climate model RCA4, Theor. Appl. Climatol., 129, 1–19, 2017. a
Isaak, D. J. and Luce, C. H.: Elevation-dependent warming of streams in mountainous regions: implications for temperature modeling and headwater climate refugia, Can. Water Resour. J., 48, 167–188, 2023. a
Jaiswal, A., Naja, M., Bhattacharjee, S., Tiwari, S., and Reddy, B.: Unraveling the dynamics of an intense pre-monsoon hailstorm and summer monsoon deep convective system over the central Himalayas using a VHF radar, Atmos. Res., 284, 106595, https://doi.org/10.1016/j.atmosres.2022.106595, 2023. a
Javed, A. and Kumar, P.: Characteristic Changes in the Strengthening Western Disturbances over Karakoram in Recent Decades, Asia-Pac. J. Atmos. Sci., 60, 255–270, https://doi.org/10.1007/s13143-024-00354-8, 2024. a
Javed, A., Kumar, P., Hodges, K. I., Sein, D. V., Dubey, A. K., and Tiwari, G.: Does the recent revival of Western Disturbances govern the Karakoram Anomaly?, J. Climate, 35, 4383–4402, 2022. a, b, c, d, e, f
Javed, A., Anshuman, K., Kumar, P., and Sachan, D.: The decline in western disturbance activity over Northern India in recent decades, Clim. Change, 176, 97, https://doi.org/10.1007/s10584-023-03571-8, 2023. a, b
Jeelani, G. and Deshpande, R. D.: Isotope fingerprinting of precipitation associated with western disturbances and Indian summer monsoons across the Himalayas, J. Earth Syst. Sci., 126, 1–13, 2017. a, b, c
Jeelani, G., Deshpande, R. D., Shah, R. A., and Hassan, W.: Influence of southwest monsoons in the Kashmir Valley, western Himalayas, Isot. Environ. Health S., 53, 400–412, 2017. a, b
Jeelani, G., Deshpande, R. D., Galkowski, M., and Rozanski, K.: Isotopic composition of daily precipitation along the southern foothills of the Himalayas: impact of marine and continental sources of atmospheric moisture, Atmos. Chem. Phys., 18, 8789–8805, https://doi.org/10.5194/acp-18-8789-2018, 2018. a
Jeelani, G., Shah, R. A., Deshpande, R. D., Dimri, A. P., Mal, S., and Sharma, A.: Isotopic analysis to quantify the role of the Indian monsoon on water resources of selected river basins in the Himalayas, Hydrol. Process., 35, e14406, https://doi.org/10.1002/hyp.14406, 2021. a
Joseph, S., Sahai, A. K., Sharmila, S., Abhilash, S., Borah, N., Chattopadhyay, R., Pillai, P. A., Rajeevan, M., and Kumar, A.: North Indian heavy rainfall event during June 2013: diagnostics and extended range prediction, Clim. Dynam., 44, 2049–2065, 2015. a
Joshi, L. M., Kotlia, B. S., Ahmad, S., Wu, C.-C., Sanwal, J., Raza, W., Singh, A. K., Shen, C.-C., Long, T., and Sharma, A. K.: Reconstruction of Indian monsoon precipitation variability between 4.0 and 1.6 ka BP using speleothem δ 18 O records from the Central Lesser Himalaya, India, Arab. J. Geosci., 10, 1–16, 2017. a
Joshi, S. K., Kumar, S., Sinha, R., Rai, S. P., Khobragade, S., and Rao, M. S.: Identifying moisture transport pathways for north-west India, Geol. J., 58, 4428–4440, 2023. a
Ju, J.-H. and Slingo, J.: The Asian summer monsoon and ENSO, Q. J. Roy. Meteor. Soc., 121, 1133–1168, 1995. a
Jury, M. W., Mendlik, T., Tani, S., Truhetz, H., Maraun, D., Immerzeel, W. W., and Lutz, A. F.: Climate projections for glacier change modelling over the Himalayas, Int. J. Climatol., 40, 1738–1754, 2020. a, b
Kalshetti, M., Chattopadhyay, R., Hunt, K. M. R., Phani, R., Joseph, S., Pattanaik, D. R., and Sahai, A. K.: Eddy transport, wave–mean flow interaction, and Eddy forcing during the 2013 Uttarakhand extreme event in the reanalysis and S2S retrospective forecast data, Int. J. Climatol., 2022. a, b, c
Kalsi, S. R. and Halder, S. R.: Satellite observations of interaction between tropics and mid-latitudes, Mausam, 43, 59–64, 1992. a, b, c
Kamil, S., Almazroui, M., Kang, I.-S., Hanif, M., Kucharski, F., Abid, M. A., and Saeed, F.: Long-term ENSO relationship to precipitation and storm frequency over western Himalaya–Karakoram–Hindukush region during the winter season, Clim. Dynam., 53, 5265–5278, 2019. a, b
Kanda, N., Negi, H. S., Rishi, M. S., and Kumar, A.: Performance of various gridded temperature and precipitation datasets over Northwest Himalayan Region, Environ. Res. Commun., 2, 085002, https://doi.org/10.1088/2515-7620/ab9991, 2020. a
Kar, R. and Quamar, M. F.: Late Pleistocene–Holocene vegetation and climate change from the Western and Eastern Himalaya (India): palynological perspective, Current Sci., 119, 195–218, 2020. a, b, c, d
Karki, R., ul Hasson, S., Gerlitz, L., Schickhoff, U., Scholten, T., and Böhner, J.: Quantifying the added value of convection-permitting climate simulations in complex terrain: a systematic evaluation of WRF over the Himalayas, Earth Syst. Dynam., 8, 507–528, https://doi.org/10.5194/esd-8-507-2017, 2017. a
Kattel, D. B., Yao, T., Ullah, K., and Rana, A. S.: Seasonal near-surface air temperature dependence on elevation and geographical coordinates for Pakistan, Theor. Appl. Climatol., 138, 1591–1613, 2019. a
Keller, J. H., Grams, C. M., Riemer, M., Archambault, H. M., Bosart, L., Doyle, J. D., Evans, J. L., Galarneau Jr., T. J., Griffin, K., Harr, P. A., Kitabatake, N., McTaggart-Cowan, R., Pantillon, F., Quinting, J. F., Reynolds, C. A., Ritchie, E. A., Torn, R. D., and Zhang, F.: The extratropical transition of tropical cyclones. Part II: Interaction with the midlatitude flow, downstream impacts, and implications for predictability, Mon. Weather Rev., 147, 1077–1106, 2019. a
Kemmannu, P. K. and Manjunatha, B. R.: Impacts of Aerosol Orographic Precipitation Interaction Associated with Western Disturbances over India Using Satellite Observations, Water, 15, 2901, https://doi.org/10.3390/w15162901, 2023. a
Kendon, E. J., Roberts, N. M., Senior, C. A., and Roberts, M. J.: Realism of rainfall in a very high-resolution regional climate model, J. Climate, 25, 5791–5806, 2012. a
Kew, S. F., Sprenger, M., and Davies, H. C.: Potential vorticity anomalies of the lowermost stratosphere: A 10-yr winter climatology, Mon. Weather Rev., 138, 1234–1249, 2010. a
Khan, S., Wani, Z. A., Ahmad, R., Gaira, K. S., and Verma, S.: Time series analysis of climatic variability and trends in Shiwalik to Pir Panjal mountain range in the Indian western Himalaya, Environ. Develop. Sustain., 26, 20355–20377, https://doi.org/10.1007/s10668-023-03476-7, 2023. a
Kidd, C., Becker, A., Huffman, G. J., Muller, C. L., Joe, P., Skofronick-Jackson, G., and Kirschbaum, D. B.: So, how much of the Earth’s surface is covered by rain gauges?, B. Am. Meteorol. Soc., 98, 69–78, 2017. a
Kimura, F. and Kitoh, A.: Downscaling by pseudo-global-warming method., in: The Final Report of the ICCAP, RIHN Project 1-1, 43–46, 2007. a
Kirschbaum, D., Stanley, T., and Zhou, Y.: Spatial and temporal analysis of a global landslide catalog, Geomorphology, 249, 4–15, 2015. a
Kirschbaum, D. B., Adler, R., Hong, Y., Hill, S., and Lerner-Lam, A.: A global landslide catalog for hazard applications: method, results, and limitations, Nat. Hazards, 52, 561–575, 2010. a
Konecky, B. L., Russell, J. M., Rodysill, J. R., Vuille, M., Bijaksana, S., and Huang, Y.: Intensification of southwestern Indonesian rainfall over the past millennium, Geophys. Res. Lett., 40, 386–391, 2013. a
Kong, Y.-L., Pang, Z.-H., and Froehlich, K.: Quantifying recycled moisture fraction in precipitation of an arid region using deuterium excess, Tellus B, 65, 19251, https://doi.org/10.3402/tellusb.v65i0.19251, 2013. a
Kotal, S. D., Roy, S. S., and Roy Bhowmik, S. K.: Catastrophic heavy rainfall episode over Uttarakhand during 16–18 June 2013 – observational aspects, Current Sci., 107, 234–245, 2014. a
Kotlia, B. S., Ahmad, S. M., Zhao, J.-X., Raza, W., Collerson, K. D., Joshi, L. M., and Sanwal, J.: Climatic fluctuations during the LIA and post-LIA in the Kumaun Lesser Himalaya, India: evidence from a 400 y old stalagmite record, Quaternary Int., 263, 129–138, 2012. a, b
Kotlia, B. S., Singh, A. K., Joshi, L. M., and Dhaila, B. S.: Precipitation variability in the Indian Central Himalaya during last ca. 4,000 years inferred from a speleothem record: Impact of Indian Summer Monsoon (ISM) and Westerlies, Quaternary Int., 371, 244–253, 2015. a
Kotlia, B. S., Singh, A. K., Zhao, J.-X., Duan, W., Tan, M., Sharma, A. K., and Raza, W.: Stalagmite based high resolution precipitation variability for past four centuries in the Indian Central Himalaya: Chulerasim cave re-visited and data re-interpretation, Quaternary Int., 444, 35–43, 2017. a, b
Krishnan, R., Sabin, T. P., Madhura, R. K., Vellore, R. K., Mujumdar, M., Sanjay, J., Nayak, S., and Rajeevan, M.: Non-monsoonal precipitation response over the Western Himalayas to climate change, Clim. Dynam., 52, 4091–4109, 2019a. a, b, c, d
Krishnan, R., Shrestha, A. B., Ren, G., Rajbhandari, R., Saeed, S., Sanjay, J., Syed, Md. A., Vellore, R., Xu, Y., You, Q., and Ren, Y.: Unravelling climate change in the Hindu Kush Himalaya: rapid warming in the mountains and increasing extremes, in: The Hindu Kush Himalaya Assessment, edited by: Wester, P., Mishra, A., Mukherji, A., and Shrestha, A., Springer, Cham, https://doi.org/10.1007/978-3-319-92288-1_3, 2019b. a
Kulkarni, A. V., Shirsat, T. S., Kulkarni, A., Negi, H. S., Bahuguna, I. M., and Thamban, M.: State of Himalayan cryosphere and implications for water security, Water Security, 14, 100101, https://doi.org/10.1016/j.wasec.2021.100101, 2021. a
Kumar, A., Singh, N., and Singh, A.: Observations on the distribution of clouds over northern India using joint CloudSat and CALIPSO measurements, Remote Sens. Lett., 10, 590–597, 2019a. a, b
Kumar, A., Singh, N., and Anshumali: Study on cloud types distribution over northern India using raDAR-liDAR (DARDAR), in: 2019 URSI Asia-Pacific Radio Science Conference (AP-RASC), 9–15 March 2019; New Delhi, India, 1–4, IEEE, 2019b. a
Kumar, N., Yadav, B. P., Gahlot, S., and Singh, M.: Winter frequency of western disturbances and precipitation indices over Himachal Pradesh, India: 1977–2007, Atmósfera, 28, 63–70, 2015. a, b
Kumar, N., Mohapatra, M., and Jaswal, A. K.: Meteorological features associated with unprecedented precipitation over India during 1st week of March 2015, J. Earth Syst. Sci., 126, 62, https://doi.org/10.1007/s12040-017-0842-y, 2017. a
Kumar, P., Saharwardi, M. S., Banerjee, A., Azam, M. F., Dubey, A. K., and Murtugudde, R.: Snowfall variability dictates glacier mass balance variability in Himalaya-Karakoram, Sci. Rep., 9, 18192, https://doi.org/10.1038/s41598-019-54553-9, 2019c. a
Kumar, P., Sanwal, J., Dimri, A. P., and Ramesh, R.: Contribution of diverse monsoon precipitation over Central and Northern India during mid to Late Holocene, Quaternary Int., 507, 217–223, 2019d. a
Kumar, P. V., Naidu, C. V., and Prasanna, K.: Recent unprecedented weakening of Indian summer monsoon in warming environment, Theor. Appl. Climatol., 140, 467–486, 2020. a
Kundu, T.: Fog over Safdarjung airfield, Indian J. Meteorol. Geophys., 4, 296–302, 1957. a
Kuniyal, J. C.: Aerosols and temperature rise in the northwestern Himalaya, India, in: Dynamics of Climate Change and Water Resources of Northwestern Himalaya, edited by: Joshi, R., Kumar, K., and Palni, L., Society of Earth Scientists Seriesm Springer, Chamm https://doi.org/10.1007/978-3-319-13743-8_9, 2015. a
Kushnir, Y., Robinson, W. A., Chang, P., and Robertson, A. W.: The physical basis for predicting Atlantic sector seasonal-to-interannual climate variability, J. Climate, 19, 5949–5970, 2006. a
Kutty, S. G., Dimri, A. P., and Gultepe, I.: Climatic trends in fog occurrence over the Indo-Gangetic plains, Int. J. Climatol., 40, 2048–2061, 2020. a
Kutty, S. G., Dimri, A. P., and Gultepe, I.: Physical Processes Affecting Radiation Fog Based on WRF Simulations and Validation, Pure Appl. Geophys., 178, 4265–4288, 2021. a
Laskar, S. I., Kotal, S. D., Bhan, S. C., and Kannan, B. A. M.: Diagnosis and Numerical Simulation of Western Disturbance and Associated Unusual Rainfall over India during the Month of March, 2015, Int. J. Earth Atoms. Sci., 2, 126–148, 2015. a, b
Lee, J.-Y., Wang, B., Kang, I.-S., Shukla, J., Kumar, A., Kug, J.-S., Schemm, J. K. E., Luo, J.-J., Yamagata, T., Fu, X., Alves, O., Stern, B., Rosati, T., and Park, C.-K.: How are seasonal prediction skills related to models’ performance on mean state and annual cycle?, Clim. Dynam., 35, 267–283, 2010. a
Lee, S. and Kim, H.-k.: The dynamical relationship between subtropical and eddy-driven jets, J. Atmos. Sci., 60, 1490–1503, 2003. a
Leipe, C., Demske, D., Tarasov, P. E., and Members, H. P.: A Holocene pollen record from the northwestern Himalayan lake Tso Moriri: implications for palaeoclimatic and archaeological research, Quaternary Int., 348, 93–112, 2014. a, b
Li, B., Chen, Y., and Shi, X.: Does elevation dependent warming exist in high mountain Asia?, Environ. Res. Lett., 15, 024012, https://doi.org/10.1088/1748-9326/ab6d7f, 2020. a
Li, J., Sun, M., Yao, X., Duan, H., Zhang, C., Wang, S., Niu, S., and Yan, X.: A Review of Karakoram Glacier Anomalies in High Mountains Asia, Water, 15, 3215, https://doi.org/10.3390/w15183215, 2023. a, b
Li, X., Chen, Y. D., and Zhou, W.: Response of winter moisture circulation to the India–Burma trough and its modulation by the South Asian waveguide, J. Climate, 30, 1197–1210, 2017. a
Lone, A., Jeelani, G., Deshpande, R. D., and Padhya, V.: Impact of Indian summer monsoon in westerly dominated water resources of western Himalayas, Isot. Environ. Health S., 58, 18–43, 2022a. a
Lone, A., Jeelani, G., Lone, S. A., Padhya, V., Deshpande, R. D., and Dimri, A. P.: Spatial and meteorological controls of stable water isotope dynamics of precipitation in Kashmir Valley, Western Himalaya, India, Isot. Environ. Health S., 59, 454–475, 2023. a
Lone, A. M., Achyuthan, H., Chakraborty, S., Metya, A., Datye, A., Kripalani, R. H., and Fousiya, A. A.: Controls on the isotopic composition of daily precipitation characterized by dual moisture transport pathways at the monsoonal margin region of North-Western India, J. Hydrol., 588, 125106, https://doi.org/10.1016/j.jhydrol.2020.125106, 2020. a, b
Lone, A. M., Singh, S. P., Shah, R. A., Achyuthan, H., Ahmad, N., Qasim, A., Tripathy, G. R., Samanta, A., and Kumar, P.: The late Holocene hydroclimate variability in the Northwest Himalaya: Sedimentary clues from the Wular Lake, Kashmir Valley, J. Asian Earth Sci., 229, 105184, https://doi.org/10.1016/j.jseaes.2022.105184, 2022b. a, b
Lone, S. A., Jeelani, G., Deshpande, R. D., and Mukherjee, A.: Stable isotope (δ18O and δD) dynamics of precipitation in a high altitude Himalayan cold desert and its surroundings in Indus river basin, Ladakh, Atmos. Res., 221, 46–57, 2019. a
Lückge, A., Doose-Rolinski, H., Khan, A. A., Schulz, H., and Von Rad, U.: Monsoonal variability in the northeastern Arabian Sea during the past 5000 years: geochemical evidence from laminated sediments, Palaeogeogr. Palaeocl., 167, 273–286, 2001. a
Lyngwa, R. V., Hassan, W. U., Nayak, M. A., and Azam, M. F.: Large fraction of winter precipitation variability in two major Himalayan basins explained by Atmospheric Rivers, J. Climate, 36, 8139–8153, 2023. a
Madhura, R. K., Krishnan, R., Revadekar, J. V., Mujumdar, M., and Goswami, B. N.: Changes in western disturbances over the Western Himalayas in a warming environment, Clim. Dynam., 44, 1157–1168, 2015. a, b, c, d
Madonna, E., Wernli, H., Joos, H., and Martius, O.: Warm conveyor belts in the ERA-Interim dataset (1979–2010). Part I: Climatology and potential vorticity evolution, J. Climate, 27, 3–26, 2014. a
Mahto, S. S. and Mishra, V.: Dominance of summer monsoon flash droughts in India, Environ. Res. Lett., 15, 104061, https://doi.org/10.1088/1748-9326/abaf1d, 2020. a
Majid, S. I., Kumar, M., Kumar, P., and Verma, N. K.: GIS-Based Flood Susceptibility Mapping of Srinagar District, India Using Weights-of-Evidence (WofE), Frequency Ratio (FR) and Fuzzy Gamma Operator (FGO), J. Indian Soc. Remot., 51, 2421–2446, 2023. a
Malik, R. A., Reshi, Z. A., Rafiq, I., and Singh, S. P.: Decline in the suitable habitat of dominant Abies species in response to climate change in the Hindu Kush Himalayan region: insights from species distribution modelling, Environ. Monitor. Assess., 194, 596, https://doi.org/10.1007/s10661-022-10245-y, 2022. a
Mandal, A., Angchuk, T., Azam, M. F., Ramanathan, A., Wagnon, P., Soheb, M., and Singh, C.: An 11-year record of wintertime snow-surface energy balance and sublimation at 4863 m a.s.l. on the Chhota Shigri Glacier moraine (western Himalaya, India), The Cryosphere, 16, 3775–3799, https://doi.org/10.5194/tc-16-3775-2022, 2022. a
Mannan, M. A., Chowdhury, M. A. M., Karmakar, S., Ahmed, S., and Rahman, A.: Analysis and prediction of rainfall associated with Western Disturbances during winter months in Bangladesh, Den-Drop (A Scientific Journal of Meteorology and Geo-physics), Bangladesh Meteorological Department, Dhaka, Bangladesh, 4, 12–24, 2017. a
Marcott, S. A., Shakun, J. D., Clark, P. U., and Mix, A. C.: A reconstruction of regional and global temperature for the past 11,300 years, Science, 339, 1198–1201, 2013. a
Mariotti, A.: How ENSO impacts precipitation in southwest central Asia, Geophys. Res. Lett., 34, L16706, https://doi.org/10.1029/2007GL030078, 2007. a
Matthews, T., Perry, L. B., Aryal, D., Elmore, A. C., Khadka, A., Pelto, M., Shrestha, D., and Wilby, R.: Weather on K2 during historic first winter ascent, Weather, 77, 49–52, 2022. a
Maurya, A. S., Rai, S. P., Joshi, N., Dutt, K. S., and Rai, N.: Snowmelt runoff and groundwater discharge in Himalayan rivers: a case study of the Satluj River, NW India, Environ. Earth Sci., 77, 1–14, 2018. a, b
McClung, D. M.: Avalanche character and fatalities in the high mountains of Asia, Ann. Glaciol., 57, 114–118, 2016. a
Meher, J. K. and Das, L.: Is the Western Himalayan region vulnerable with respect to downscaled precipitation?, Theor. Appl. Climatol., 149, 233–252, 2022. a, b
Meher, J. K. and Das, L.: Probabilistic evaluation of three generations of climate models for simulating precipitation over the Western Himalayas, J. Earth Syst. Sci., 133, 15, https://doi.org/10.1007/s12040-023-02216-9, 2024. a
Mehta, M., Kumar, V., Garg, S., and Shukla, A.: Little Ice Age glacier extent and temporal changes in annual mass balance (2016–2019) of Pensilungpa Glacier, Zanskar Himalaya, Reg. Environ. Change, 21, 1–18, 2021. a, b
Midhuna, T. and Dimri, A.: Impact of Arctic Oscillation on Indian winter monsoon, Meteorol. Atmos. Phys., 131, 1157–1167, 2019. a, b, c
Midhuna, T. M. and Dimri, A. P.: Future projection of winter precipitation over northwest India and associated regions using CORDEX-SA experiments, Theor. Appl. Climatol., 139, 1317–1331, 2020. a
Midhuna, T. M. and Dimri, A. P.: Future changes in sub-seasonal variability of Indian winter precipitation, Int. J. Climatol., 42, 2384–2397, 2022. a, b
Midhuna, T. M. and Pattanaik, D. R.: The dependence of Indian winter precipitation extreme on the North Atlantic Oscillation, Natural Hazards, 117, 1869–1885, 2023a. a
Midhuna, T. M. and Pattanaik, D. R.: Predictive skill of extended range forecast of 2020–21 winter precipitation over North India, Meteorol. Atmos. Phys., 135, 26, https://doi.org/10.1007/s00703-023-00965-2, 2023b. a
Midhuna, T. M., Kumar, P., and Dimri, A. P.: A new Western Disturbance Index for the Indian winter monsoon, J. Earth Syst. Sci., 129, 59, 2020. a, b, c, d, e, f, g
Midhuna, T. M., Dimri, A. P., Suneeth, K. V., and Pattanaik, D. R.: Future frequency and intensity of Western Disturbance(s), Int. J. Climatol., 43, 1756–1772, 2023. a
Midya, S., Pal, S., Dutta, R., Gole, P., Chattopadhyay, G., Karmakar, S., Saha, U., and Hazra, S.: A preliminary study on pre-monsoon summer thunderstorms using ground-based total lightning data over Gangetic West Bengal, Indian J. Phys., 95, 1–9, 2021. a
Miles, E., McCarthy, M., Dehecq, A., Kneib, M., Fugger, S., and Pellicciotti, F.: Health and sustainability of glaciers in High Mountain Asia, Nat. Commun., 12, 2868, https://doi.org/10.1038/s41467-021-23073-4, 2021. a
Mishra, P. K., Anoop, A., Schettler, G., Prasad, S., Jehangir, A., Menzel, P., Naumann, R., Yousuf, A., Basavaiah, N., Deenadayalan, K., Wiesner, M. G., and Gaye, B.: Reconstructed late Quaternary hydrological changes from Lake Tso Moriri, NW Himalaya, Quaternary Int., 371, 76–86, 2015. a
Mishra, P. K., Prasad, S., Marwan, N., Anoop, A., Krishnan, R., Gaye, B., Basavaiah, N., Stebich, M., Menzel, P., and Riedel, N.: Contrasting pattern of hydrological changes during the past two millennia from central and northern India: Regional climate difference or anthropogenic impact?, Global Planet. Change, 161, 97–107, 2018. a
Mishra, S. K., Veselka, T. D., Prusevich, A. A., Grogan, D. S., Lammers, R. B., Rounce, D. R., Ali, S. H., and Christian, M. H.: Differential impact of climate change on the hydropower economics of two river basins in high mountain Asia, Front. Environ. Sci., 8, 26, https://doi.org/10.3389/fenvs.2020.00026, 2020. a
Mohanty, U. C., Madan, O. P., Rao, P. L. S., and Raju, P. V. S.: Meteorological fields associated with western disturbances in relation to glacier basins of western Himalayas during winter season, Tech. rep., Center for Atmospheric Sciences, Indian Institute of Technology, New Delhi, 1998. a, b, c
Mohanty, U. C., Madan, O. P., Raju, P. V. S., Bhatla, R., and Rao, P. L. S.: A study on certain dynamic and thermodynamic aspects associated with Western Disturbances over north-west Himalaya, in: The Himalayan Environment, pp. 113–122, New Age International Pvt. Ltd., 1999. a
Mooley, D. A.: The role of western disturbances in the production of weather over India during different seasons, Indian J. Meteorol. Geophys., 8, 253–260, 1957. a, b
Mukherji, A., Sinisalo, A., Nüsser, M., Garrard, R., and Eriksson, M.: Contributions of the cryosphere to mountain communities in the Hindu Kush Himalaya: a review, Reg. Environ. Change, 19, 1311–1326, 2019. a
Mukhopadhyay, B. and Khan, A.: Rising river flows and glacial mass balance in central Karakoram, J. Hydrol., 513, 192–203, 2014. a
Mull, S. and Desai, B.: The origin and structure of the winter depression of Northwest India, Tech. Rep. 25, India Meteorological Department, 1947. a, b, c
Munz, P. M., Lückge, A., Siccha, M., Böll, A., Forke, S., Kucera, M., and Schulz, H.: The Indian winter monsoon and its response to external forcing over the last two and a half centuries, Clim. Dynam., 49, 1801–1812, 2017. a, b, c
Murugavel, P., Prabha, T. V., Pandithurai, G., Gopalakrishnan, V., and D Pawar, S.: Physical mechanisms associated with the intense lightning over Indian region, Int. J. Climatol., 2021. a
Nabi, S., Ahanger, M. A., and Dar, A. Q.: Spatio-temporal variability of temperature and precipitation in a Himalayan watershed, Nat. Hazards, 115, 1743–1760, 2023. a
Nag, D., Phartiyal, B., Agrawal, S., Kumar, P., Sharma, R., Kumar, K., Sharma, A., and Joshi, M.: Westerly-monsoon variations since the last deglaciation from semi-arid Ladakh region, Trans Himalaya, India, Palaeogeogr. Palaeocl., 618, 111515, https://doi.org/10.1016/j.palaeo.2023.111515, 2023. a, b
Nageswararao, M. M., Mohanty, U. C., Ramakrishna, S. S. V. S., Nair, A., and Prasad, S. K.: Characteristics of winter precipitation over Northwest India using high-resolution gridded dataset (1901–2013), Global Planet. Change, 147, 67–85, 2016. a, b, c
Nageswararao, M. M., Dhekale, B. S., and Mohanty, U. C.: Impact of climate variability on various Rabi crops over Northwest India, Theor. Appl. Climatol., 131, 503–521, 2018a. a, b
Nageswararao, M. M., Mohanty, U. C., Ramakrishna, S. S. V. S., and Dimri, A. P.: An intercomparison of observational precipitation data sets over Northwest India during winter, Theor. Appl. Climatol., 132, 181–207, 2018b. a
Narasimha Rao, N., Devi, U., Shekhar, M. S., and Singh, G. P.: Trends of winter precipitation extremes over Northwest Himalaya, Hydrol. Sci. J., 66, 1882–1891, 2021a. a
Narasimha Rao, N., Shekhar, M. S., and Singh, G.: Performance of 4D-Var Data Assimilation on Extreme Snowfall Forecasts over the Western Himalaya Using WRF Model, Asia-Pac. J. Atmos. Sci., 57, 555–571, 2021b. a
Narayanan, P., Basistha, A., and Sachdeva, K.: Understanding trends and shifts in rainfall in parts of northwestern India based on global climatic indices, Weather, 71, 198–203, 2016. a
Nash, D., Carvalho, L. M., Rutz, J. J., and Jones, C.: Influence of the freezing level on atmospheric rivers in High Mountain Asia: WRF case studies of orographic precipitation extremes, Clim. Dynam., 62, 589–607, 2024. a
Natali, S., Doveri, M., Giannecchini, R., Baneschi, I., and Zanchetta, G.: Is the deuterium excess in precipitation a reliable tracer of moisture sources and water resources fate in the western Mediterranean? New insights from Apuan Alps (Italy), J. Hydrol., 614, 128497, https://doi.org/10.1016/j.jhydrol.2022.128497, 2022. a
Neal, R., Robbins, J., Dankers, R., Mitra, A., Jayakumar, A., Rajagopal, E. N., and Adamson, G.: Deriving optimal weather pattern definitions for the representation of precipitation variability over India, Int. J. Climatol., 40, 342–360, 2020. a, b, c, d
Negi, H. S., Kumar, A., Kanda, N., Thakur, N., and Singh, K.: Status of glaciers and climate change of East Karakoram in early twenty-first century, Sci. Total Environ., 753, 141914, https://doi.org/10.1016/j.scitotenv.2020.141914, 2021. a
Newton, A., Thunell, R., and Stott, L.: Changes in the Indonesian Throughflow during the past 2000 yr, Geology, 39, 63–66, 2011. a
Nischal, Rohtash, K, S., Pathaikara, A., Punde, P., and Attada, R.: Hydrological Extremes in Western Himalayas-Trends and Their Physical Factors, in: Natural Hazards – New Insights, edited by: Mokhtari, D. M., chap. 20, IntechOpen, Rijeka, https://doi.org/10.5772/intechopen.109445, 2023. a
Nischal, S., Attada, R., and Hunt, K. M. R.: Evaluating winter precipitation over the western Himalayas in a high-resolution Indian regional reanalysis using multisource climate datasets, J. Appl. Meteorol. Climatol., 61, 1613–1633, 2022. a, b, c, d, e, f
Norris, J., Carvalho, L. M. V., Jones, C., and Cannon, F.: WRF simulations of two extreme snowfall events associated with contrasting extratropical cyclones over the western and central Himalaya, J. Geophys. Res.-Atmos., 120, 3114–3138, 2015. a
Norris, J., Carvalho, L. M. V., Jones, C., Cannon, F., Bookhagen, B., Palazzi, E., and Tahir, A. A.: The spatiotemporal variability of precipitation over the Himalaya: evaluation of one-year WRF model simulation, Clim. Dynam., 49, 2179–2204, 2017. a
Norris, J., Carvalho, L. M., Jones, C., and Cannon, F.: Deciphering the contrasting climatic trends between the central Himalaya and Karakoram with 36 years of WRF simulations, Clim. Dynam., 52, 159–180, 2019. a
O'Brien, S. R., Mayewski, P. A., Meeker, L. D., Meese, D. A., Twickler, M. S., and Whitlow, S. I.: Complexity of Holocene climate as reconstructed from a Greenland ice core, Science, 270, 1962–1964, 1995. a
Olsen, J., Anderson, N. J., and Knudsen, M. F.: Variability of the North Atlantic Oscillation over the past 5,200 years, Nat. Geosci., 5, 808–812, 2012. a
Omesh, B., Jitendra, P., and Chaudhary, L. B.: Consequences of western disturbance-triggered cooling on the flowering of tree species in the Himalayan Terai region., Current Sci., 109, 1781–1782, 2015. a
Orr, A., Ahmad, B., Alam, U., Appadurai, A., Bharucha, Z. P., Biemans, H., Bolch, T., Chaulagain, N. P., Dhaubanjar, S., Dimri, A. P., Dixon, H., Fowler, H. J., Gioli, G., Halvorson, S. J., Hussain, A., Jeelani, G., Kamal, S., Khalid, I. S., Liu, S., Lutz, A., Mehra, M. K., Miles, E., Momblanch, A., Muccione, V., Mukherji, A., Mustafa, D., Najmuddin, O., Nasimi, M. N., Nüsser, M., Pandey, V. P., Parveen, S., Pellicciotti, F., Pollino, C., Potter, E., Qazizada, M. R., Ray, S., Romshoo, S., Sarkar, S. K., Sawas, A., Sen, S., Shah, A., Shah, M. A. A., Shea, J. M.,Sheikh, A. T., Shrestha, A. B., Tayal, S., Tigala, S., Virk, Z. T., Wester, P., and Wescoat Jr., J. L.: Knowledge priorities on climate change and water in the Upper Indus Basin: A horizon scanning exercise to identify the top 100 research questions in social and natural sciences, Earth's Future, 10, e2021EF002619, https://doi.org/10.1029/2021EF002619, 2022. a
Oza, H., Padhya, V., Ganguly, A., and Deshpande, R. D.: Investigating hydrometeorology of the Western Himalayas: Insights from stable isotopes of water and meteorological parameters, Atmos. Res., 268, 105997, https://doi.org/10.1016/j.atmosres.2021.105997, 2022. a
Pal, I., Robertson, A. W., Lall, U., and Cane, M. A.: Modeling winter rainfall in Northwest India using a hidden Markov model: understanding occurrence of different states and their dynamical connections, Clim. Dynam., 44, 1003–1015, 2015. a, b
Pal, J., Chaudhuri, S., Roychowdhury, A., and Basu, D.: An investigation of the influence of the southern annular mode on Indian summer monsoon rainfall, Meteorol, Appl,, 24, 172–179, 2017. a
Palazzi, E., Hardenberg, J., and Provenzale, A.: Precipitation in the Hindu-Kush Karakoram Himalaya: Observations and future scenarios, J. Geophys. Res.-Atmos., 118, 85–100, 2013. a
Palazzi, E., Ahmad, T. A., Cristofanelli, P., Vuillermoz, E., and Provenzale, A.: Climatic Characterization of Baltoro Glacier (Karakoram) and Northern Pakistan from In-situ Stations, in: Engineering Geology for Society and Territory - Volume 1, edited by: Lollino, G., Manconi, A., Clague, J., Shan, W., and Chiarle, M., Springer, Cham, https://doi.org/10.1007/978-3-319-09300-0_7, 2015a. a
Palazzi, E., von Hardenberg, J., Terzago, S., and Provenzale, A.: Precipitation in the Karakoram-Himalaya: a CMIP5 view, Clim. Dynam., 45, 21–45, 2015b. a, b, c
Palazzi, E., Filippi, L., and von Hardenberg, J.: Insights into elevation-dependent warming in the Tibetan Plateau-Himalayas from CMIP5 model simulations, Clim. Dynam., 48, 3991–4008, 2017. a, b, c
Palazzi, E., Mortarini, L., Terzago, S., and Von Hardenberg, J.: Elevation-dependent warming in global climate model simulations at high spatial resolution, Clim. Dynam., 52, 2685–2702, 2019. a, b
Panday, P. K., Thibeault, J., and Frey, K. E.: Changing temperature and precipitation extremes in the Hindu Kush-Himalayan region: An analysis of CMIP3 and CMIP5 simulations and projections, Int. J. Climatol., 35, 3058–3077, 2015. a
Para, J. A., Kesarkar, A., Bhate, J., Singh, V., Panchal, A., Munsi, A., Shivamurthy, Y., Lotus, S., Ahmad, N., and Sheikh, J. A.: Large-Scale dynamics of western disturbances caused extreme precipitation on 24–27 January 2017 over Jammu and Kashmir, India, Model. Earth Syst. Environ., 6, 99–107, 2020. a
Park, H.-S., Kim, S.-J., Seo, K.-H., Stewart, A. L., Kim, S.-Y., and Son, S.-W.: The impact of Arctic sea ice loss on mid-Holocene climate, Nat. Commun., 9, 4571, https://doi.org/10.1038/s41467-018-07068-2, 2018. a
Patil, M. N., Dharmaraj, T., Waghmare, R. T., Singh, S., Pithani, P., Kulkarni, R., Dhangar, N., Siingh, D., Chinthalu, G. R., Singh, R., and Ghude, S.: Observations of carbon dioxide and turbulent fluxes during fog conditions in north India, J. Earth Syst. Sci., 129, 1–12, 2020. a, b
Patil, R. and Kumar, P. P.: WRF model sensitivity for simulating intense western disturbances over North West India, Model. Earth Syst. Environ., 2, 1–15, 2016. a
Patil, R. and Kumar, P. P.: WRF Model Sensitivity to Initial Conditions and Study of Some Important Features Associated with Intense Western Disturbances Over NW India, International Journal of Engineering Technology Science and Research, 4, 9R8P8T, https://conferenceinfo.org/49-IETE-Delhi-Conference-publishedpapers (last access: 1 January 2025), 2017. a
Pattanaik, D. R., Sahai, A. K., Mandal, R., Muralikrishna, R. P., Dey, A., Chattopadhyay, R., Joseph, S., Tiwari, A. D., and Mishra, V.: Evolution of operational extended range forecast system of IMD: Prospects of its applications in different sectors, Mausam, 70, 233–264, 2019. a
Paulot, F., Naik, V., and W. Horowitz, L.: Reduction in Near-Surface Wind Speeds With Increasing CO2 May Worsen Winter Air Quality in the Indo-Gangetic Plain, Geophys. Res. Lett., 49, e2022GL099039, https://doi.org/10.1029/2022GL099039, 2022. a, b
Payra, S. and Mohan, M.: Multirule based diagnostic approach for the fog predictions using WRF modelling tool, Adv. Meteorol., 2014, 456065, https://doi.org/10.1155/2014/456065, 2014. a
Pena-Ortiz, C., Gallego, D., Ribera, P., Ordonez, P., and Alvarez-Castro, M. D. C.: Observed trends in the global jet stream characteristics during the second half of the 20th century, J. Geophys. Res.-Atmos., 118, 2702–2713, 2013. a, b
Pepin, N., Bradley, R. S., Diaz, H. F., Baraer, M., Caceres, E. B., Forsythe, N., Fowler, H., Greenwood, G., Hashmi, M. Z., Liu, X. D., Miller, J. R., Ning, L., Ohmura, A., Palazzi, E., Rangwala, I., Schoener, W., Severskiy, I., Shahgedanova, M., Wang, M. B., Williamson, S. N., Yang, D. Q., and Grp, M. R. I. E. W.: Elevation-dependent warming in mountain regions of the world, Nat. Clim. Change, 5, 424–430, https://doi.org/10.1038/NCLIMATE2563, 2015. a
Pepin, N. C., Arnone, E., Gobiet, A., Haslinger, K., Kotlarski, S., Notarnicola, C., Palazzi, E., Seibert, P., Serafin, S., Schöner, W., Terzago, S., Thornton, J. M., Vuille, M., and Adler, C.: Climate changes and their elevational patterns in the mountains of the world, Rev. Geophys., 60, e2020RG000730, https://doi.org/10.1029/2020RG000730, 2022. a
Petrie, C. A. and Weeks, L.: The Iranian plateau and the Indus river basin, in: Climate Changes in the Holocene, edited by: Chiotis, E., 293–326, CRC Press, https://doi.org/10.1201/9781351260244, 2018. a
Pfahl, S. and Sodemann, H.: What controls deuterium excess in global precipitation?, Clim. Past, 10, 771–781, https://doi.org/10.5194/cp-10-771-2014, 2014. a
Phadtare, N. R.: Sharp decrease in summer monsoon strength 4000–3500 cal yr BP in the Central Higher Himalaya of India based on pollen evidence from alpine peat, Quaternary Res., 53, 122–129, 2000. a
Phartiyal, B. and Nag, D.: Sedimentation, tectonics and climate in Ladakh, NW Trans-Himalaya-with a special reference to Late Quaternary Period, Geosyst. Geoenviron., 1, 100031, https://doi.org/10.1016/j.geogeo.2022.100031, 2022. a, b, c
Phartiyal, B., Nag, D., and Joshi, P.: Holocene climatic record of Ladakh, Trans-Himalaya, in: Holocene Climate Change and Environment, edited by: Kumaran, N. and Damodara, P., 61–89, Elsevier, https://doi.org/10.1016/B978-0-323-90085-0.00023-1, 2022. a, b
Pisharoty, P. R. and Desai, B. N.: Western disturbances and Indian weather, Indian J. Meteorol. Geophys., 7, 333–338, 1956. a, b, c, d, e, f, g, h
Portmann, R., Sprenger, M., and Wernli, H.: The three-dimensional life cycles of potential vorticity cutoffs: a global and selected regional climatologies in ERA-Interim (1979–2018), Weather Clim. Dynam., 2, 507–534, https://doi.org/10.5194/wcd-2-507-2021, 2021. a, b
Prasad, V., Phartiyal, B., and Sharma, A.: Evidence of enhanced winter precipitation and the prevalence of a cool and dry climate during the mid to late Holocene in mainland Gujarat, India, The Holocene, 17, 889–896, 2007. a
Prathipati, V. K., Naidu, C. V., and Konatham, P.: Inconsistency in the frequency of rainfall events in the Indian summer monsoon season, Int. J. Climatol., 39, 4907–4923, 2019. a
Prein, A. F., Rasmussen, R. M., Ikeda, K., Liu, C., Clark, M. P., and Holland, G. J.: The future intensification of hourly precipitation extremes, Nat. Clim. Change, 7, 48, https://doi.org/10.1038/nclimate3168, 2017. a
Priestley, M. D. K., Pinto, J. G., Dacre, H. F., and Shaffrey, L. C.: Rossby wave breaking, the upper level jet, and serial clustering of extratropical cyclones in western Europe, Geophys. Res. Lett., 44, 514–521, 2017. a
Qin, Y., Ren, G.-Y., Wu, L.-X., and Zhang, P.-F.: The relationship between elevation-dependent warming and long-term trend of surface air temperature lapse rate, Atmos. Res., 307, 107478, https://doi.org/10.1016/j.atmosres.2024.107478, 2024. a
Qiu, T., Huang, W., Wright, J. S., Yang, Z., and Wang, B.: Impacts of Western Disturbances on Wintertime Precipitation Over the Southeastern Tibetan Plateau, J. Geophys. Res.-Atmos., 127, e2021JD035789, https://doi.org/10.1029/2021JD035789, 2022. a, b, c, d
Raghuvanshi, A. S. and Agarwal, A.: Multiscale dynamics of transient merging between western disturbances and monsoonal lows: Connections to the July 2023 flood in Himachal Pradesh, Atmos. Res., 304, 107401, https://doi.org/10.1016/j.atmosres.2024.107401, 2024. a
Rahman, G., Rahman, A.-u., Munawar, S., Moazzam, M. F. U., Dawood, M., Miandad, M., and Panezai, S.: Trend analysis of historical and future precipitation projections over a diverse topographic region of Khyber Pakhtunkhwa using SDSM, J. Water Clim. Change, 13, 3792–3811, 2022. a
Rajbhandari, R., Shrestha, A., Kulkarni, A., Patwardhan, S. K., and Bajracharya, S. R.: Projected changes in climate over the Indus river basin using a high resolution regional climate model (PRECIS), Clim. Dynam., 44, 339–357, 2015. a
Rajeevan, M., Bhate, J., Kale, J. D., and Lal, B.: Development of a high resolution daily gridded rainfall data for the Indian region, Meteorological Monographs, 22, ftp://squall.met.fsu.edu/LAU/070108_1446/doc/ref_report.pdf.pdf (last access: 1 January 2025), 2005. a
Rajeevan, M., Pai, D. S., Anil Kumar, R., and Lal, B.: New statistical models for long-range forecasting of southwest monsoon rainfall over India, Clim. Dynam., 28, 813–828, 2007. a
Raju, A., Parekh, A., Kumar, P., and Gnanaseelan, C.: Evaluation of the impact of AIRS profiles on prediction of Indian summer monsoon using WRF variational data assimilation system, J. Geophys. Res.-Atmos., 120, 8112–8131, 2015. a
Raju, P. V. S., Bhatla, R., and Mohanty, U. C.: A study on certain aspects of kinetic energy associated with western disturbances over northwest India, Atmósfera, 24, 375–384, 2011. a
Rakesh, V., Singh, R., and Joshi, P. C.: Intercomparison of the performance of MM5/WRF with and without satellite data assimilation in short-range forecast applications over the Indian region, Meteorol. Atmos. Phys., 105, 133–155, 2009a. a
Rakesh, V., Singh, R., Yuliya, D., Pal, P. K., and Joshi, P. C.: Impact of variational assimilation of MODIS thermodynamic profiles in the simulation of western disturbance, Int. J. Remote Sens., 30, 4867–4887, 2009b. a, b
Ramanathan, V. and Saha, K. R.: Application of a primitive equation barotropic model to predict movement of “Western Disturbances”, J. Appl. Meteorol., 11, 268–272, 1972. a, b
Ramasastri, K. S.: Snow melt modeling studies in India, 59–70, in: The Himalayan Environment, edited by: Dash, S. K. and Bahadur, J., New Age International, 59–70, 1999. a
Ramaswamy, C.: On the sub-tropical jet stream and its role in the development of large-scale convection, Tellus, 8, 26–60, 1956. a
Ran, M. and Chen, L.: The 4.2 ka BP climatic event and its cultural responses, Quaternary Int., 521, 158–167, 2019. a
Ranalkar, M. R., Chaudhari, H. S., Hazra, A., Sawaisarje, G. K., and Pokhrel, S.: Dynamical features of incessant heavy rainfall event of June 2013 over Uttarakhand, India, Nat. Hazards, 80, 1579–1601, 2016. a
Rangachary, N. and Bandyopadhyay, B. K.: An analysis of the synoptic weather pattern associated with extensive avalanching in Western Himalaya, Int. Assoc. of Hydrol. Sci. Publ, 162, 311–316, 1987. a
Rangwala, I., Miller, J. R., and Xu, M.: Warming in the Tibetan Plateau: possible influences of the changes in surface water vapor, Geophys. Res. Lett., 36, L06703, https://doi.org/10.1029/2009GL037245, 2009. a
Rani, S. I., Arulalan, T., George, J. P., Rajagopal, E., Renshaw, R., Maycock, A., Barker, D. M., and Rajeevan, M.: IMDAA: High-Resolution Satellite-Era Reanalysis for the Indian Monsoon Region, J. Climate, 34, 5109–5133, 2021. a
Rao, P. C., Archana, S., and Jaswal, A. K.: Intense precipitation causing floods over Himalayan region of northern India–a case study on role of atmospheric rivers, J. Ind. Geophys. Union, 20, 191–200, 2016. a, b
Rao, P. L. S.: Circulation characteristics over the Himalayas during winter season, Meteorol. Atmos. Phys., 83, 19–33, 2003. a
Rao, V. B. and Rao, S. T.: A theoretical and synoptic study of western disturbances, Pure Appl. Geophys., 90, 193–208, 1971. a, b
Rao, Y. (Ed.): Southwest monsoon, vol. 366, India Meteorological Department, New Delhi, https://imetsociety.org/wp-content/pdf/docs/swmonsoon_yprao.pdf (last access: 8 January 2025), 1976. a
Rao, Y. P. and Srinivasan, V.: Discussion of typical synoptic weather situation: winter western disturbances and their associated features, Tech. Rep. IMD Forecasting Manual Report-III 1.1, India Meteorological Department, 1969. a, b, c, d, e, f, g, h
Rashid, I., Majeed, U., Aneaus, S., Cánovas, J. A. B., Stoffel, M., Najar, N. A., Bhat, I. A., and Lotus, S.: Impacts of Erratic Snowfall on Apple Orchards in Kashmir Valley, India, Sustainability, 12, 9206, https://doi.org/10.3390/su12219206, 2020. a
Rasmussen, R., Baker, B., Kochendorfer, J., Meyers, T., Landolt, S., Fischer, A. P., Black, J., Thériault, J. M., Kucera, P., Gochis, D., Smith, C., Nitu, R., Hall, M., Ikeda, K., and Gutmann, E.: How well are we measuring snow: The NOAA/FAA/NCAR winter precipitation test bed, B. Am. Meteorol. Soc., 93, 811–829, 2012. a
Ratnam, J. V., Behera, S. K., Annamalai, H., Ratna, S. B., Rajeevan, M., and Yamagata, T.: ENSO's far reaching connection to Indian cold waves, Sci. Rep., 6, 1–12, 2016. a
Ravishankar, H., Adak, T., Singh, V., Pandey, B., Singh, A., and BR, S.: Empirical appraisal of some weather parameters’ dynamics for their possibl e implications on mango production in some important mango growing region s with special reference to Lucknow region of Uttar Pradesh, Clim. Dynam. in Horticultural Sciences, 1, 61–84, 2015. a
Rawat, S., Gupta, A. K., Sangode, S. J., Srivastava, P., and Nainwal, H. C.: Late Pleistocene–Holocene vegetation and Indian summer monsoon record from the Lahaul, northwest Himalaya, India, Quaternary Sci. Rev., 114, 167–181, 2015. a
Rayner, N. A., Parker, D. E., Horton, E. B., Folland, C. K., Alexander, L. V., Rowell, D. P., Kent, E. C., and Kaplan, A.: Global analyses of sea surface temperature, sea ice, and night marine air temperature since the late nineteenth century, J. Geophys. Res., 108, 4407, https://doi.org/10.1029/2002JD002670, 2003. a
Reinhold, B.: Orographic modulation of baroclinic instability, J. Atmos. Sci., 47, 1697–1713, 1990. a
Remya, R., Kottayil, A., and Mohanakumar, K.: Influence of sudden stratospheric warming and quasi biennial oscillation on western disturbance over north India, J. Atmos. Solar-Terr. Phy., 160, 1–10, 2017. a
Ridley, J., Wiltshire, A., and Mathison, C.: More frequent occurrence of westerly disturbances in Karakoram up to 2100, Sci. Total Environ., 468, S31–S35, 2013. a, b
Riehl, H.: Jet streams of the atmosphere, Tech. rep., Department of Atmospheric Science, Colorado State University, tech. Rep. 32, 1962. a
Riley, C., Rupper, S., Steenburgh, J. W., Strong, C., Kochanski, A. K., and Wolvin, S.: Characteristics of historical precipitation in high mountain Asia based on a 15-year high resolution dynamical downscaling, Atmosphere, 12, 355, https://doi.org/10.3390/atmos12030355, 2021. a, b
Roads, J. O.: Stable near-resonant states forced by orography in a simple baroclinic model, J. Atmos. Sci., 37, 2381–2395, 1980. a
Robbins Schug, G., Blevins, K. E., Cox, B., Gray, K., and Mushrif-Tripathy, V.: Infection, disease, and biosocial processes at the end of the Indus Civilization, PLoS One, 8, e84814, https://doi.org/10.1371/journal.pone.0084814, 2013. a
Roberts, N., Eastwood, W. J., Kuzucuoğlu, C., Fiorentino, G., and Caracuta, V.: Climatic, vegetation and cultural change in the eastern Mediterranean during the mid-Holocene environmental transition, The Holocene, 21, 147–162, 2011. a
Roberts, N. M., Cole, S. J., Forbes, R. M., Moore, R. J., and Boswell, D.: Use of high-resolution NWP rainfall and river flow forecasts for advance warning of the Carlisle flood, north-west England, Meteorol. Appl., 16, 23–34, 2009. a
Rowan, A. V.: The `Little Ice Age' in the Himalaya: A review of glacier advance driven by Northern Hemisphere temperature change, The Holocene, 27, 292–308, 2017. a
Roy, I., Tomar, N., Ranhotra, P. S., and Sanwal, J.: Proxy Response Heterogeneity to the Indian Monsoon During Last Millennium in the Himalayan Region, Front. Ecol. Evol., 10, 778825, https://doi.org/10.3389/fevo.2022.778825, 2022. a
Roy, S. C. and Chatterji, G.: Origin of Nor'westers, Nature, 124, 481–481, 1929. a
Roy, S. S.: The impacts of ENSO, PDO, and local SSTs on winter precipitation in India, Phys. Geogr., 27, 464–474, 2006. a, b
Rühland, K., Phadtare, N. R., Pant, R. K., Sangode, S. J., and Smol, J. P.: Accelerated melting of Himalayan snow and ice triggers pronounced changes in a valley peatland from northern India, Geophys. Res. Lett., 33, L15709, https://doi.org/10.1029/2006GL026704, 2006. a
Sadaf, N., Lin, Y., and Dong, W.: Wet spells over the core monsoon domain of northern Pakistan during the summer season, Int. J. Climatol., 41, 1402–1420, 2021. a
Sadler, J. C.: The upper tropospheric circulation over the global tropics, Department of Meteorology, University of Hawaii Honolulu, Hawaii, 1975. a
Safdar, F., Khokhar, M. F., Mahmood, F., Khan, M. Z. A., and Arshad, M.: Observed and predicted precipitation variability across Pakistan with special focus on winter and pre-monsoon precipitation, Environ. Sci. Pollut. Res., 30, 4510–4530, 2023. a
Saji, N. H., Goswami, B. N., Vinayachandran, P. N., and Yamagata, T.: A Dipole Mode in the tropical Indian Ocean, Nature, 401, 360—363, https://doi.org/10.1038/43854, 1999. a
Salerno, F., Guyennon, N., Yang, K., Shaw, T. E., Lin, C., Colombo, N., Romano, E., Gruber, S., Bolch, T., Alessandri, A., Cristofanelli, P., Putero, D., Diolaiuti, G., Tartari, G., Verza, G., Thakuri, S., Balsamo, G., Miles, E. S., and Pellicciotti, F.: Local cooling and drying induced by Himalayan glaciers under global warming, Nat. Geosci., 16, 1120–1127, 2023. a
Samra, J. S., Singh, G., and Ramakrishna, Y. S.: Cold wave of 2002-03 – Impact on agriculture, Natural Resource Management Division, Indian Council of Agricultural Research, 2003. a
Sanap, S. D.: Dynamical linkages between Arctic Oscillations and winter precipitation patterns over the Indian Region, Global Planet. Change, 231, 104293, https://doi.org/10.1016/j.gloplacha.2023.104293, 2023. a, b
Sandeep, A. and Prasad, V. S.: On the Variability of Cold Wave Episodes Over Northwest India Using an NGFS Retrospective Analysis, Pure Appl. Geophys., 177, 1157–1166, 2020. a
Sankar, N. V. and Babu, C. A.: Role of vorticity advection and thermal advection in the development of western disturbance during North Indian winter, Meteorol. Atmos. Phys., 132, 515–529, 2020. a, b, c
Sankar, N. V. and Babu, C. A.: Study on Environmental and Thermodynamic Factors that influence Precipitation associated with Western Disturbances over North India during Winter, Pure Appl. Geophys., 178, 4073–4096, 2021. a, b
Sankar, N. V., Babu, C. A., and Anil: Utility of INSAT 3D/3DR products in understanding the physical processes in clouds associated with western disturbance affecting North India during winter, Int. J. Remote Sens., 42, 8440–8467, 2021. a
Sanwal, J., Kotlia, B. S., Rajendran, C., Ahmad, S. M., Rajendran, K., and Sandiford, M.: Climatic variability in Central Indian Himalaya during the last 1800 years: Evidence from a high resolution speleothem record, Quaternary Int., 304, 183–192, 2013. a
Saranya, P., Krishan, G., Rao, M. S., Kumar, S., and Kumar, B.: Controls on water vapor isotopes over Roorkee, India: Impact of convective activities and depression systems, J. Hydrol., 557, 679–687, 2018. a
Sarkar, A., Dutta, D., Chakraborty, P., Dutta, S. K., Majumdar, S., Payra, S., and Bhatla, R.: Influence of cumulus convection and cloud microphysics parameterizations on the prediction of Western Disturbances, Meteorol. Atmos. Phys., 132, 413–426, 2019. a, b
Sarkar, A., Dutta, D., Chakraborty, P., Dutta, S. K., Majumdar, S., Payra, S., and Bhatla, R.: Influence of cumulus convection and cloud microphysics parameterizations on the prediction of Western Disturbances, Meteorol. Atmos. Phys., 132, 413–426, 2020. a
Satheesh Chandran, P. R., Sunilkumar, S. V., Muhsin, M., and Alladi, H. K.: Effect of Synoptic-Scale Dynamics on the Vertical Distribution of Tropospheric Ozone Over the Arabian Sea and the Indian Ocean During the Boreal Winter of 2018, J. Geophys. Res.-Atmos., 127, e2021JD036412, https://doi.org/10.1029/2021JD036412, 2022. a
Sawaisarje, G. K., Khare, P., Shirke, C. Y., Deepakumar, S., and Narkhede, N. M.: Study of winter fog over Indian subcontinent: Climatological perspectives, Mausam, 65, 19–28, 2014. a, b
Saxena, S., Singh, C., and Srivastava, K.: Meteorological observations analysis for forewarning of exceptionally heavy rainfall over Himalayan region of India: a case study over Uttarakhand during October, 2021, Nat. Hazards, 120, 2403–2413, https://doi.org/10.1007/s11069-023-06282-6, 2023. a
Schemm, S., Sprenger, M., and Wernli, H.: When during their life cycle are extratropical cyclones attended by fronts?, B. Am. Meteorol. Soc., 99, 149–165, 2018. a
Schiemann, R., Lüthi, D., and Schär, C.: Seasonality and interannual variability of the westerly jet in the Tibetan Plateau region, J. Climate, 22, 2940–2957, 2009. a, b, c, d
Scroxton, N., Burns, S. J., McGee, D., Godfrey, L. R., Ranivoharimanana, L., Faina, P., and Tiger, B. H.: Tropical Indian Ocean basin hydroclimate at the Mid-to Late-Holocene transition and the double drying hypothesis, Quaternary Sci. Rev., 300, 107837, https://doi.org/10.1016/j.quascirev.2022.107837, 2022. a, b
Sebastian, D. E., Ganguly, S., Krishnaswamy, J., Duffy, K., Nemani, R., and Ghosh, S.: Multi-scale association between vegetation growth and climate in India: a wavelet analysis approach, Remote Sens., 11, 2703, https://doi.org/10.3390/rs11222703, 2019. a
Selvi, S. and Rajapandian, S.: Analysis of lightning hazards in India, Int. J. Disast. Risk Re., 19, 22–24, 2016. a
Semwal, G. and Dimri, A. P.: Impact of initial and boundary conditions on simulations of western disturbances and associated precipitation, Nat. Hazards, 64, 1405–1424, 2012. a, b
Sen Gupta, A. and England, M. H.: Coupled ocean–atmosphere feedback in the southern annular mode, J. Climate, 20, 3677–3692, 2007. a
Sharma, P., Sen, B., Balling Jr, R. C., Roy, S. S., and Sen Roy, S.: Diurnal cycle of summer season thunderstorm activity in India, Theor. Appl. Climatol., 151, 1567–1583, https://doi.org/10.1007/s00704-022-04339-3, 2023. a
Shekhar, M., Bhardwaj, A., Singh, S., Ranhotra, P. S., Bhattacharyya, A., Pal, A. K., Roy, I., Martín-Torres, F. J., and Zorzano, M.-P.: Himalayan glaciers experienced significant mass loss during later phases of little ice age, Sci. Rep., 7, 10305, https://doi.org/10.1038/s41598-017-09212-2, 2017. a
Shekhar, M., Pal, A. K., Bhattacharyya, A., Ranhotra, P. S., and Roy, I.: Tree-ring based reconstruction of winter drought since 1767 CE from Uttarkashi, Western Himalaya, Quaternary Int., 479, 58–69, 2018. a
Shekhar, M. S., Chand, H., Kumar, S., Srinivasan, K., and Ganju, A.: Climate-change studies in the western Himalaya, Ann. Glaciol., 51, 105–112, 2010. a, b, c, d
Shrestha, S., Yao, T., and Adhikari, T. R.: Analysis of rainfall trends of two complex mountain river basins on the southern slopes of the Central Himalayas, Atmos. Res., 215, 99–115, 2019. a, b
Shukla, B. P., John, J., Padmakumari, B., Das, D., Thirugnanasambantham, D., and Gairola, R.: Did dust intrusion and lofting escalate the catastrophic widespread lightning on 16th April 2019, India?, Atmos. Res., 266, 105933, https://doi.org/10.1016/j.atmosres.2021.105933, 2022. a
Silverman, N. L., Maneta, M. P., Chen, S.-H., and Harper, J. T.: Dynamically downscaled winter precipitation over complex terrain of the Central Rockies of Western Montana, USA, Water Resour. Res., 49, 458–470, 2013. a
Singh, A. T., Rahaman, W., Sharma, P., Laluraj, C. M., Patel, L. K., Pratap, B., Gaddam, V. K., and Thamban, M.: Moisture sources for precipitation and hydrograph components of the Sutri Dhaka glacier basin, Western Himalayas, Water, 11, 2242, https://doi.org/10.3390/w11112242, 2019a. a, b
Singh, B. and Thapliyal, R.: Cloudburst events observed over Uttarakhand during monsoon season 2017 and their analysis, Mausam, 73, 91–104, 2022. a
Singh, C. and Chand, R.: Exceptionally heavy rainfall over Uttarakhand during 15-18 June, 2013-A case study, Mausam, 66, 741–750, 2015. a
Singh, D., Sharma, V., and Juyal, V.: Observed linear trend in few surface weather elements over the Northwest Himalayas (NWH) during winter season, J. Earth Syst. Sci., 124, 553–565, 2015. a
Singh, D., Kumar, A., Shekhar, M. S., and Garg, S.: The impacts of the approaching western disturbances (WDs) on the surface meteorological variables over the north-west Himalaya (NWH), India, J. Earth Syst. Sci., 128, 1–18, 2019b. a, b
Singh, G., Joshi, R. D., Chopra, S. K., and Singh, A. B.: Late Quaternary history of vegetation and climate of the Rajasthan Desert, India, Philos. T. Roy. Soc. Lond. B, 267, 467–501, 1974. a
Singh, J., Singh, V., and Ojha, C.: Elevation dependent precipitation changes in historical and future times in the Himalayan Upper Ganga Basin, India, Atmos. Res., 307, 107501, https://doi.org/10.1016/j.atmosres.2024.107501, 2024. a
Singh, M. S.: Upper air circulation associated with a Western Disturbance, Mausam, 14, 156–172, 1963. a
Singh, M. S.: The study of the jet stream over India and to its north in winter, Part-I, Indian J. Met. Geophys, 22, 1–14, 1971. a
Singh, M. S. and Agnihotri, C. L.: Baroclinity over India in winter and its relation to western disturbances and jet streams. I, Indian J. Meteorol. Hydrol. Geophys., 28, 303–310, 1977. a, b
Singh, M. S. and Kumar, S.: Study of a western disturbance, Mausam, 28, 233–242, 1977. a
Singh, M. S., Rao, A. V. R. K., and Gupta, S. C.: Development and movement of a mid-tropospheric cyclone in the westerlies over India, Mausam, 32, 45–50, 1981. a
Singh, O. and Singh, J.: Lightning fatalities over India: 1979–2011, Meteorol. Appl., 22, 770–778, 2015. a
Smith, D., Dorling, S., Renfrew, I., Ross, A., and Poku, C.: Fog trends in India: relationships to fog type and western disturbances, Int. J. Climatol., 43, 818–836, 2022. a, b, c, d, e
Smith, R. B.: The influence of mountains on the atmosphere, in: Advances in geophysics, edited by: Saltzman, B., 21, 87–230, Elsevier, https://doi.org/10.1016/S0065-2687(08)60262-9, 1979. a
Spate, M., Penny, D., Yatoo, M., and Betts, A.: Re-examining climate-driven Malthusian collapse in Kashmir: New palaeoenvironmental context for the archaeological record, Quaternary Int., 623, 127–138, 2022. a
Sperber, K. R. and Annamalai, H.: The use of fractional accumulated precipitation for the evaluation of the annual cycle of monsoons, Clim. Dynam., 43, 3219–3244, 2014. a
Sperber, K. R., Annamalai, H., Kang, I.-S., Kitoh, A., Moise, A., Turner, A. G., Wang, B., and Zhou, T.: The Asian summer monsoon: an intercomparison of CMIP5 vs. CMIP3 simulations of the late 20th century, Clim. Dynam., 41, 2711–2744, https://doi.org/10.1007/s00382-012-1607-6, 2013. a
Sreeparvathy, V. and Srinivas, V. V.: Global assessment of spatiotemporal variability of wet, normal and dry conditions using multiscale entropy-based approach, Sci. Rep., 12, 1–18, 2022. a
Srinivasan, K., Ganju, A., and Sharma, S. S.: Usefulness of mesoscale weather forecast for avalanche forecasting, Current Sci, 88, 921–926, 2005. a
Srivastava, S. K., Sharma, A. R., and Sachdeva, K.: A ground observation based climatology of winter fog: study over the Indo-Gangetic Plains, India, International Journal of Environmental and Ecological Engineering, 10, 742–753, 2016. a, b
Staubwasser, M., Sirocko, F., Grootes, P. M., and Segl, M.: Climate change at the 4.2 ka BP termination of the Indus valley civilization and Holocene south Asian monsoon variability, Geophys. Res. Lett., 30, 1425, https://doi.org/10.1029/2002GL016822, 2003. a, b
Stohl, A. and James, P.: A Lagrangian analysis of the atmospheric branch of the global water cycle. Part I: Method description, validation, and demonstration for the August 2002 flooding in central Europe, J. Hydrometeorol., 5, 656–678, 2004. a
Stohl, A. and James, P.: A Lagrangian analysis of the atmospheric branch of the global water cycle. Part II: Moisture transports between Earth's ocean basins and river catchments, J. Hydrometeorol., 6, 961–984, 2005. a
Strangeways, I. (Ed.): Precipitation: theory, measurement and distribution, Cambridge University Press, ISBN 9781139460019, 2006. a
Strangeways, I.: A history of rain gauges., Weather, 65, 133–138, 2010. a, b
Su, B., Huang, J., Gemmer, M., Jian, D., Tao, H., Jiang, T., and Zhao, C.: Statistical downscaling of CMIP5 multi-model ensemble for projected changes of climate in the Indus River Basin, Atmos. Res., 178, 138–149, 2016. a
Su, F.-G., Duan, X.-L., Chen, D.-L., Hao, Z.-C., and Cuo, L.: Evaluation of the global climate models in the CMIP5 over the Tibetan Plateau, J. Climate, 26, 3187–3208, 2013. a
Subbaramayya, I. and Raju, A. S. N.: A study of tropospheric wave disturbances over India in winter, Pure Appl. Geophys., 120, 437–452, 1982. a, b, c
Sundriyal, S., Shukla, T., Tripathee, L., Dobhal, D. P., Tiwari, S. K., and Bhan, U.: Deposition of atmospheric pollutant and their chemical characterization in snow pit profile at Dokriani Glacier, Central Himalaya, J. Mount. Sci., 15, 2236–2246, 2018. a, b
Sunil, S. and Padmakumari, B.: High temporal variability of wintertime fog, aerosol and Red-Blue-Ratio using ground-based observations of shortwave irradiance and sky imagery over a station in Indo-Gangetic Basin: Optical and Radiative characteristics, Atmos. Environ., 226, 117382, https://doi.org/10.1016/j.atmosenv.2020.117382, 2020. a, b
Syed, F. S., Giorgi, F., Pal, J. S., and King, M. P.: Effect of remote forcings on the winter precipitation of central southwest Asia part 1: observations, Theor. Appl. Climatol., 86, 147–160, 2006. a, b, c
Syed, F. S., Giorgi, F., Pal, J., and Keay, K.: Regional climate model simulation of winter climate over Central–Southwest Asia, with emphasis on NAO and ENSO effects, Int. J. Climatol., 30, 220–235, 2010. a
Syed, F. S., Körnich, H., and Tjernström, M.: On the fog variability over south Asia, Clim. Dynam., 39, 2993–3005, 2012. a
Szymczak, R. K., Marosz, M., Grzywacz, T., Sawicka, M., and Naczyk, M.: Death zone weather extremes mountaineers have experienced in successful ascents, Front. Physiol., 12, 696335, https://doi.org/10.3389/fphys.2021.696335, 2021. a
Terzago, S., von Hardenberg, J., Palazzi, E., and Provenzale, A.: Snowpack changes in the Hindu Kush–Karakoram–Himalaya from CMIP5 global climate models, J. Hydrometeorol., 15, 2293–2313, 2014. a
Thapa, K., Endreny, T. A., and Ferguson, C. R.: Atmospheric rivers carry nonmonsoon extreme precipitation into Nepal, J. Geophys. Res.-Atmos., 123, 5901–5912, 2018. a
Thapa, U. K., Stevenson, S., and Midhun, M.: Orbital Forcing Strongly Influences the Poleward Shift of the Spring Himalayan Jet During the Past Millennium, Geophys. Res. Lett., 49, e2021GL095955, https://doi.org/10.1029/2021GL095955, 2022. a
Thapliyal, R. and Singh, B.: A case study of exceptionally heavy rainfall event over Uttarakhand, India on 18th October, 2021 and its forecasting, Mausam, 74, 717–740, 2023a. a, b, c, d
Thapliyal, R. and Singh, B.: Heavy rainfall forecasting for Dehradun capital city during monsoon season 2020, Mausam, 74, 141–150, 2023b. a
Thayyen, R. J. and Gergan, J. T.: Role of glaciers in watershed hydrology: a preliminary study of a “Himalayan catchment”, The Cryosphere, 4, 115–128, https://doi.org/10.5194/tc-4-115-2010, 2010. a, b
Thayyen, R. J., Dimri, A. P., Kumar, P., and Agnihotri, G.: Study of cloudburst and flash floods around Leh, India, during August 4–6, 2010, Nat. Hazards, 65, 2175–2204, 2013. a
Thomas, B., Kunchala, R. K., Singh, B. B., and Kumar, K. N.: Climatology of Rossby wave breaking over the subtropical Indian region, J. Geophys. Res.-Atmos., 128, e2022JD038344, https://doi.org/10.1029/2022JD038344, 2023. a
Thomas, L., Dash, S. K., and Mohanty, U. C.: Influence of various land surface parameterization schemes on the simulation of Western Disturbances, Meteorol. Appl., 21, 635–643, 2014. a, b
Thomas, L., Dash, S. K., Mohanty, U. C., and Babu, C. A.: Features of western disturbances simulated over north India using different land-use data sets, Meteorol. Appl., 25, 246–253, 2018. a
Thurnherr, I., Kozachek, A., Graf, P., Weng, Y., Bolshiyanov, D., Landwehr, S., Pfahl, S., Schmale, J., Sodemann, H., Steen-Larsen, H. C., Toffoli, A., Wernli, H., and Aemisegger, F.: Meridional and vertical variations of the water vapour isotopic composition in the marine boundary layer over the Atlantic and Southern Ocean, Atmos. Chem. Phys., 20, 5811–5835, https://doi.org/10.5194/acp-20-5811-2020, 2020. a
Tian, Y., Peters-Lidard, C. D., Eylander, J. B., Joyce, R. J., Huffman, G. J., Adler, R. F., Hsu, K.-l., Turk, F. J., Garcia, M., and Zeng, J.: Component analysis of errors in satellite-based precipitation estimates, J. Geophys. Res.-Atmos., 114, D24101, https://doi.org/10.1029/2009JD011949, 2009. a
Torrence, C. and Webster, P. J.: Interdecadal changes in the ENSO–monsoon system, J. Climate, 12, 2679–2690, 1999. a
Tory, K. J. and Frank, W. M.: Tropical cyclone formation, edited by: Chan, J. C. L. and Kepert, J. D., 55–91, World Scientific, https://doi.org/10.1142/9789814293488_0002, 2010. a
Trivedi, A. and Chauhan, M. S.: Pollen proxy records of Holocene vegetation and climate change from Mansar Lake, Jammu region, India, Current Sci., 95, 1347–1354, 2008. a
Turner, A. G., Inness, P. M., and Slingo, J. M.: The role of the basic state in the ENSO-monsoon relationship and implications for predictability, Q. J. Roy. Meteor. Soc., 131, 781–804, https://doi.org/10.1256/qj.04.70, 2005. a
Ullah, I., Ma, X., Yin, J., Saleem, F., Syed, S., Omer, A., Habtemicheal, B. A., Liu, M., and Arshad, M.: Observed changes in seasonal drought characteristics and their possible potential drivers over Pakistan, Int. J. Climatol., 42, 1576–1596, 2022. a, b
Unnikrishnan, C. K., Pawar, S., and Gopalakrishnan, V.: Satellite-observed lightning hotspots in India and lightning variability over tropical South India, Adv. Space Res., 68, 1690–1705, 2021. a, b
Usman, M., Liedl, R., Zhang, F., and Zaman, M.: Groundwater irrigated agriculture evolution in central Punjab, Pakistan, edited by: Lichtfouse, E., Sustainable Agriculture Reviews 33: Climate Impact on Agriculture, 259–294, vol. 33, Springer, Cham, https://doi.org/10.1007/978-3-319-99076-7_9, 2018. a
Valdiya, K. S.: Anomalous weather events in central Himalaya: real-world observations and some questions, Current Sci., 119, 19–25, 2020. a, b, c, d, e, f
van Hengstum, P. J., Donnelly, J. P., Fall, P. L., Toomey, M. R., Albury, N. A., and Kakuk, B.: The intertropical convergence zone modulates intense hurricane strikes on the western North Atlantic margin, Sci. Rep., 6, 1–10, 2016. a
Veeraraghavan, K. and Nath, T.: A satellite study of an active Western Disturbance, Mausam, 40, 303–306, 1989. a, b
Vellore, R. K., Kaplan, M. L., Krishnan, R., Lewis, J. M., Sabade, S., Deshpande, N., Singh, B. B., Madhura, R. K., and Rao, M. V. S. R.: Monsoon-extratropical circulation interactions in Himalayan extreme rainfall, Clim. Dynam., 46, 3517–3546, 2016. a, b, c
Verma, S., Ramana, M. V., and Kumar, R.: Atmospheric rivers fueling the intensification of fog and haze over Indo-Gangetic Plains, Sci. Rep., 12, 5139, https://doi.org/10.1038/s41598-022-09206-9, 2022. a, b
Victor, J. N., Afreen, S., Siingh, D., Chandra, S., Singh, R. P., Potdar, S. S., Bashir, G., Kazhugasalamoorthy, S., Nirmal Kumar, R., Singh, V., and Ahmad, N.: Electrical Characteristics of Thunderstorms Under Different Weather Conditions in the Kashmir Valley, India, Pure Appl. Geophys., 180, 1185–1204, 2023. a
Vinayachandran, P. N., Francis, P. A., and Rao, S. A.: Indian Ocean dipole: processes and impacts, Current trends in science, 46, 569–589, 2009. a
Wang, T., Yang, S., Wen, Z., Wu, R., and Zhao, P.: Variations of the winter India-Burma Trough and their links to climate anomalies over southern and eastern Asia, J. Geophys. Res.-Atmos., 116, D23118, https://doi.org/10.1029/2011JD016373, 2011. a
Wang, Y. and Chen, Y.: Significant climate impact of highly hygroscopic atmospheric aerosols in Delhi, India, Geophys. Res. Lett., 46, 5535–5545, 2019. a
Wanner, H., Beer, J., Bütikofer, J., Crowley, T. J., Cubasch, U., Flückiger, J., Goosse, H., Grosjean, M., Joos, F., Kaplan, J. O., Küttel, M., Müller, S. A., Prentice, I. C., Solomina, O., Stocker, T. F., Tarasov, P., Wagner, M., and Widmann, M.: Mid-to Late Holocene climate change: an overview, Quaternary Sci. Rev., 27, 1791–1828, 2008. a
Waqas, A. and Athar, H.: Spatiotemporal variability in daily observed precipitation and its relationship with snow cover of Hindukush, Karakoram and Himalaya region in northern Pakistan, Atmos. Res., 228, 196–205, 2019. a
Wernli, H. and Schwierz, C.: Surface cyclones in the ERA-40 dataset (1958–2001). Part I: Novel identification method and global climatology, J. Atmos. Sci., 63, 2486–2507, 2006. a
Wernli, H. and Sprenger, M.: Identification and ERA-15 climatology of potential vorticity streamers and cutoffs near the extratropical tropopause, J. Atmos. Sci., 64, 1569–1586, 2007. a
Winiger, M. G. H. Y., Gumpert, M., and Yamout, H.: Karakorum–Hindukush–western Himalaya: assessing high-altitude water resources, Hydrol. Process., 19, 2329–2338, 2005. a
Wirth, S. B., Glur, L., Gilli, A., and Anselmetti, F. S.: Holocene flood frequency across the Central Alps–solar forcing and evidence for variations in North Atlantic atmospheric circulation, Quaternary Sci. Rev., 80, 112–128, 2013. a
Wu, T.-W. and Qian, Z.-A.: The relation between the Tibetan winter snow and the Asian summer monsoon and rainfall: An observational investigation, J. Climate, 16, 2038–2051, 2003. a
Xavier, P. K., Marzin, C., and Goswami, B. N.: An objective definition of the Indian summer monsoon season and a new perspective on the ENSO–monsoon relationship, Q. J. Roy. Meteor. Soc., 133, 749–764, 2007. a
Xie, Y., Mauzerall, D. L., Zhou, M., and Hunt, K. M. R.: Half of India's recent winter PM2.5 air quality improvements are driven by meteorology, Nat. Sustain., 7, 983–993, https://doi.org/10.1038/s41893-024-01366-y, 2024. a, b
Xu, C., Yan, M., Ning, L., and Liu, J.: Summer westerly jet in northern hemisphere during the mid-holocene: a multi-model study, Atmosphere, 11, 1193, https://doi.org/10.3390/atmos11111193, 2020. a
Yadav, B. P., Kumar, N., and Lotus, S.: Synoptic & climatological aspects of extreme rainfall over western Himalayas towards end of 2014 southwest monsoon season, Mausam, 68, 597–606, 2017a. a, b
Yadav, R. K., Rupa Kumar, K., and Rajeevan, M.: Increasing influence of ENSO and decreasing influence of AO/NAO in the recent decades over northwest India winter precipitation, J. Geophys. Res.-Atmos., 114, D12112, https://doi.org/10.1029/2008JD011318, 2009. a, b, c, d
Yadav, R. K., Kumar, K. R., and Rajeevan, M.: Characteristic features of winter precipitation and its variability over northwest India, J. Earth Syst. Sci., 121, 611–623, 2012. a, b, c
Yadav, R. R., Gupta, A. K., Kotlia, B. S., Singh, V., Misra, K. G., Yadava, A. K., and Singh, A. K.: Recent wetting and glacier expansion in the northwest Himalaya and Karakoram, Sci. Rep., 7, 1–8, 2017b. a
Yadav, R. R., Gupta, A. K., Kotlia, B. S., Singh, V., Misra, K. G., Yadava, A. K., and Singh, A. K.: Recent wetting and glacier expansion in the northwest Himalaya and Karakoram, Sci. Rep., 7, 6139, https://doi.org/10.1038/s41598-017-06388-5, 2017c. a
Yadava, P. K., Soni, M., Verma, S., Kumar, H., Sharma, A., and Payra, S.: The major lightning regions and associated casualties over India, Nat. Hazards, 101, 217–229, 2020. a
Yang, S., Lau, K. M., and Kim, K. M.: Variations of the East Asian jet stream and Asian–Pacific–American winter climate anomalies, J. Climate, 15, 306–325, 2002. a
Yatagai, A., Kamiguchi, K., Arakawa, O., Hamada, A., Yasutomi, N., and Kitoh, A.: APHRODITE: Constructing a Long-Term Daily Gridded Precipitation Dataset for Asia Based on a Dense Network of Rain Gauges, B. Am. Meteorol. Soc., 93, 1401–1415, https://doi.org/10.1175/BAMS-D-11-00122.1, 2012. a, b
Yatagai, A., Masuda, N., Tanaka, K., and Higuchi, A.: APHRODITE-2: Improved grid precipitation algorithm – Initial results, Abstracts of the Society of Hydrology and Water Resources, 30, 30, 2017. a
Ye, H., Bao, Z., and Feng, X.: Connections of Siberian snow onset dates to the following summer's monsoon conditions over Southeast Asia, Int. J. Climatol., 25, 1567–1584, 2005. a
You, Q., Chen, D., Wu, F., Pepin, N., Cai, Z., Ahrens, B., Jiang, Z., Wu, Z., Kang, S., and AghaKouchak, A.: Elevation dependent warming over the Tibetan Plateau: Patterns, mechanisms and perspectives, Earth-Sci. Rev., 210, 103349, https://doi.org/10.1016/j.earscirev.2020.103349, 2020. a
Yuval, J. and Kaspi, Y.: Eddy activity response to global warming–like temperature changes, J. Climate, 33, 1381–1404, 2020. a
Zaz, S. N., Romshoo, S. A., Krishnamoorthy, R. T., and Viswanadhapalli, Y.: Analyses of temperature and precipitation in the Indian Jammu and Kashmir region for the 1980–2016 period: implications for remote influence and extreme events, Atmos. Chem. Phys., 19, 15–37, https://doi.org/10.5194/acp-19-15-2019, 2019. a, b, c
Zhu, L. and Fan, G.: Assessment and projection of elevation-dependent warming over the Tibetan Plateau by CMIP6 models, Theor. Appl. Climatol., 147, 1713–1723, 2022. a
Ziegler, A. D., Wasson, R. J., Sundriyal, Y., Srivastava, P., Sasges, G., Ramchunder, S. J., Ong, C. E., Nepal, S. K., McAdoo, B. G., Gillen, J., Bishwokarma, D., Bhardwaj, A., and Apollo, M.: A call for reducing tourism risk to environmental hazards in the Himalaya, Environmental Hazards, 22, 1–28, 2023. a
The choice of 350 hPa arises from Fig. 8, which shows composite WDs have their maximum vorticity at this pressure level.
Modern-Era Retrospective analysis for Research and Applications version 2
Asian Precipitation – Highly-Resolved Observational Data Integration Towards Evaluation of Water Resources
The IMD also define a “western depression”, as a strong WD that has two or more closed isobars in surface pressure. That term has not entered common use and has become conflated with active WD, which is the term we recommend for systems associated with heavy precipitation.
The SAM and NAO are, strictly speaking, atmospheric phenomena. However, they also rectify onto SSTs forming very similar patterns to those shown here (Kushnir et al., 2006; Sen Gupta and England, 2007).
Arctic Oscillation: a mode of climate variability related to the NAO but with no fixed periodicity, whose positive phase is associated with low surface pressure over the Arctic and high surface pressure in the midlatitudes.
ka, or kiloannum, measures the number of years, in thousands, before 1950 CE.
Since a warmer tropical atmosphere can hold more moisture (about 7 % K−1 at saturation), in the absence of a large-scale circulation change, we would expect a robust increase in winter precipitation.
- Abstract
- Introduction
- Structure, dynamics, thermodynamics, and life cycle
- Precipitation and natural hazards
- Large-scale forcing and teleconnections
- Model evaluation and verification
- Forecasting
- Climate change
- Conclusions
- Data availability
- Author contributions
- Competing interests
- Disclaimer
- Acknowledgements
- Financial support
- Review statement
- References
- Abstract
- Introduction
- Structure, dynamics, thermodynamics, and life cycle
- Precipitation and natural hazards
- Large-scale forcing and teleconnections
- Model evaluation and verification
- Forecasting
- Climate change
- Conclusions
- Data availability
- Author contributions
- Competing interests
- Disclaimer
- Acknowledgements
- Financial support
- Review statement
- References